On the robustness of minimum-norm interpolators
arXiv (Cornell University)(2020)
摘要
This article develops a general theory for minimum-norm interpolated estimators in linear models in the presence of additive, potentially adversarial, errors. In particular, no conditions on the errors are imposed. A quantitative bound for the prediction error is given, relating it to the Rademacher complexity of the covariates, the norm of the minimum norm interpolator of the errors and the shape of the subdifferential around the true parameter. The general theory is illustrated with several examples: the sparse linear model with minimum $\ell_1$-norm or group Lasso penalty interpolation, the low rank trace regression model with nuclear norm minimization, and minimum Euclidean norm interpolation in the linear model. In case of sparsity or low-rank inducing norms, minimum norm interpolation yields a prediction error of the order of the average noise level, provided that the overparameterization is at least a logarithmic factor larger than the number of samples. Lower bounds that show near optimality of the results complement the analysis.
更多查看译文
关键词
robustness,minimum-norm
AI 理解论文
溯源树
样例
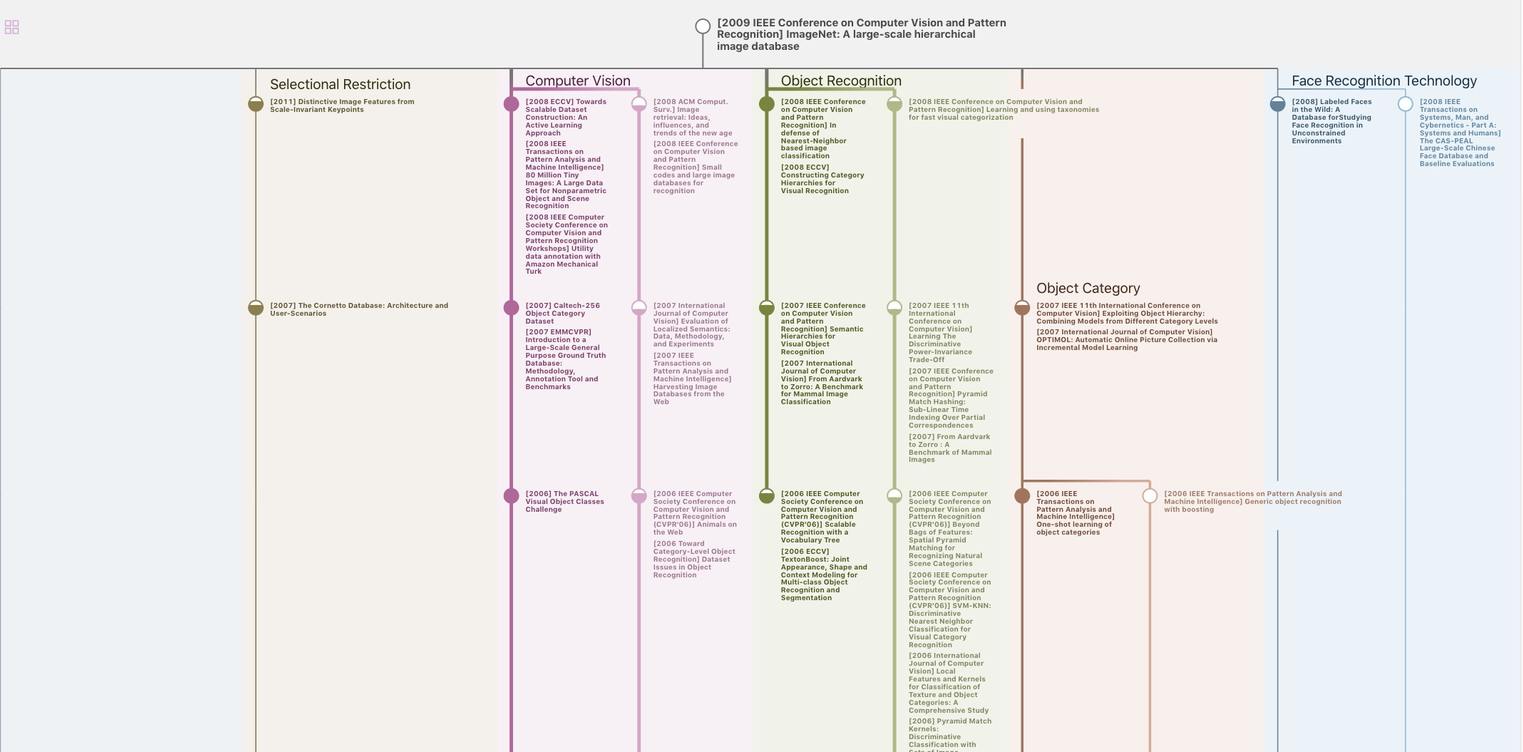
生成溯源树,研究论文发展脉络
Chat Paper
正在生成论文摘要