Few-shot Link Prediction in Dynamic Networks
WSDM(2021)
摘要
Dynamic link prediction, which aims at forecasting future edges of a node in a dynamic network, is an important problem in network science and has a wide range of real-world applications. A key property of dynamic networks is that new nodes and links keep coming over time and these new nodes usually have only a few links at their arrivals. However, how to predict future links for these few-shot nodes in a dynamic network has not been well studied. Existing dynamic network representation learning methods were not specialized for few-shot scenarios and thus would lead to suboptimal performances. In this paper, we propose a novel model based on a meta-learning framework, dubbed as MetaDyGNN, for few-shot link prediction in dynamic networks. Specifically, we propose a meta-learner with hierarchical time interval-wise and node-wise adaptions to extract general knowledge behind this problem. We also design a simple and effective dynamic graph neural network (GNN) module to characterize the local structure of each node in meta-learning tasks. As a result, the learned general knowledge serves as model initializations, and can quickly adapt to new nodes with a fine-tuning process on only a few links. Experimental results show that our proposed MetaDyGNN significantly outperforms state-of-the-art methods on three publicly available datasets.
更多查看译文
关键词
link prediction, dynamic network, few-shot prediction, meta-learning, graph neural networks
AI 理解论文
溯源树
样例
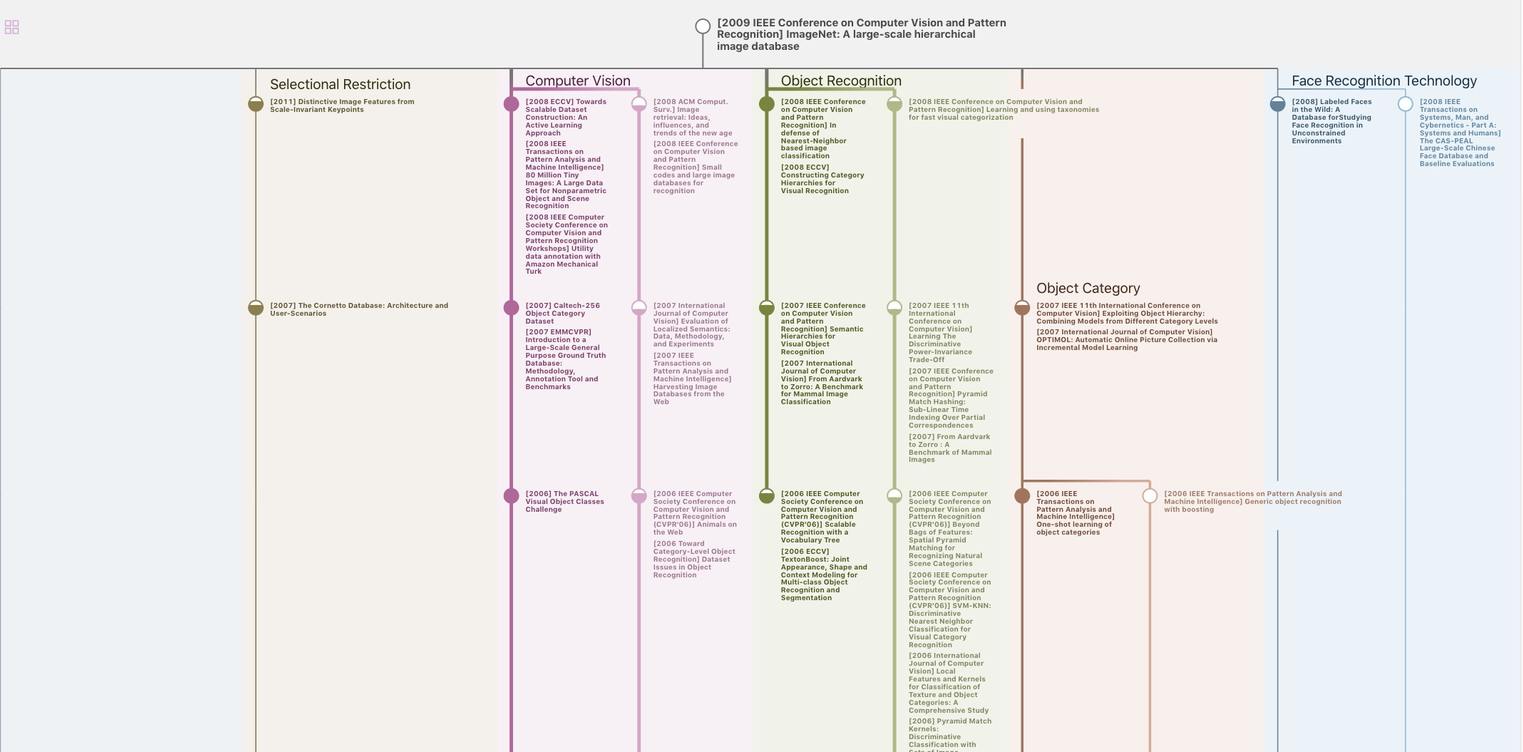
生成溯源树,研究论文发展脉络
Chat Paper
正在生成论文摘要