Comparison of data mining methods to predict mechanical properties of concrete with fly ash and alccofine
JOURNAL OF MATERIALS RESEARCH AND TECHNOLOGY-JMR&T(2021)
摘要
In order to predict the influence of fly ash (FA) and Alccofine (AL) on the compressive strength (CS), flexural strength (FS), split tensile strength (STS), and rapid chloride permeability test (RCPT) of concrete in a different age of samples, an experimental-based dataset was selected. This research was carried out by investigating the forecasting ac-curacy of different data mining (DM) models. To gain this, a score-based system is pro-posed to compare the applied method's productivity based on R, RMSE, and MAE results. As shown in the results, CS, FS, STS, and RCPT's predicted values are very close to the experimental values in both training and testing samples. By considering the highest R among the developed models, the MLP model was the most efficient algorithm, at 0.9983, 0.9981, and 0.985 to predict CS, FS, and STS, respectively. Regarding RCPT results, Additive regression algorithm has the highest accuracy with TRS at 53. According to the ranking score, for prediction all four mechanical properties of concrete modified with FA and AL, among the applied models, SMOreg has the lowest rank, and GPR could be recognized as the second-best method. (c) 2021 Published by Elsevier B.V. This is an open access article under the CC BY-NC-ND license (http://creativecommons.org/licenses/by-nc-nd/4.0/).
更多查看译文
关键词
Fly ash,Alccofine,Machine learning,Data mining,Compressive strength,Split tensile strength,Flexural strength,Rapid chloride permeability test,Comparison
AI 理解论文
溯源树
样例
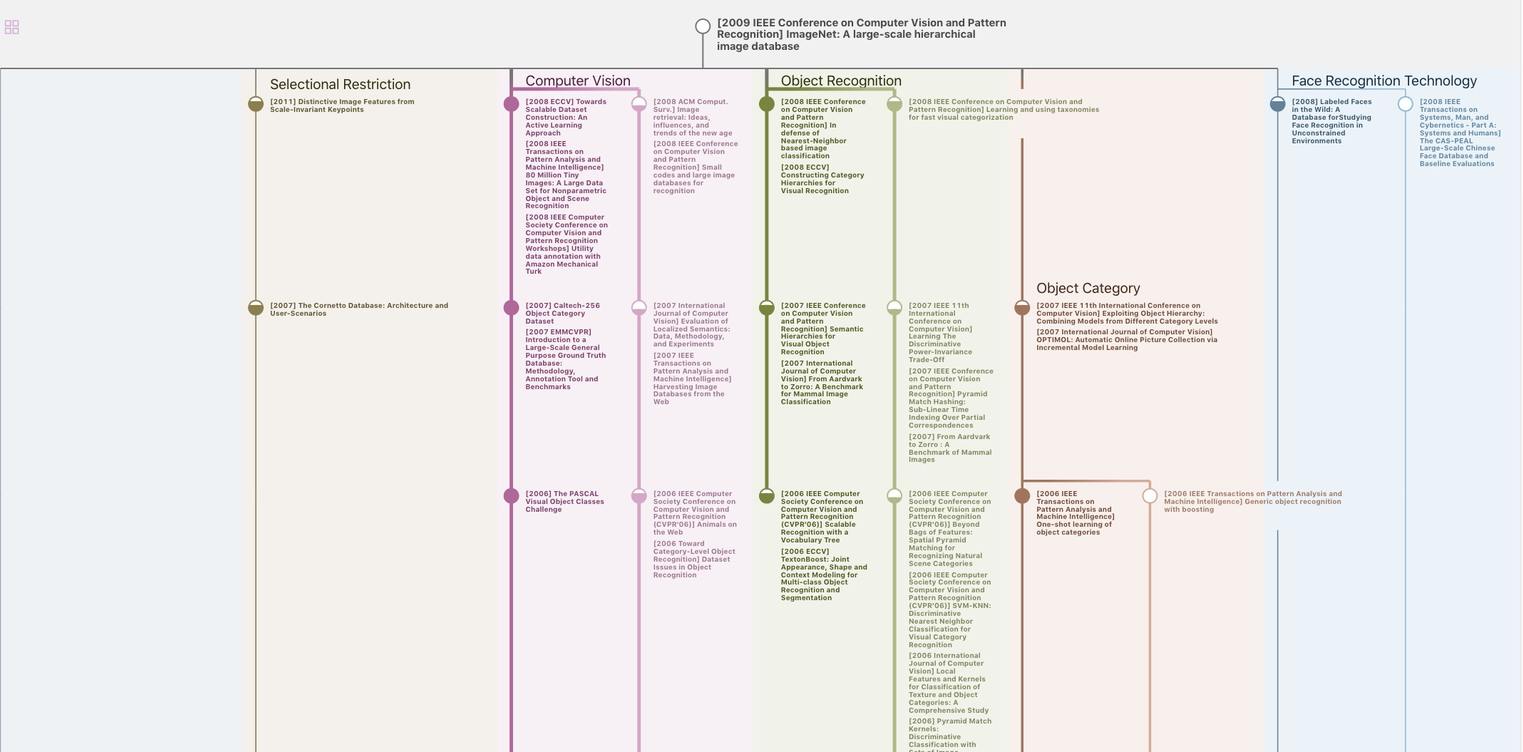
生成溯源树,研究论文发展脉络
Chat Paper
正在生成论文摘要