Use of U-Net Convolutional Neural Networks for Automated Segmentation of Fecal Material for Objective Evaluation of Bowel Preparation Quality in Colonoscopy
DIAGNOSTICS(2022)
摘要
Background: Adequate bowel cleansing is important for colonoscopy performance evaluation. Current bowel cleansing evaluation scales are subjective, with a wide variation in consistency among physicians and low reported rates of accuracy. We aim to use machine learning to develop a fully automatic segmentation method for the objective evaluation of the adequacy of colon preparation. Methods: Colonoscopy videos were retrieved from a video data cohort and transferred to qualified images, which were randomly divided into training, validation, and verification datasets. The fecal residue was manually segmented. A deep learning model based on the U-Net convolutional network architecture was developed to perform automatic segmentation. The performance of the automatic segmentation was evaluated on the overlap area with the manual segmentation. Results: A total of 10,118 qualified images from 119 videos were obtained. The model averaged 0.3634 s to segmentate one image automatically. The models produced a strong high-overlap area with manual segmentation, with 94.7% +/- 0.67% of that area predicted by our AI model, which correlated well with the area measured manually (r = 0.915, p < 0.001). The AI system can be applied in real-time qualitatively and quantitatively. Conclusions: We established a fully automatic segmentation method to rapidly and accurately mark the fecal residue-coated mucosa for the objective evaluation of colon preparation.
更多查看译文
关键词
artificial intelligence, automated segmentation, U-NET, colonoscopy, colonoscopy preparation quality
AI 理解论文
溯源树
样例
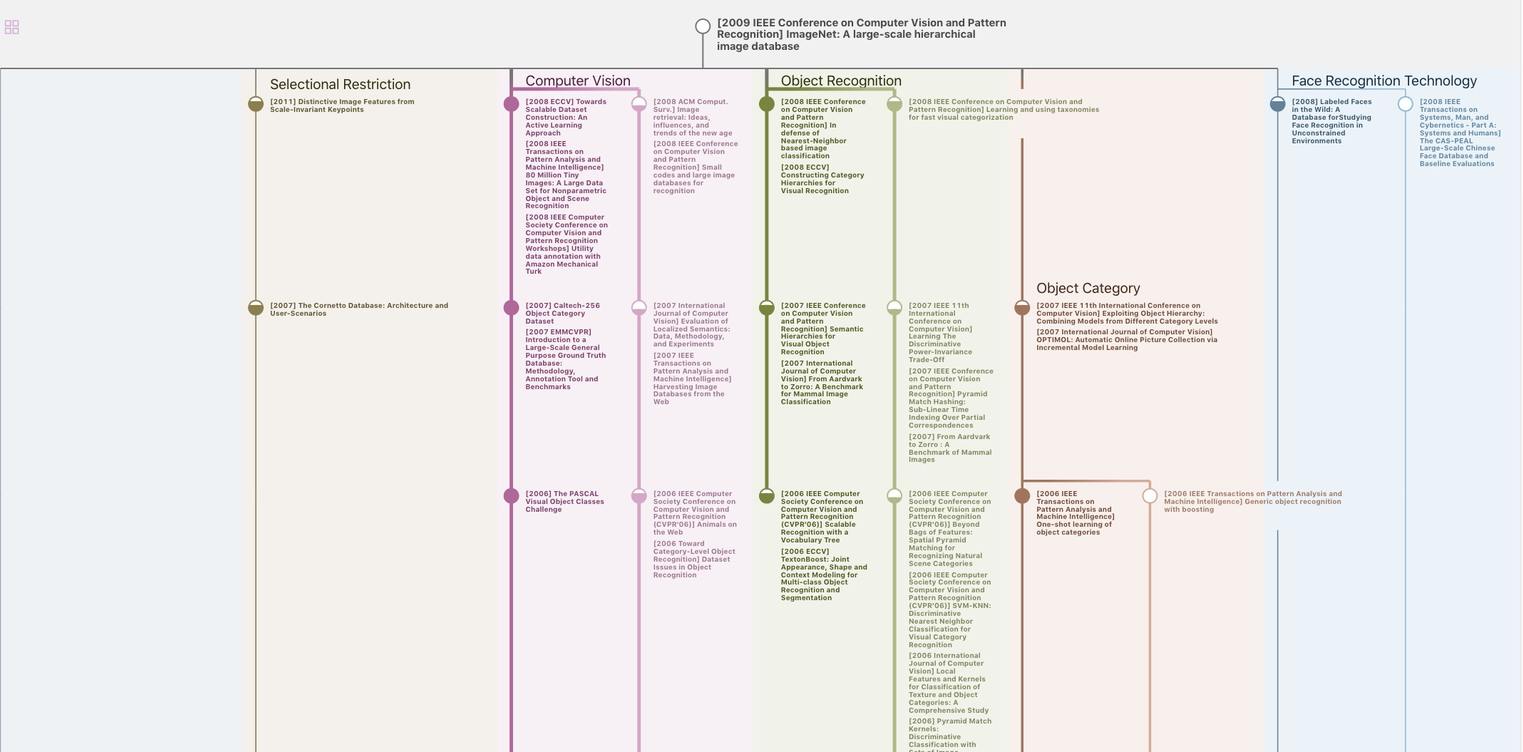
生成溯源树,研究论文发展脉络
Chat Paper
正在生成论文摘要