Large-scale node classification with bootstrapping
semanticscholar(2021)
摘要
Effectively and efficiently deploying graph neural networks (GNNs) at scale remains one of the most challenging aspects of graph representation learning. Many powerful solutions have only ever been validated on comparatively small datasets, often with counter-intuitive outcomes—a barrier which has recently been broken by the Open Graph Benchmark Large-Scale Challenge (OGB-LSC). Here we describe our OGB-LSC entry for the MAG240M-LSC benchmark: a deep transductive node classifier powered by bootstrapping. Our model achieved an award-level (top-3) performance. In doing so, we demonstrate strong evidence of scalable self-supervised graph representation learning, and utility of deep GNNs—both very important open issues. Our code is publicly available at: https://github.com/deepmind/deepmind-research/tree/master/ogb_lsc/mag.
更多查看译文
AI 理解论文
溯源树
样例
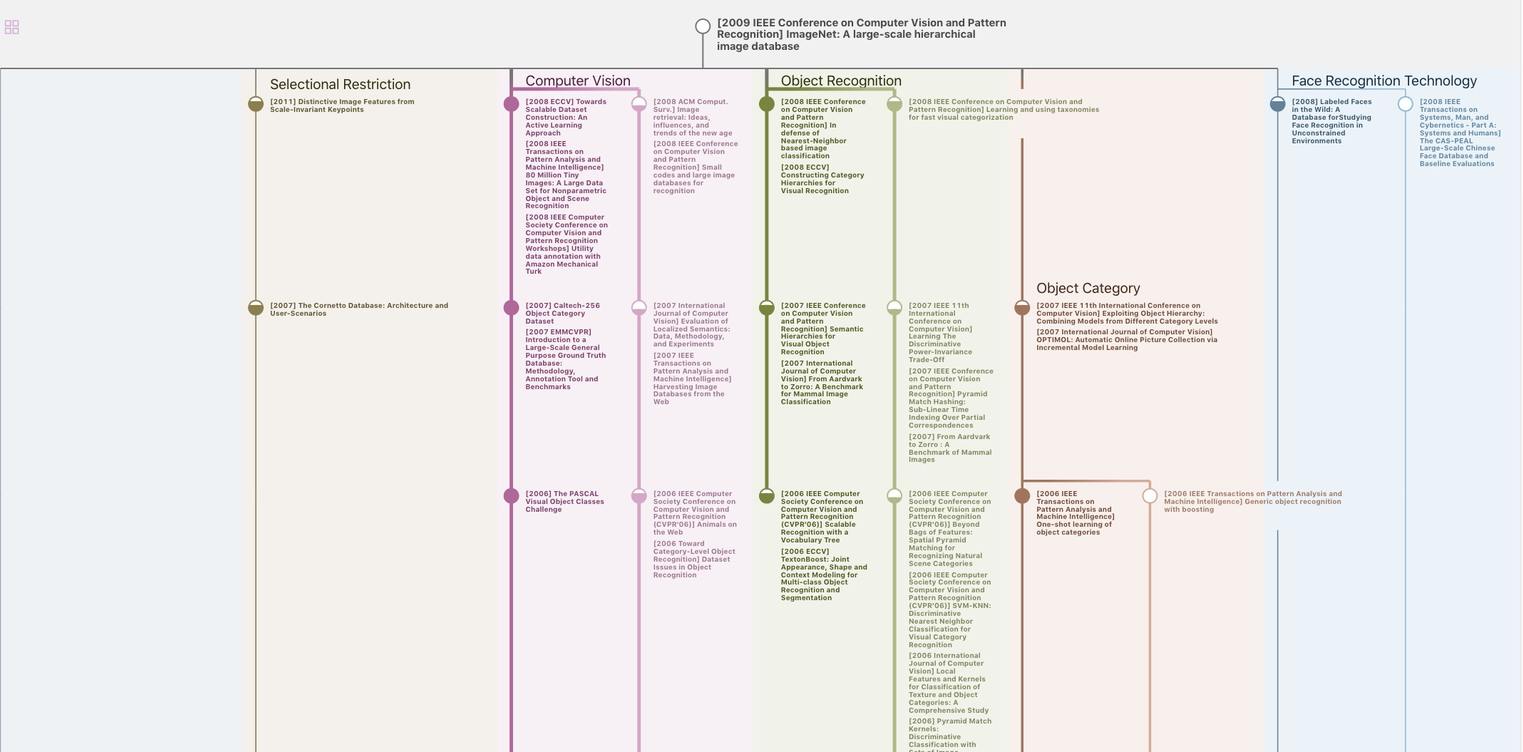
生成溯源树,研究论文发展脉络
Chat Paper
正在生成论文摘要