Decision support for GPU acceleration by predicting energy savings and programming effort
SUSTAINABLE COMPUTING-INFORMATICS & SYSTEMS(2022)
摘要
As the number of heterogeneous embedded systems used in IoT applications increases, there is a lack of software tools to assist developers to meet the challenge of reducing energy consumption. Indeed, there are only few performance prediction tools for heterogeneous systems in the literature and they typically focus on the prediction of speedup by acceleration. In this work, we propose a methodology for analyzing CPU applications in order to estimate the potential Energy gains by offloading a piece of code on an embedded GPU. The proposed methodology provides several features beyond the state of the art of existing predictors, including the combination of static analysis and dynamic instrumentation approaches and the prediction of the programming effort of developing the CUDA kernel of a CPU code, using advanced metrics. The methodology is supported by a tool-flow and it is demonstrated and evaluated on modern heterogeneous embedded systems (Nvidia), where shows classification accuracy above 75%. The results show that the proposed methodology can assist application developers in the early design choice of investing effort to acceleration considering the expected Energy Savings and the Effort required to develop acceleration-specific code.
更多查看译文
关键词
Energy consumption,Embedded systems,GPU acceleration,Software design
AI 理解论文
溯源树
样例
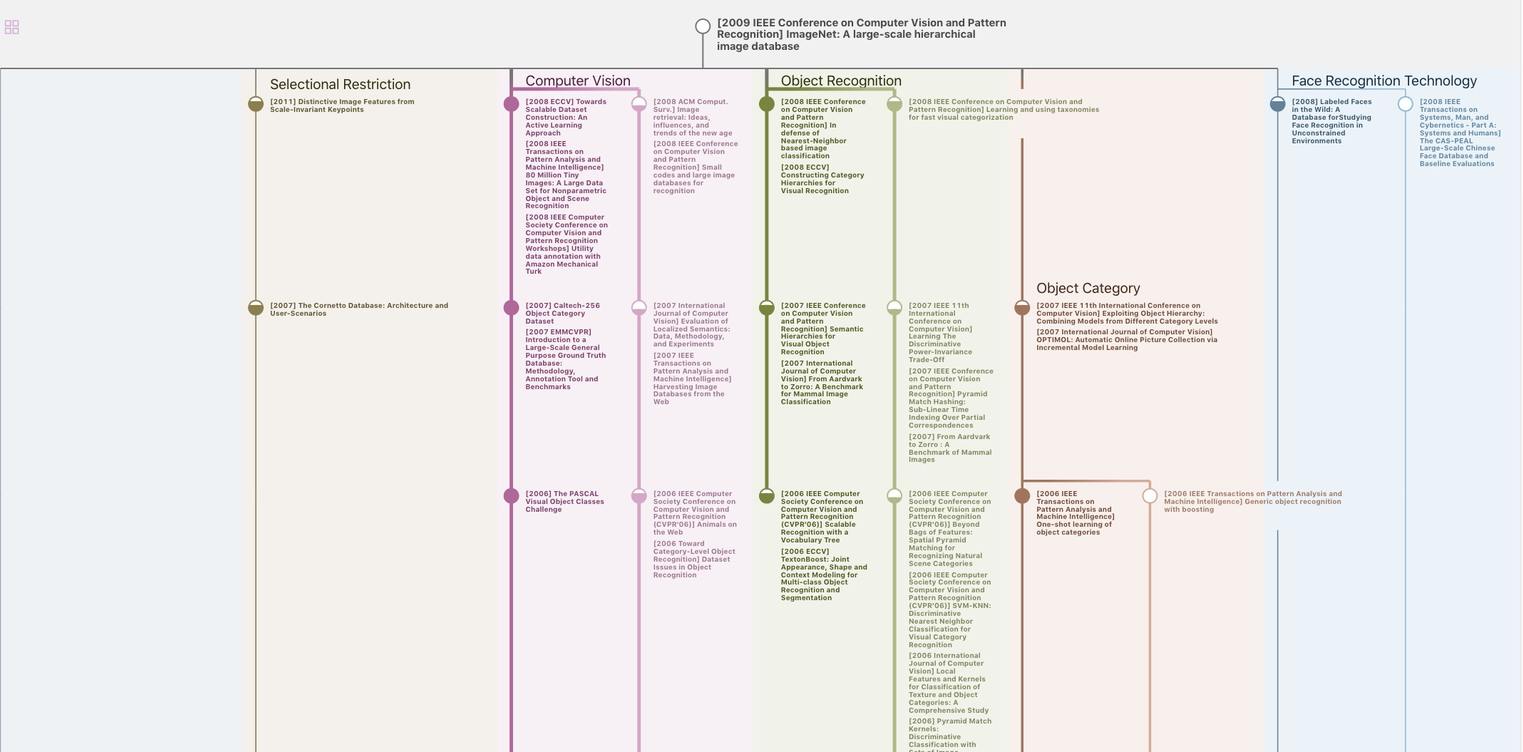
生成溯源树,研究论文发展脉络
Chat Paper
正在生成论文摘要