Explainable Machine Learning-driven Strategy for Automated Trading Pattern Extraction
semanticscholar(2021)
摘要
Financial markets are a source of non-stationary multidimensional time series which has been drawing attention for decades. Each financial instrument has its specific changing over time properties, making their analysis a complex task. Improvement of understanding and development of methods for financial time series analysis is essential for successful operation on financial markets. In this study we propose a volume-based data pre-processing method for making financial time series more suitable for machine learning pipelines. We use a statistical approach for assessing the performance of the method. Namely, we propose a set of research questions, formally state the hypotheses, compute effect sizes with confidence intervals, and reject the null hypotheses. We additionally assess the trading performance of the proposed method on historical data and compare it to a previously published approach. Our analysis shows that the proposed volume-based method allows successful classification of the financial time series patterns, and also leads to better classification performance than a price action-based method, excelling specifically on more liquid financial instruments. Finally, we propose an approach for obtaining feature interactions directly from tree-based models and compare the approach to SHAP method.
更多查看译文
关键词
extraction,pattern,learning-driven
AI 理解论文
溯源树
样例
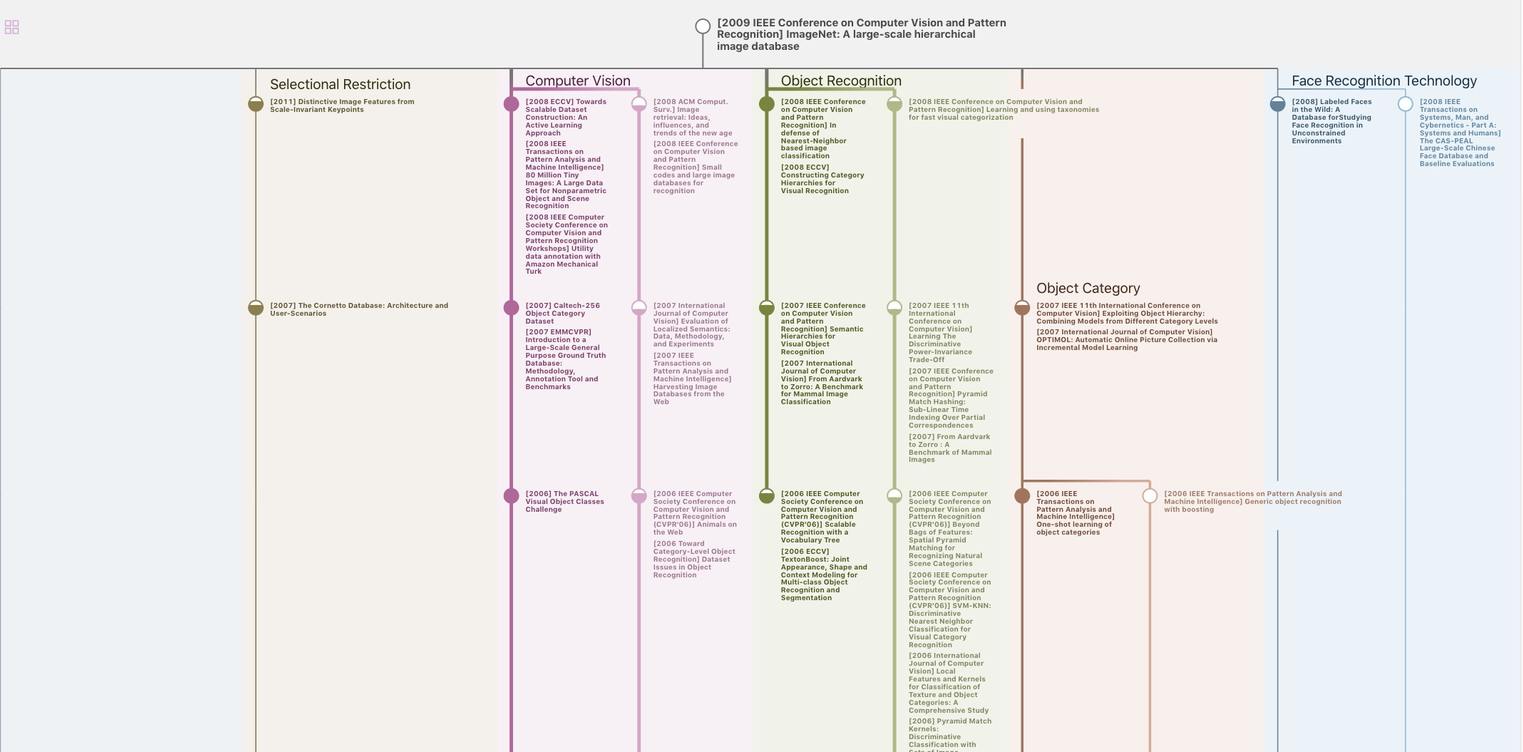
生成溯源树,研究论文发展脉络
Chat Paper
正在生成论文摘要