RESEPT: tissue architecture inference and visualization from spatially resolved transcriptomics
semanticscholar(2021)
摘要
We present RESEPT, a deep-learning framework for characterizing and visualizing tissue architecture from spatially resolved transcriptomics by reconstructing and segmenting a transcriptome mapped RGB image. RESEPT can identify the tissue architecture, and represent corresponding marker genes and biological functions accurately. RESEPT also provides critical insights into the underlying mechanisms driving the complex tissue heterogeneities in Alzheimer's disease and glioblastoma. Main Tissue architecture is the biological foundation of spatial heterogeneity within complex organs like the human brain1, and is thereby essential in understanding the underlying pathogenesis of human diseases, including cancer2 and Alzheimer's disease (AD)3. Recent advances in spatially resolved technologies such as 10x Genomics Visium provide spatial context together with highthroughput gene expression for exploring tissue domains, cell types, cell-cell communications, and their biological consequences4. Some graph-based clustering methods (e.g., Seurat5 and Giotto6), statistical methods (e.g., BayesSpace7), or deep learning-based methods (e.g., stLearn8 and SpaGCN9) can identify spatial architecture and interpret spatial heterogeneity. Intrinsic tissue architecture, however, often cannot be fully revealed using existing methods due to a lack of strong spatial representation for the biological context in tissues (Supplementary Note 1 summarizes the challenges of existing tools). Therefore, it is still challenging to accurately characterize tissue architectures and the underlying biological functions from spatial transcriptomics. . CC-BY-NC-ND 4.0 International license available under a was not certified by peer review) is the author/funder, who has granted bioRxiv a license to display the preprint in perpetuity. It is made The copyright holder for this preprint (which this version posted July 9, 2021. ; https://doi.org/10.1101/2021.07.08.451210 doi: bioRxiv preprint
更多查看译文
AI 理解论文
溯源树
样例
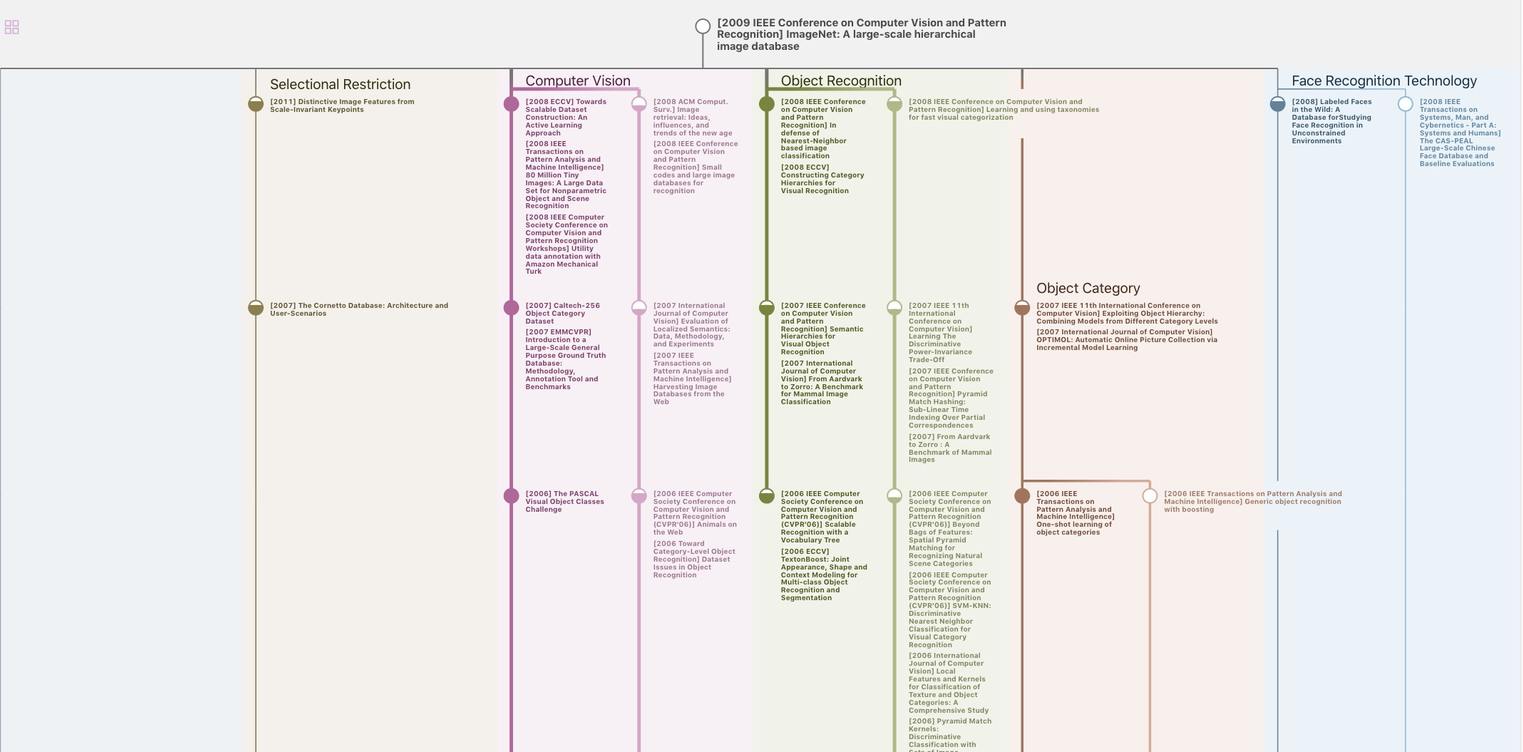
生成溯源树,研究论文发展脉络
Chat Paper
正在生成论文摘要