ICON: Supplementary Material
semanticscholar(2021)
摘要
1. Detailed proof of the regularizing effect of inverse consistency This section details our derivation for the smoothness properties emerging from approximate inverse consistency. Denote by Φ θ (x) the output map of a network for images (I , I) and by Φ θ (x) the output map between (I B , I) Recall that we add two independent spatial white noises n1(x), n2(x) ∈ R (x ∈ [0, 1] withN = 2 orN = 3 the dimension of the image) of variance 1 for each spatial location to the two output maps and define Φ θε (x) := Φ AB θ (x) + εn1(Φ AB θ (x)) and Φ θε (x) := Φ BA θ (x) + εn2(Φ BA θ (x)) with ε a positive parameter. We consider the following loss L = λ ( ‖Φ θε ◦ Φ θε − Id ‖2 + ‖Φ θε ◦ Φ θε − Id ‖2 ) + ‖I ◦ Φ θ − I‖2 + ‖I ◦ Φ θ − I‖2 . (1) Throughout this section, we give the details of the expansion in ε of the loss, thus we use the standard notations o and O w.r.t ε → 0. We focus on the first two terms (that we denote by λLinv) since the regularizing property comes from the inverse consistency. We expand one of the first two terms of (1) since by symmetry the other is similar. If the noise is bounded (or with high probability in the case of Gaussian noise), we have ‖Φ θε ◦ Φ θε − Id ‖2 = ‖Φ θ ◦ Φ θ + εn1(Φ θ ◦ Φ θ ) + dΦ θε (εn2(Φ θ ))− Id ‖2 + o(ε) , (2) where dΦ denotes the Jacobian of Φ. By developing the squares and taking expectation, we get
更多查看译文
AI 理解论文
溯源树
样例
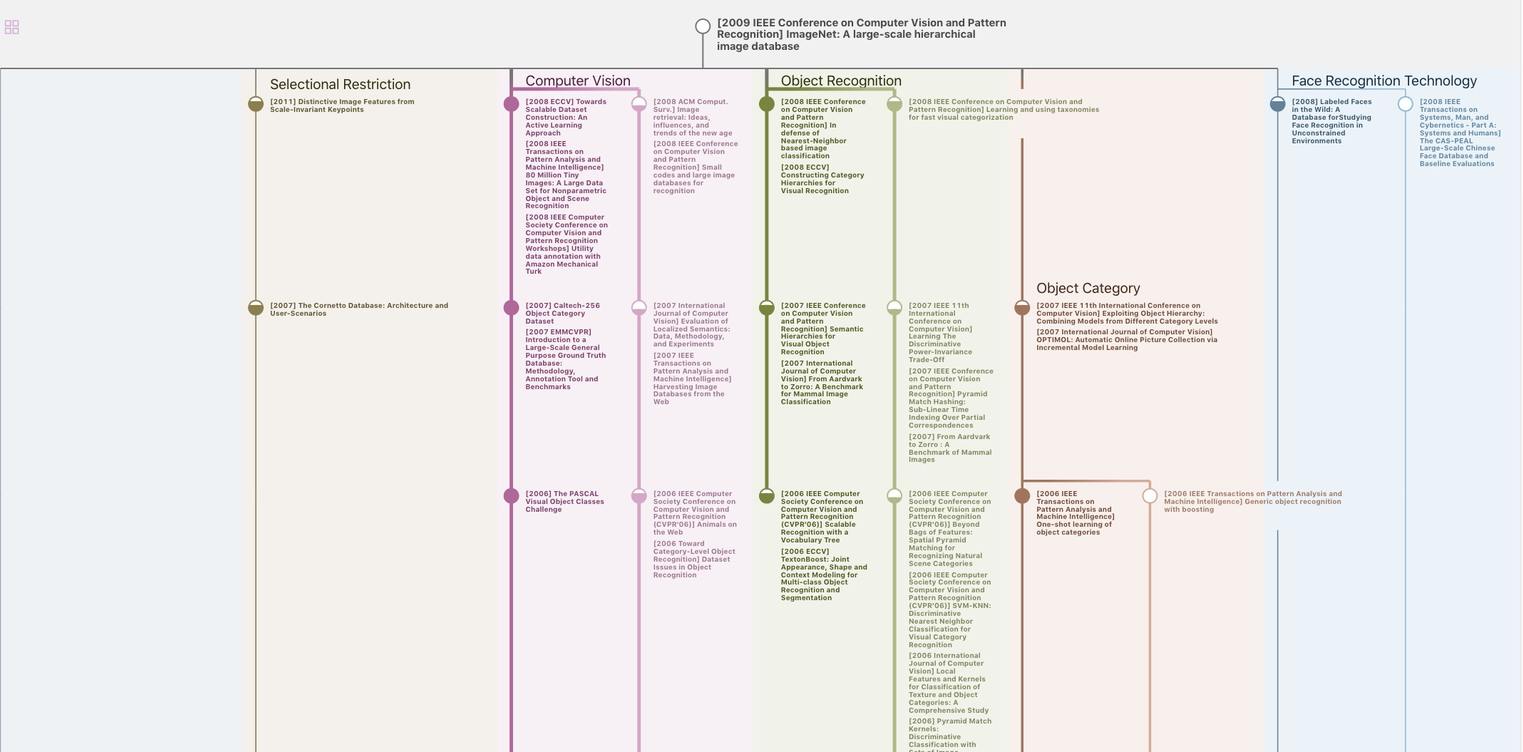
生成溯源树,研究论文发展脉络
Chat Paper
正在生成论文摘要