Unsupervised Testing of NLU models with Multiple Views
semanticscholar(2021)
摘要
In Natural Language Understanding (NLU) systems in voice assistants, new domains are added on a regular basis. This poses the practical problem of evaluating the performance of NLU models on domains where no manually annotated data is available. In this paper, we present an unsupervised testing method that we call Cross-View Testing (CVT) for ranking multiple intent classification models using only unlabeled test data. The approach relies on a number of labeling functions to automatically annotate test data in the target domain. The labeling functions include intent classification models trained on different domains, as well as heuristic rules. Specifically, we combine the annotations of multiple models with different output spaces by training a combiner model on synthetic data. In our experiments, the proposed model outperforms the target models by very large margins, and its predictions can be used as a proxy of ground truth for unsupervised model evaluation.
更多查看译文
AI 理解论文
溯源树
样例
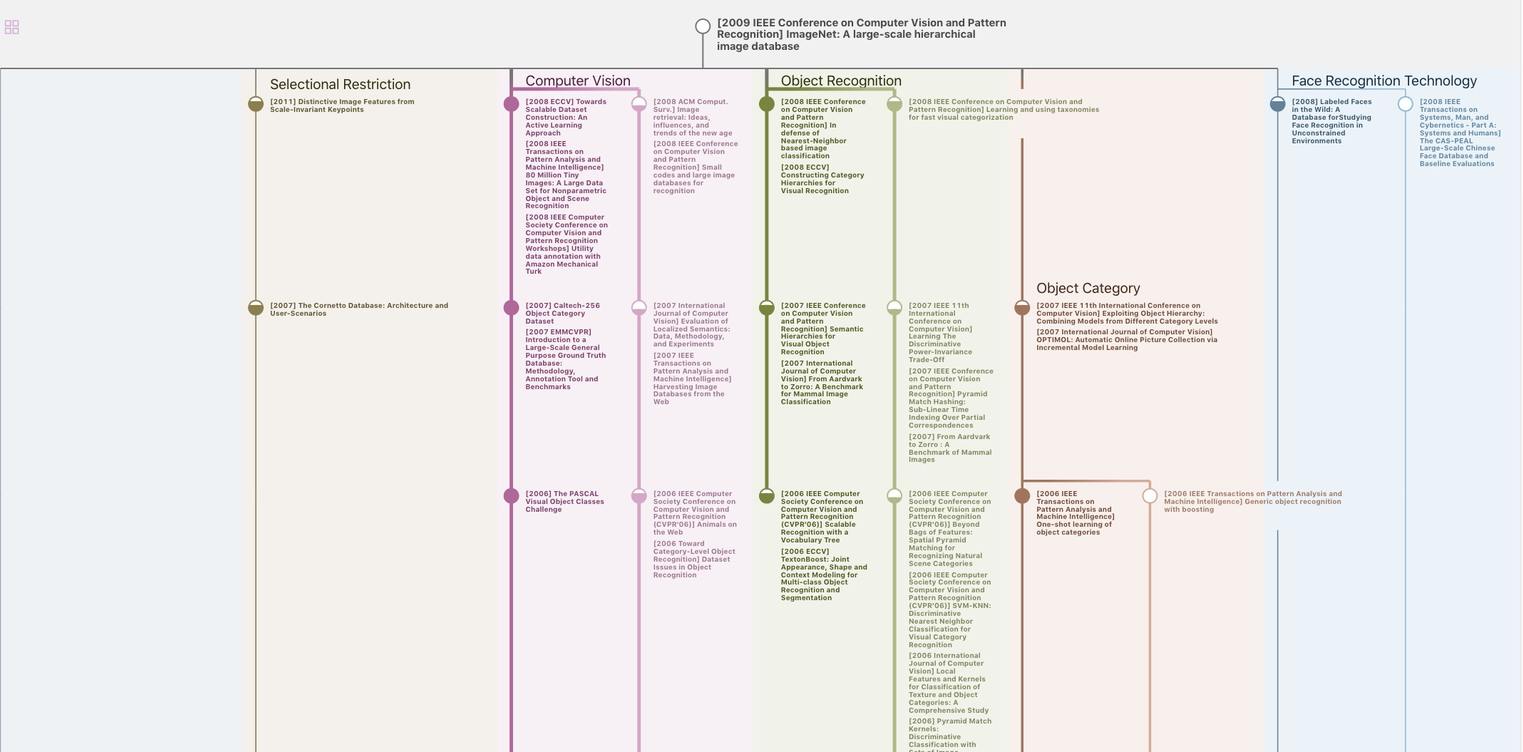
生成溯源树,研究论文发展脉络
Chat Paper
正在生成论文摘要