Contrast-Based Unsupervised Hashing Learning With Multi-Hashcode
IEEE Signal Processing Letters(2022)
摘要
Due to high storage and calculation efficiency, hash-based methods have been widely used in image retrieval systems. Unsupervised deep hashing methods can learn the binary representations of images effectively without any annotations. The strategy of constraining hash code in the previous unsupervised methods may not fully utilize the structural information in semantic similarity. To address this problem, we propose a new strategy based on contrastive learning to capture high-level semantic similarity among features and preserve it in generated hash codes. In addition, we employ a novel framework to handle hash codes with different lengths simultaneously which is more time-saving in generating hash codes than existing methods. Extensive experiments on MIRFlickr, NUS-WIDE, and COCO benchmark datasets show that our method makes great improvement on the performance of unsupervised image retrieval.
更多查看译文
关键词
Contrastive learning,image retrieval,unsupervised hashing
AI 理解论文
溯源树
样例
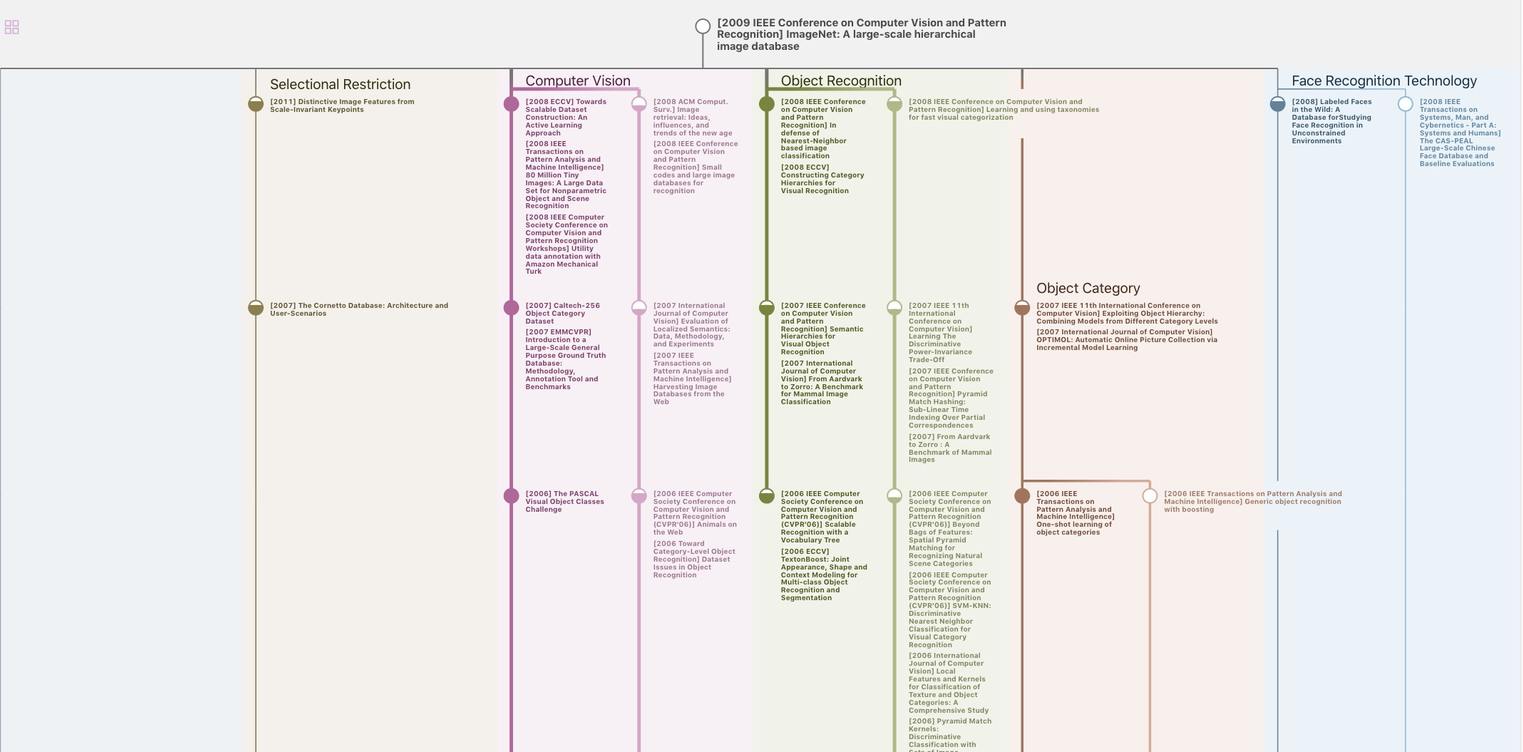
生成溯源树,研究论文发展脉络
Chat Paper
正在生成论文摘要