Branch-and-bound Algorithm for Optimal Sparse Canonical Correlation Analysis
EXPERT SYSTEMS WITH APPLICATIONS(2021)
摘要
Canonical correlation analysis (CCA) is a family of multivariate statistical methods for extracting mutual information contained in multiple datasets. To improve the interpretability of CCA, here we focus on the mixed-integer optimization (MIO) approach to sparse estimation. This approach was first proposed for sparse linear regression in the 1970s, but it has recently received renewed attention due to advances in optimization algorithms and computer hardware. We first derive an MIO problem for optimal sparse CCA estimation. To solve this MIO problem exactly, we propose a branch-and-bound algorithm based on the generalized eigenvalue problem for computing effective lower and upper bounds. We prove that our algorithm finds a solution with guaranteed optimality in terms of the canonical correlation. Computational results demonstrate that our method is much faster than direct application of optimization software to the MIO problem. Moreover, our method can provide better-quality solutions than can forward stepwise selection and L1regularized estimation in terms of generalization performance. These results enhance the potential of optimal sparse estimation in multivariate statistical analyses. ∗Corresponding author Preprint submitted to Elsevier Journal April 25, 2021
更多查看译文
关键词
Canonical correlation analysis,Branch-and-bound algorithm,Sparse estimation,Mixed-integer optimization,Statistics
AI 理解论文
溯源树
样例
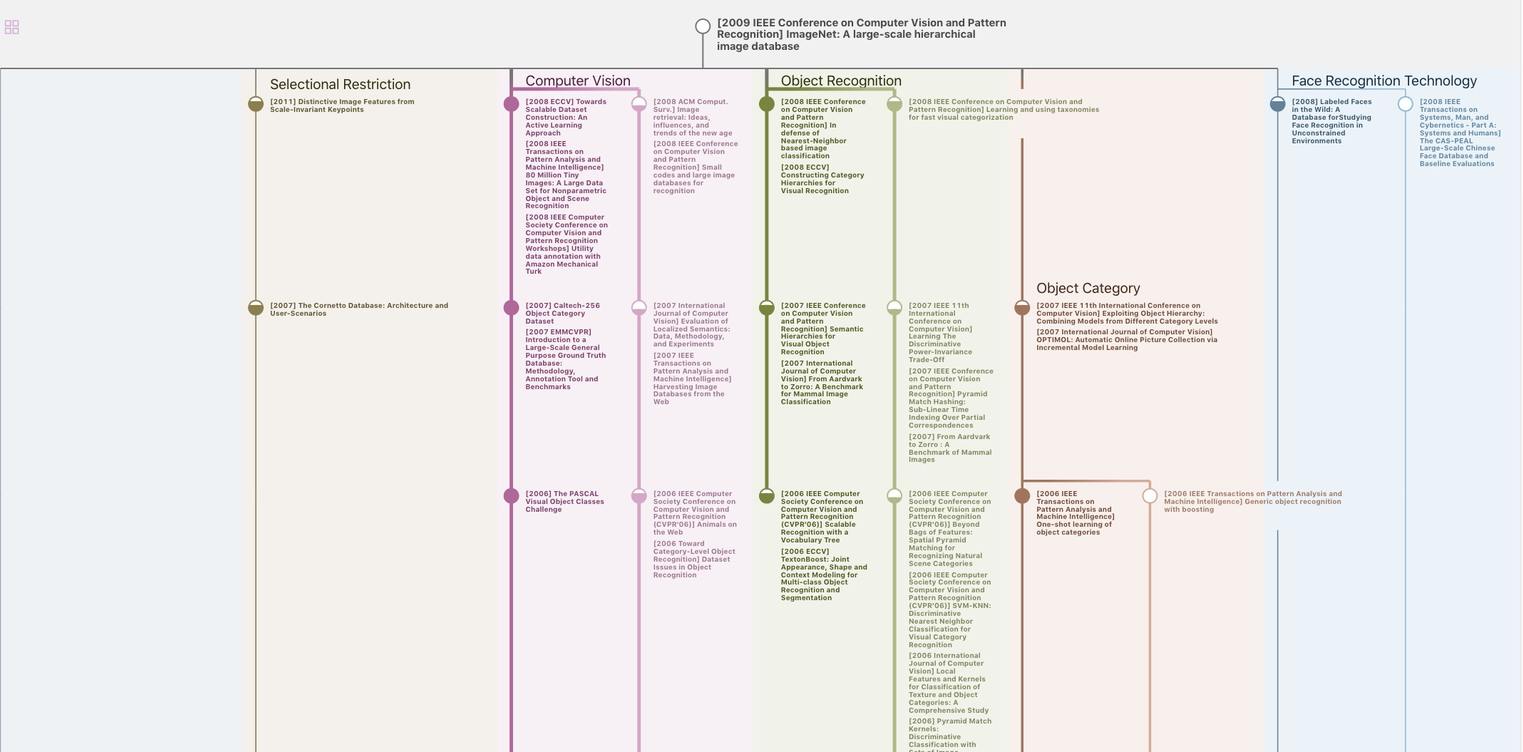
生成溯源树,研究论文发展脉络
Chat Paper
正在生成论文摘要