Grayscale medical image segmentation method based on 2D&3D object detection with deep learning
BMC MEDICAL IMAGING(2022)
摘要
Background Grayscale medical image segmentation is the key step in clinical computer-aided diagnosis. Model-driven and data-driven image segmentation methods are widely used for their less computational complexity and more accurate feature extraction. However, model-driven methods like thresholding usually suffer from wrong segmentation and noises regions because different grayscale images have distinct intensity distribution property thus pre-processing is always demanded. While data-driven methods with deep learning like encoder-decoder networks always are always accompanied by complex architectures which require amounts of training data. Methods Combining thresholding method and deep learning, this paper presents a novel method by using 2D&3D object detection technologies. First, interest regions contain segmented object are determined with fine-tuning 2D object detection network. Then, pixels in cropped images are turned as point cloud according to their positions and grayscale values. Finally, 3D object detection network is applied to obtain bounding boxes with target points and boxes’ bottoms and tops represent thresholding values for segmentation. After projecting to 2D images, these target points could composite the segmented object. Results Three groups of grayscale medical images are used to evaluate the proposed image segmentation method. We obtain the IoU (DSC) scores of 0.92 (0.96), 0.88 (0.94) and 0.94 (0.94) for segmentation accuracy on different datasets respectively. Also, compared with five state of the arts and clinically performed well models, our method achieves higher scores and better performance. Conclusions The prominent segmentation results demonstrate that the built method based on 2D&3D object detection with deep learning is workable and promising for segmentation task of grayscale medical images.
更多查看译文
关键词
Grayscale medical image, Image segmentation, Deep learning, Object detection, Point cloud
AI 理解论文
溯源树
样例
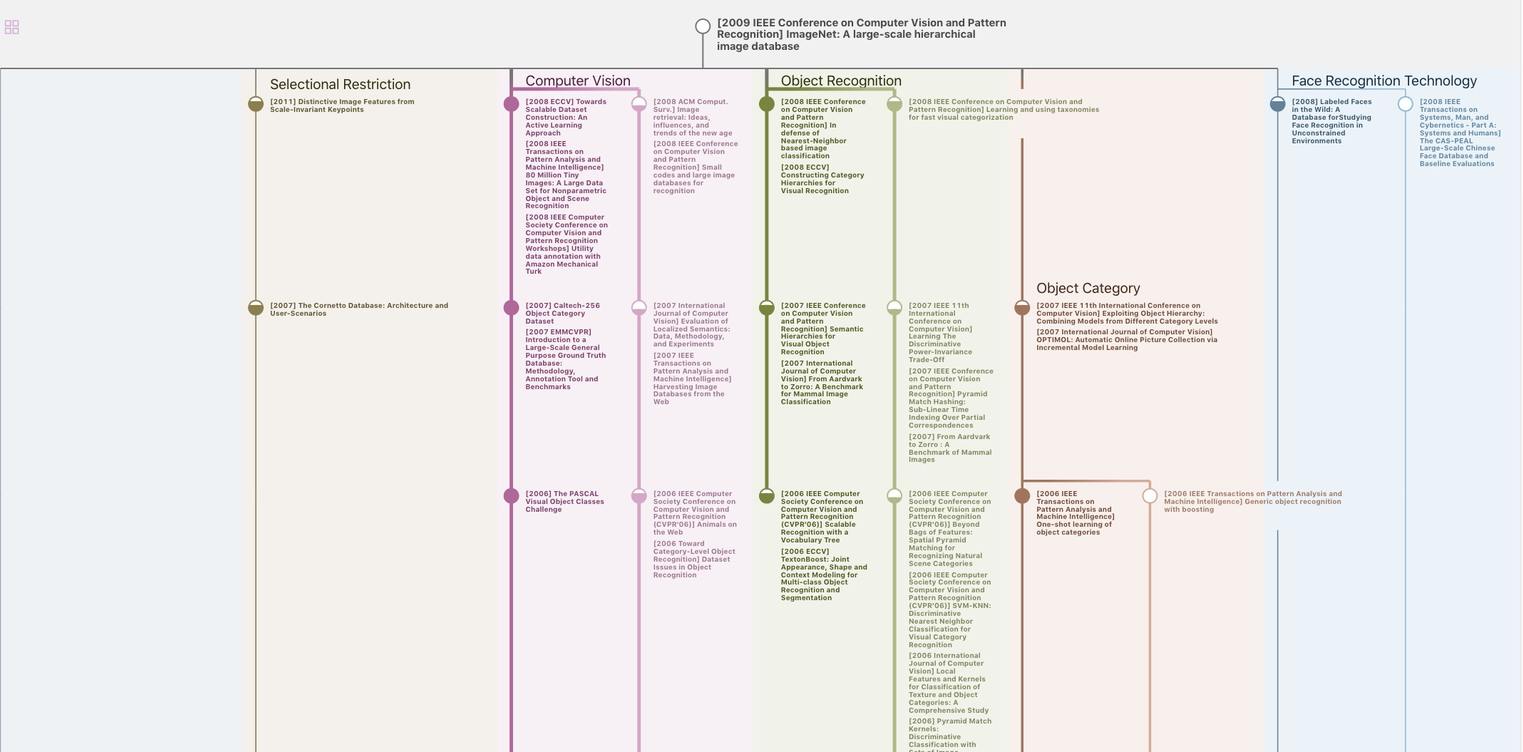
生成溯源树,研究论文发展脉络
Chat Paper
正在生成论文摘要