Automated Learning of Radiation Therapy Dose Distribution for Head and Neck Cancer
semanticscholar(2021)
摘要
In the past decades, 3D dose optimization, with the goal of providing a prescription dose to the tumor while maximally sparing surrounding healthy organs at risk (OARs), has been playing a pivotal role in external beam radiation therapy. To reach a practical clinical treatment plan, the optimization solution needs to be adjusted by human planners. However, this procedure necessitates intensive computations combined with iterative inputs from an expert human planner. This is a time-intensive process with dose distribution solutions that are highly dependent on the human expertise of an institution. To remedy the computational time issue, and also reduce the variability, while capturing the existing knowledge, we use machine learning capabilities. In particular, we propose a suitable feature matrix for head and neck cancer and train Deep Neural Network models to mimic the Planned Dose Distribution procedure. When trained, the model can provide dose values in less than a second. Toward this, a specific Convolutional Neural Network called U-Net model is utilized. The numerical results show that this model reduces the solution time of each patient on the utilized data from 3-6 hours to 1 second while providing highly accurate solutions such that the average error is approximately 3.2% and 7.0% for cancerous organs and OARs, respectively.
更多查看译文
AI 理解论文
溯源树
样例
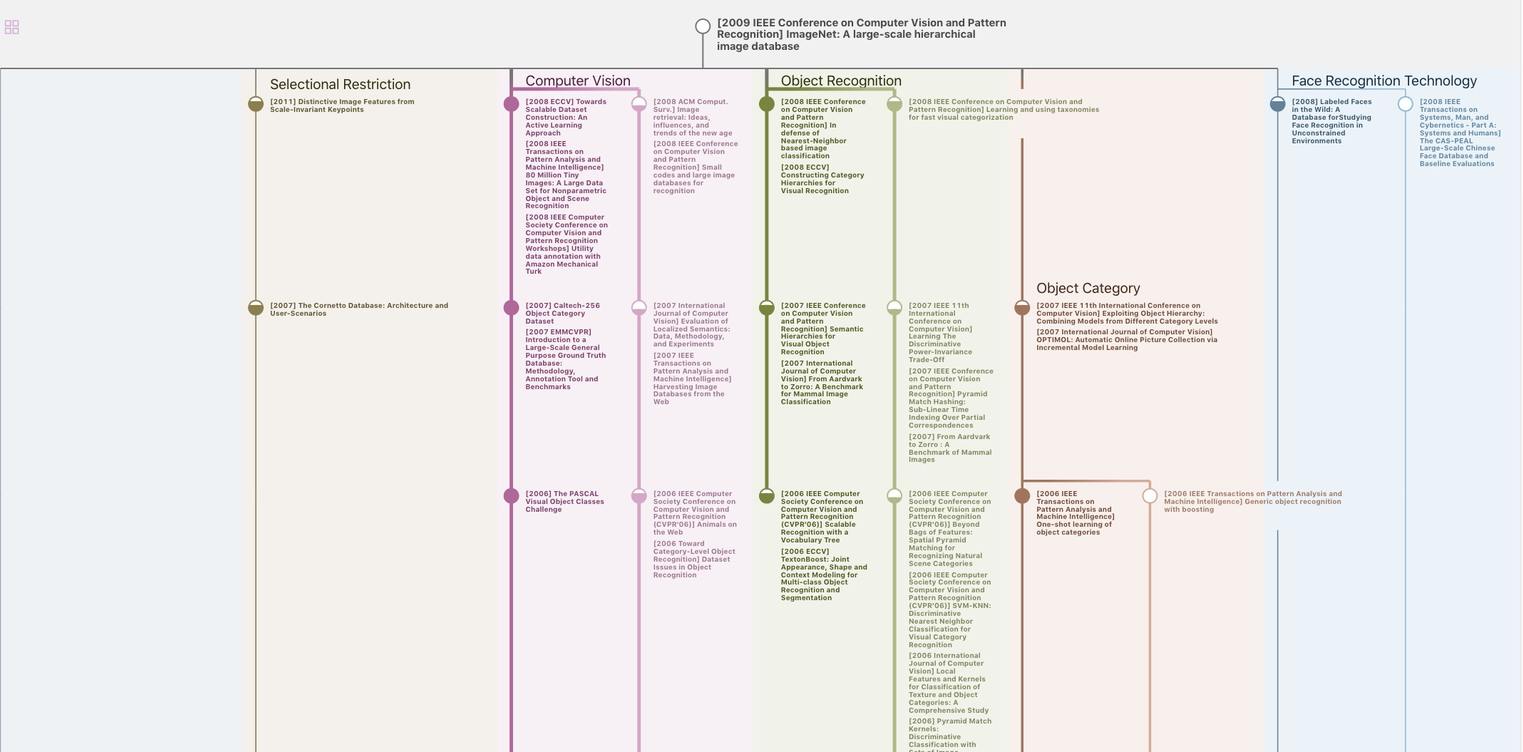
生成溯源树,研究论文发展脉络
Chat Paper
正在生成论文摘要