Graph Neural Network via Edge Convolution for Hyperspectral Image Classification
IEEE GEOSCIENCE AND REMOTE SENSING LETTERS(2022)
摘要
Graph neural network (GNN) has recently gained increasing attention in the hyperspectral image (HSI) classification. Compared with convolutional neural network (CNN), GNN can effectively relieve the scarcity of labeled data. In our method, we first perform feature learning on large-scale irregular regions through GNN and then extract local spatial-spectral features at the pixel level. Besides, we incorporate edge convolution (EdgeConv) into GNN to adaptively capture the interrelationship of the representative descriptors and fully exploit the discriminative features on graph. Experiments on several HSI datasets show that our method can achieve better classification performance compared with the state-of-the-art HSI classification methods.
更多查看译文
关键词
Convolutional neural networks,Feature extraction,Image edge detection,Message passing,Convolution,Hyperspectral imaging,Time complexity,Convolutional neural network (CNN),edge convolution (EdgeConv),graph neural network (GNN),hyperspectral image (HSI)
AI 理解论文
溯源树
样例
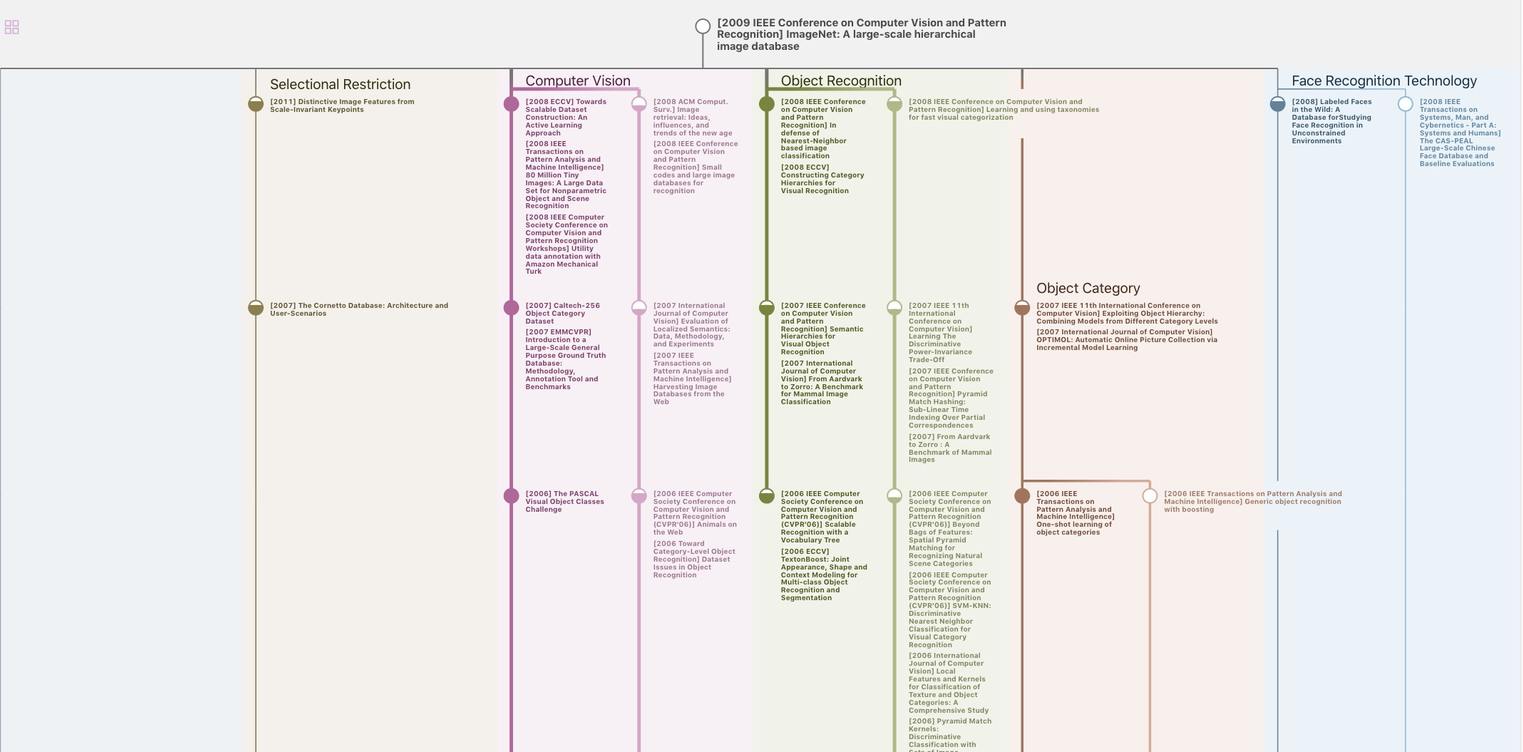
生成溯源树,研究论文发展脉络
Chat Paper
正在生成论文摘要