Recurrent Neural Hidden Markov Model for High-order Transition
ACM TRANSACTIONS ON ASIAN AND LOW-RESOURCE LANGUAGE INFORMATION PROCESSING(2022)
摘要
We propose a method to pay attention to high-order relations among latent states to improve the conventional HMMs that focus only on the latest latent state, since they assume Markov property. To address the high-order relations, we apply an RNN to each sequence of latent states, because the RNN can represent the information of an arbitrary-length sequence with their cell: a fixed-size vector. However, the simplest way, which provides all latent sequences explicitly for the RNN, is intractable due to the combinatorial explosion of the search space of latent states. Thus, we modify the RNN to represent the history of latent states from the beginning of the sequence to the current state with a fixed number of RNN cells whose number is equal to the number of possible states. We conduct experiments on unsupervised POS tagging and synthetic datasets. Experimental results show that the proposed method achieves better performance than previous methods. In addition, the results on the synthetic dataset indicate that the proposed method can capture the high-order relations.
更多查看译文
关键词
Neural networks,POS tagging
AI 理解论文
溯源树
样例
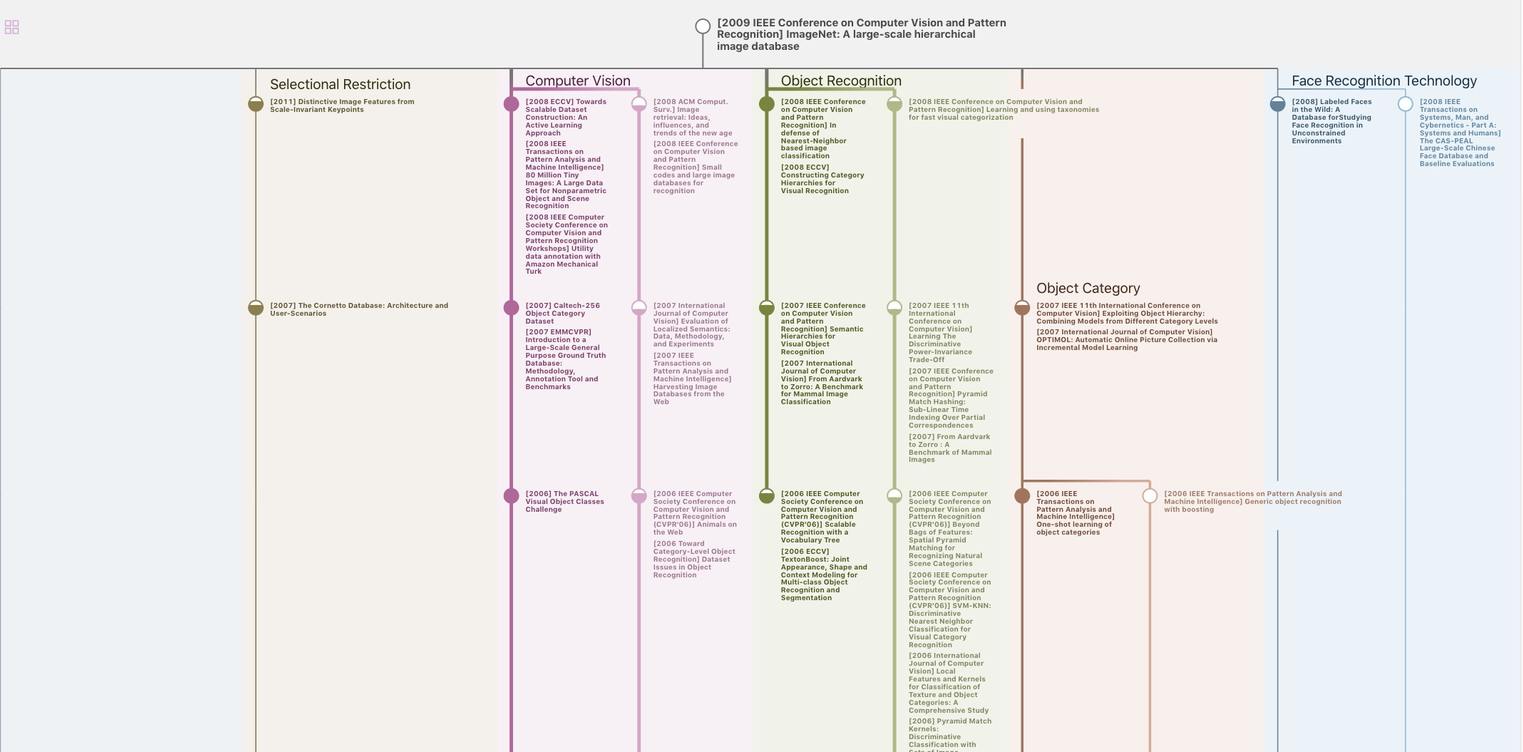
生成溯源树,研究论文发展脉络
Chat Paper
正在生成论文摘要