Bounded Space Differentially PrivateQuantiles
semanticscholar(2021)
摘要
Estimating the quantiles of a large dataset is a fundamental problem in both the streaming algorithms literature and the differential privacy literature. However, all existing private mechanisms for distribution-independent quantile computation require space at least linear in the input sizen. In this work, we devise a differentially private algorithm for the quantile estimation problem with strongly sublinear space complexity. Our main mechanism estimates any α-approximate quantile of a length-n stream over a data universe X with probability 1 − β using O ( log( |X |/β) log(αεn)
更多查看译文
AI 理解论文
溯源树
样例
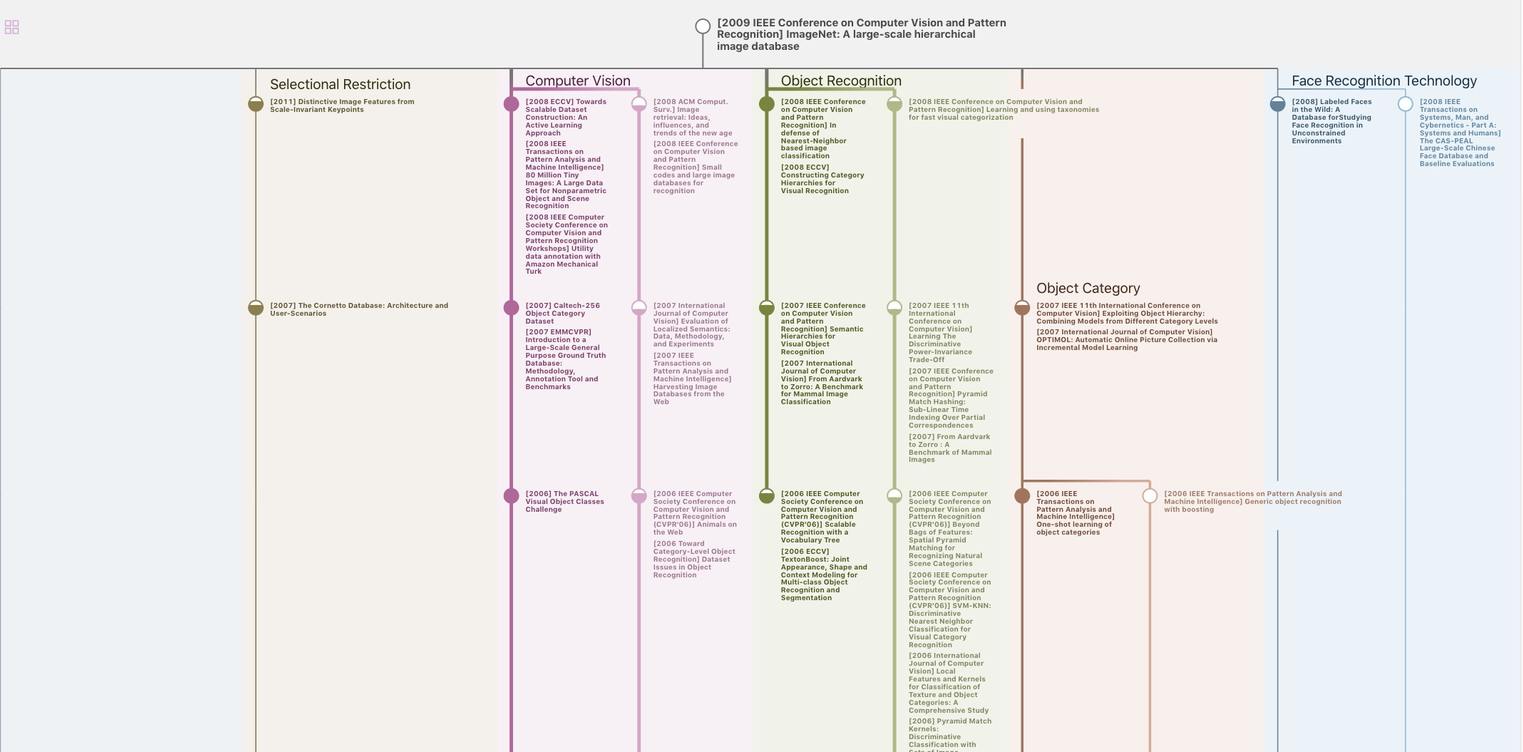
生成溯源树,研究论文发展脉络
Chat Paper
正在生成论文摘要