High-Order Representation Learning for Multivariate Time Series Forecasting
semanticscholar(2021)
摘要
Modeling dynamic relations between recording channels and the long-term dependencies are critical in multivariate time series. Recent approaches leverage graph neural networks to capture the direct first-order relationship between channels. While this is useful to capture cooccurrence patterns, they do not reveal indirect higher-order relationships governed by latent processes. To this end, we propose a dual message-passing recurrent neural system that disentangles the observed recording processes from the unobserved governing processes. The messages are passed in both the bottom-up and top-down manners: The bottom-up signals are aggregated to capture governing patterns, while the top-down messages augment the dynamics of low-level processes. Each process maintains its own memory of historical data, allowing processspecific long-term patterns to form. Throughout extensive experiments on real-world time-series forecasting datasets, we prove the robustness and efficiency of our approach across different scenarios.
更多查看译文
AI 理解论文
溯源树
样例
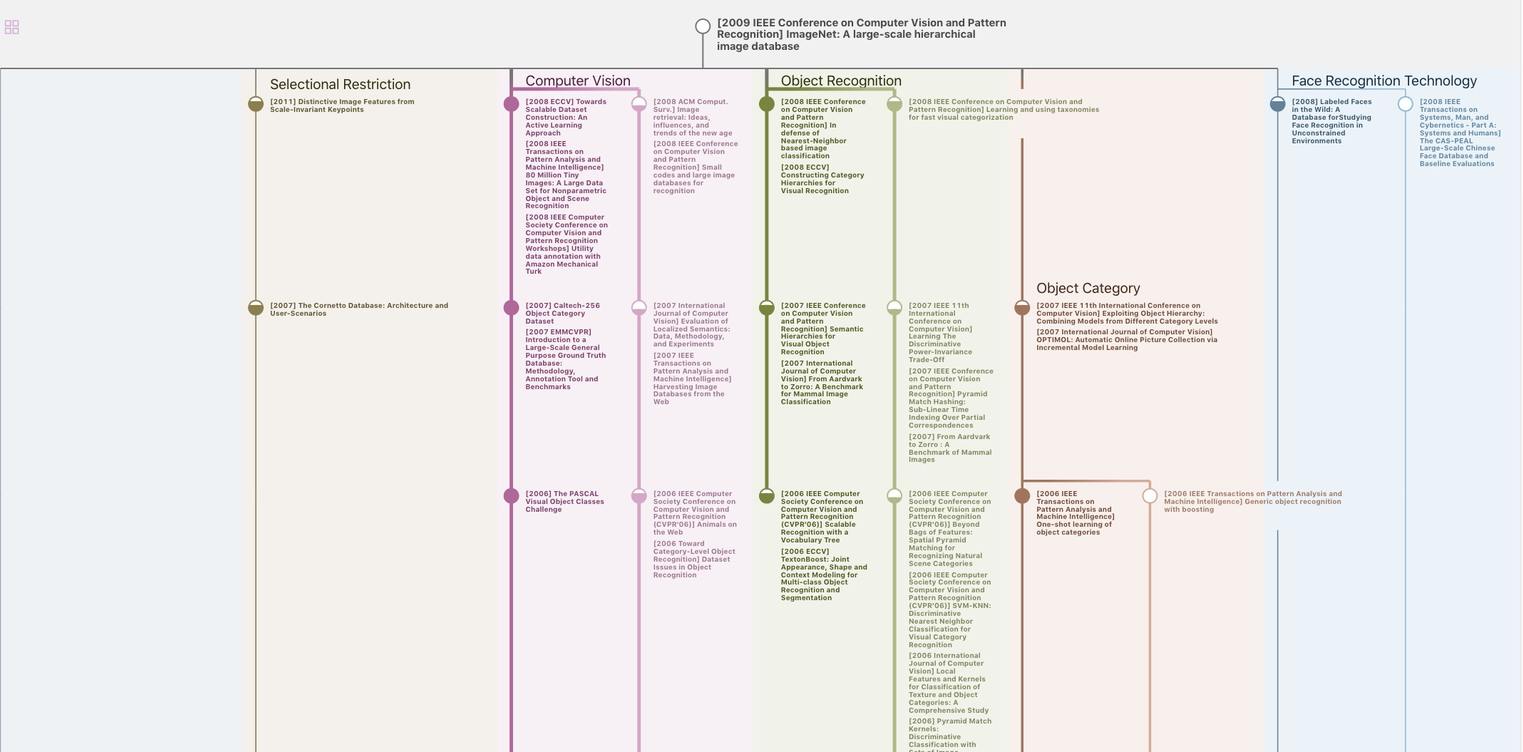
生成溯源树,研究论文发展脉络
Chat Paper
正在生成论文摘要