Active learning of deep surrogates for pdes
semanticscholar(2021)
摘要
Surrogate models for partial-differential equations are widely used in the design of metamaterials to rapidly evaluate the behavior of composable components. However, the training cost of accurate surrogates by machine learning can rapidly increase with the number of variables. We present an active learning algorithm and apply it to train deep surrogates of Helmholtz’s equation and linear elasticity in solid mechanics. For the two problems of interest, our algorithm reduces the number of simulations required compared to uniform random samples by more than an order of magnitude for a neural-network surrogate model and by four, respectively. Results show that the surrogate evaluation is faster than a direct solve by over two orders of magnitude and over five orders of magnitude, respectively.
更多查看译文
AI 理解论文
溯源树
样例
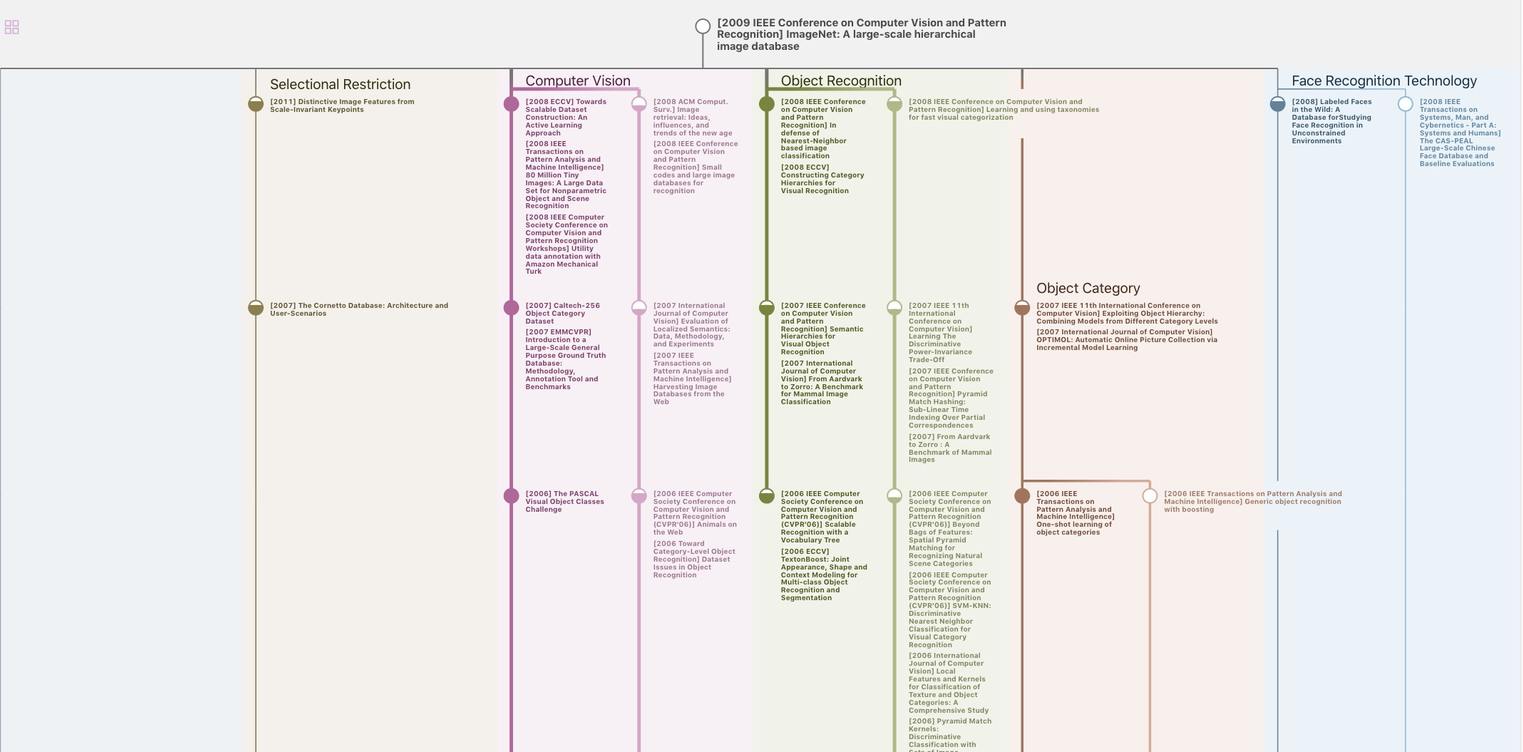
生成溯源树,研究论文发展脉络
Chat Paper
正在生成论文摘要