Network Experiment Design for Estimating Direct Treatment Effects
semanticscholar(2020)
摘要
Network experiment design refers to the design of controlled experiments for interacting units with the goal of estimating a causal effect of interest. Estimating the effect of treatment alone on units’ outcome, known as direct treatment effect, in network experiments is challenging due to information spillover between peers through shared edges. Prominent methods for network experiment design mostly focus on estimating total treatment effects, the combination of peer effects and direct treatment effects. Less focus has been given to approaches that provide an unbiased estimation of direct treatment effect. We present a framework that takes advantage of independent sets and assigns treatment and control only to a set of non-adjacent nodes in a graph, in order to disentangle peer effects from direct treatment effect estimation. Randomizing over independent set nodes removes peer effects between nodes in the experiment while canceling out the peer effects from nodes outside the experiment. Through a series of simulated experiments on synthetic and real-world network datasets, we show that our framework significantly increases the accuracy of direct treatment effect estimation in network experiments.
更多查看译文
AI 理解论文
溯源树
样例
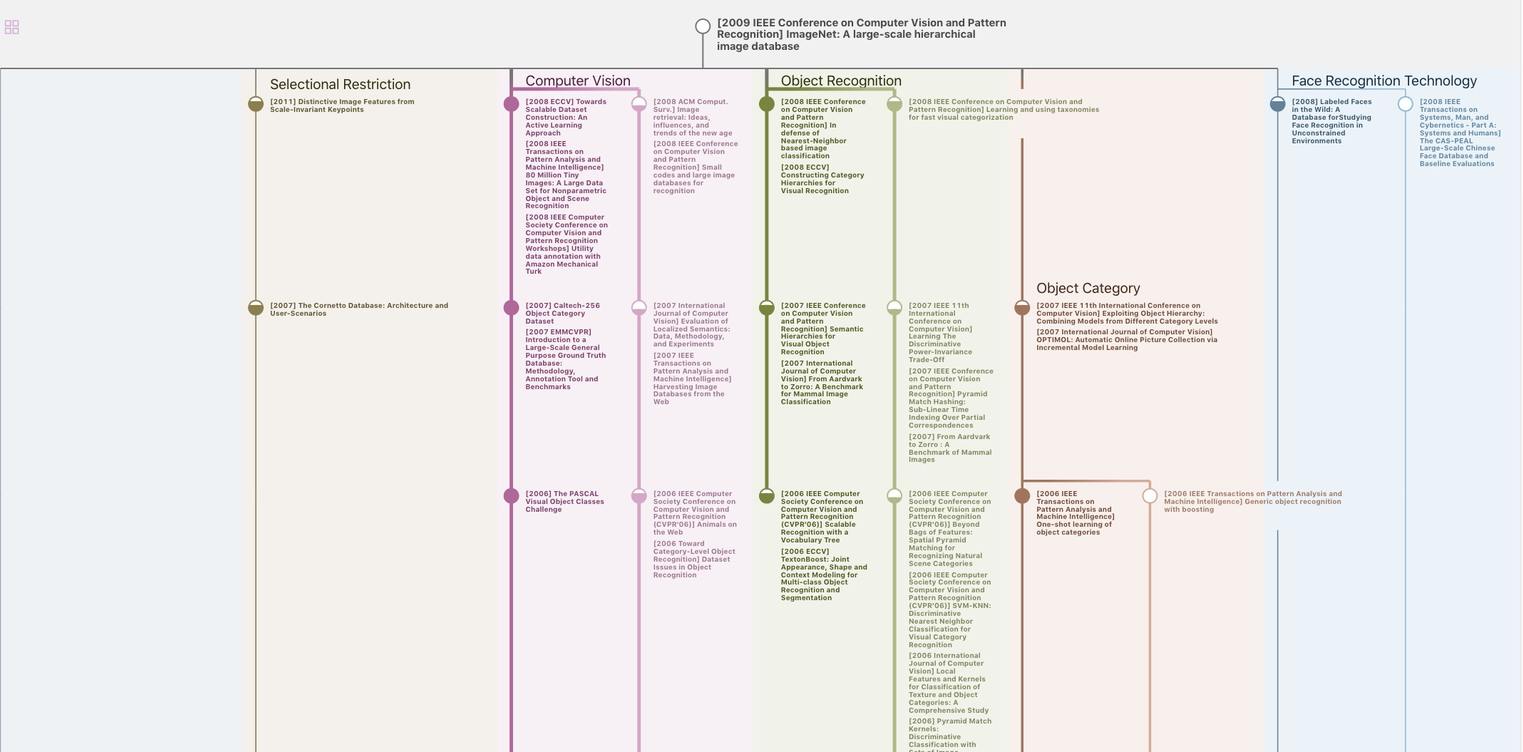
生成溯源树,研究论文发展脉络
Chat Paper
正在生成论文摘要