On Learning Prediction-Focused Mixtures.
arXiv (Cornell University)(2021)
摘要
Probabilistic models help us encode latent structures that both model the data and are ideally also useful for specific downstream tasks. Among these, mixture models and their time-series counterparts, hidden Markov models, identify discrete components in the data. In this work, we focus on a constrained capacity setting, where we want to learn a model with relatively few components (e.g. for interpretability purposes). To maintain prediction performance, we introduce prediction-focused modeling for mixtures, which automatically selects the dimensions relevant to the prediction task. Our approach identifies relevant signal from the input, outperforms models that are not prediction-focused, and is easy to optimize; we also characterize when prediction-focused modeling can be expected to work.
更多查看译文
关键词
mixtures,learning,prediction-focused
AI 理解论文
溯源树
样例
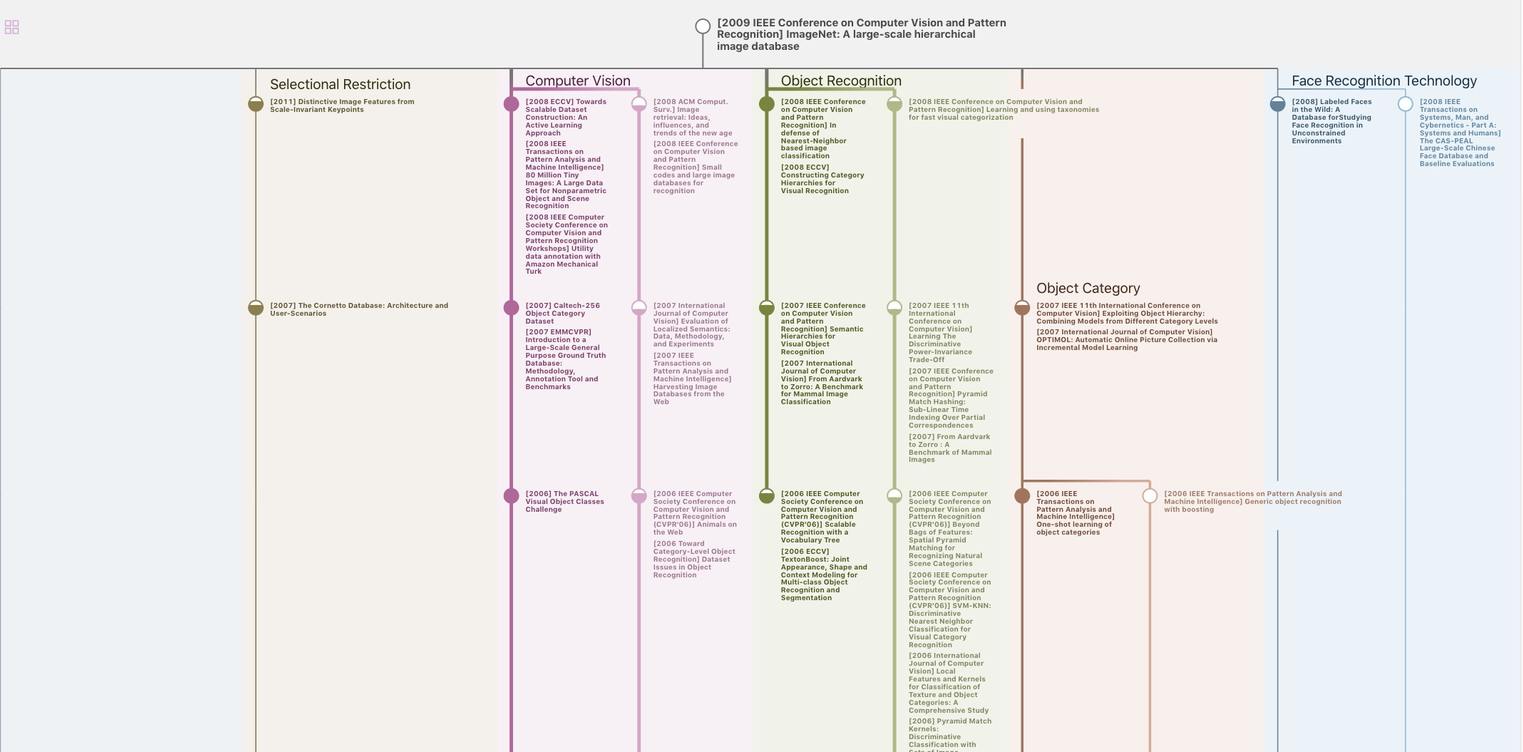
生成溯源树,研究论文发展脉络
Chat Paper
正在生成论文摘要