Wearable Motion Capture: Reconstructing and Predicting 3D Human Poses From Wearable Sensors
IEEE JOURNAL OF BIOMEDICAL AND HEALTH INFORMATICS(2023)
摘要
Reconstructing and predicting 3D human walking poses in unconstrained measurement environments have the potential to use for health monitoring systems for people with movement disabilities by assessing progression after treatments and providing information for assistive device controls. The latest pose estimation algorithms utilize motion capture systems, which capture data from IMU sensors and third-person view cameras. However, third-person views are not always possible for outpatients alone. Thus, we propose the wearable motion capture problem of reconstructing and predicting 3D human poses from the wearable IMU sensors and wearable cameras, which aids clinicians' diagnoses on patients out of clinics. To solve this problem, we introduce a novel Attention-Oriented Recurrent Neural Network (AttRNet) that contains a sensorwise attention-oriented recurrent encoder, a reconstruction module, and a dynamic temporal attention-oriented recurrent decoder, to reconstruct the 3D human pose over time and predict the 3D human poses at the following time steps. To evaluate our approach, we collected a new Wearable-MotionCapture dataset using wearable IMUs and wearable video cameras, along with the musculoskeletal joint angle ground truth. The proposed AttRNet shows high accuracy on the new lower-limbWearableMotionCapture dataset, and it also outperforms the state-of-the-art methods on two public full-body pose datasets: DIP-IMU and TotalCaputre.
更多查看译文
关键词
3D pose reconstruction,3d pose prediction,recurrent neural network,wearable sensing
AI 理解论文
溯源树
样例
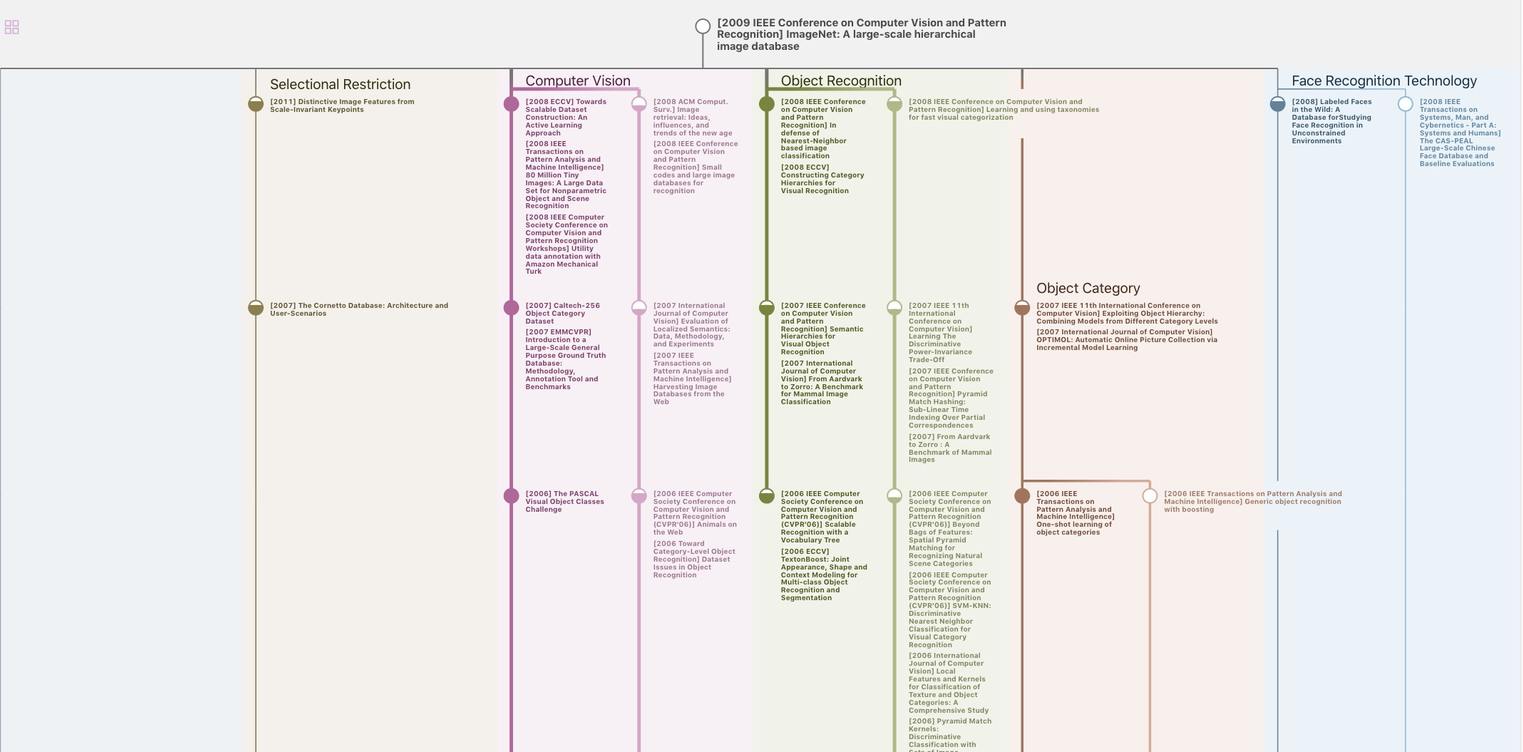
生成溯源树,研究论文发展脉络
Chat Paper
正在生成论文摘要