Fast estimation for robust supervised classification with mixture models
PATTERN RECOGNITION LETTERS(2021)
摘要
Label noise is known to negatively impact the performance of classification algorithms. In this paper, we develop a model robust to label noise that uses both labelled and unlabelled samples. In particular, we propose a novel algorithm to optimize the model parameters that scales efficiently w.r.t. the number of training samples. Our contribution relies on a consensus formulation of the original objective function that is highly parallelizable. The optimization is performed with the Alternating Direction Method of Multipliers framework. Experimental results on synthetic datasets show an improvement of several orders of magnitude in terms of processing time, with no loss in terms of accuracy. Our method appears also tailored to handle real data with significant label noise. (c) 2021 The Author(s). Published by Elsevier B.V. This is an open access article under the CC BY license ( http://creativecommons.org/licenses/by/4.0/ )
更多查看译文
关键词
Classification,Label noise,ADMM,Convex optimization,Clustering
AI 理解论文
溯源树
样例
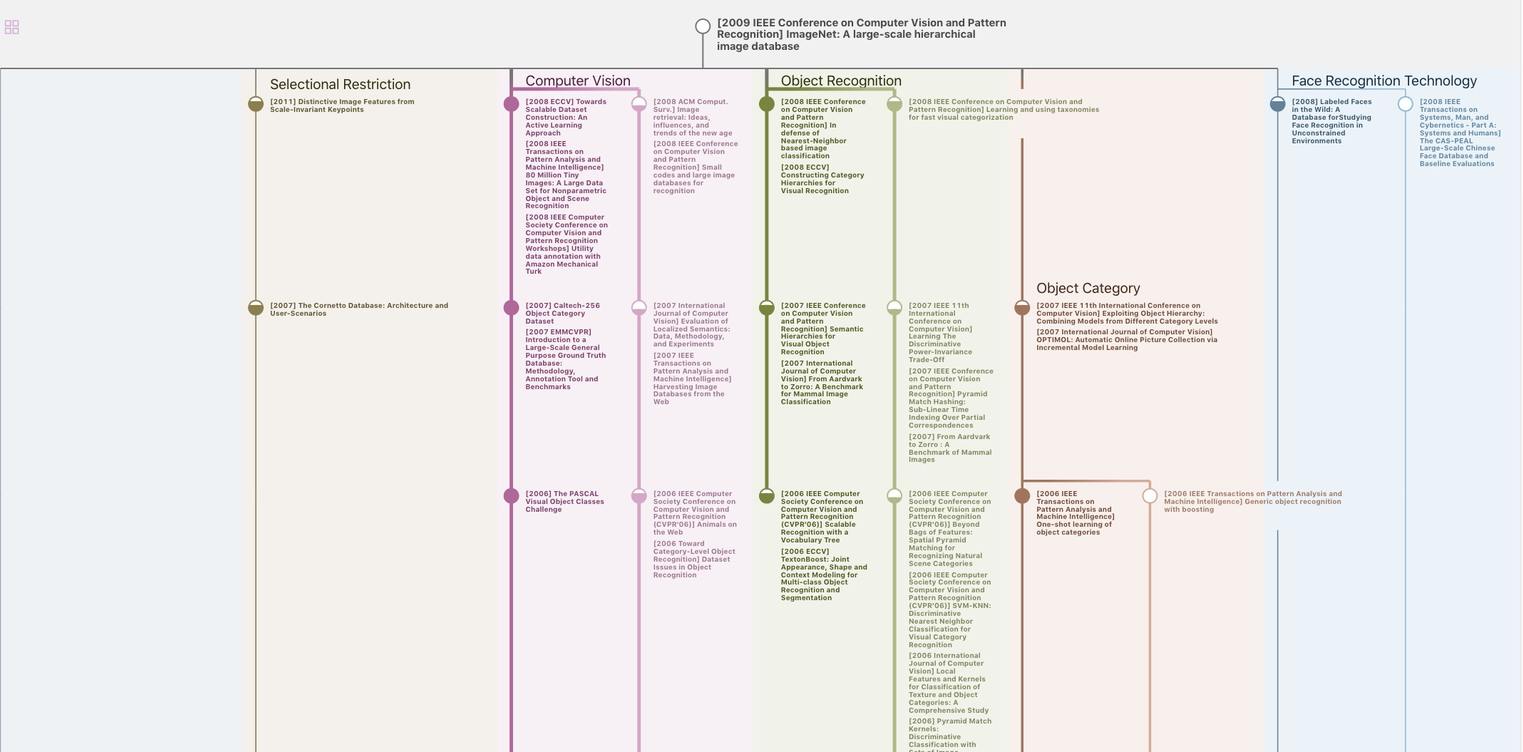
生成溯源树,研究论文发展脉络
Chat Paper
正在生成论文摘要