Supplementary Material: Quasi-Dense Similarity Learning for Multiple Object Tracking
semanticscholar(2021)
摘要
tracker=dict( type=’QuasiDenseEmbedTracker’, # score threshold to start a new track init_score_thr=0.8, # score threshold to continue a track obj_score_thr=0.5, # score threshold for data association match_score_thr=0.5, # number of frames to keep tracks memo_tracklet_frames=10, # number of frames to keep backdrops memo_backdrop_frames=1, # momentum to update the embeddings memo_momentum=0.8, # duplicate removal to tackle multi-targets cases nms_backdrop_iou_thr=0.3, nms_class_iou_thr=0.7, # the matching metric match_metric=’bisoftmax’) Dataset specific parameters. Our object association only relies on appearance, so it is robust to different motion patterns in different datasets. The experiments share the same tracking parameters except TAO, because TAO uses 3D mAP, instead of CLEAR MOT metrics, for evaluation. On TAO, the terms “init_score_thr" and “obj_score_thr" are set to 0.0001 to obtain a high recall. Considering the numerous tracks with these thresholds, we do not maintain backdrops in these experiments.
更多查看译文
AI 理解论文
溯源树
样例
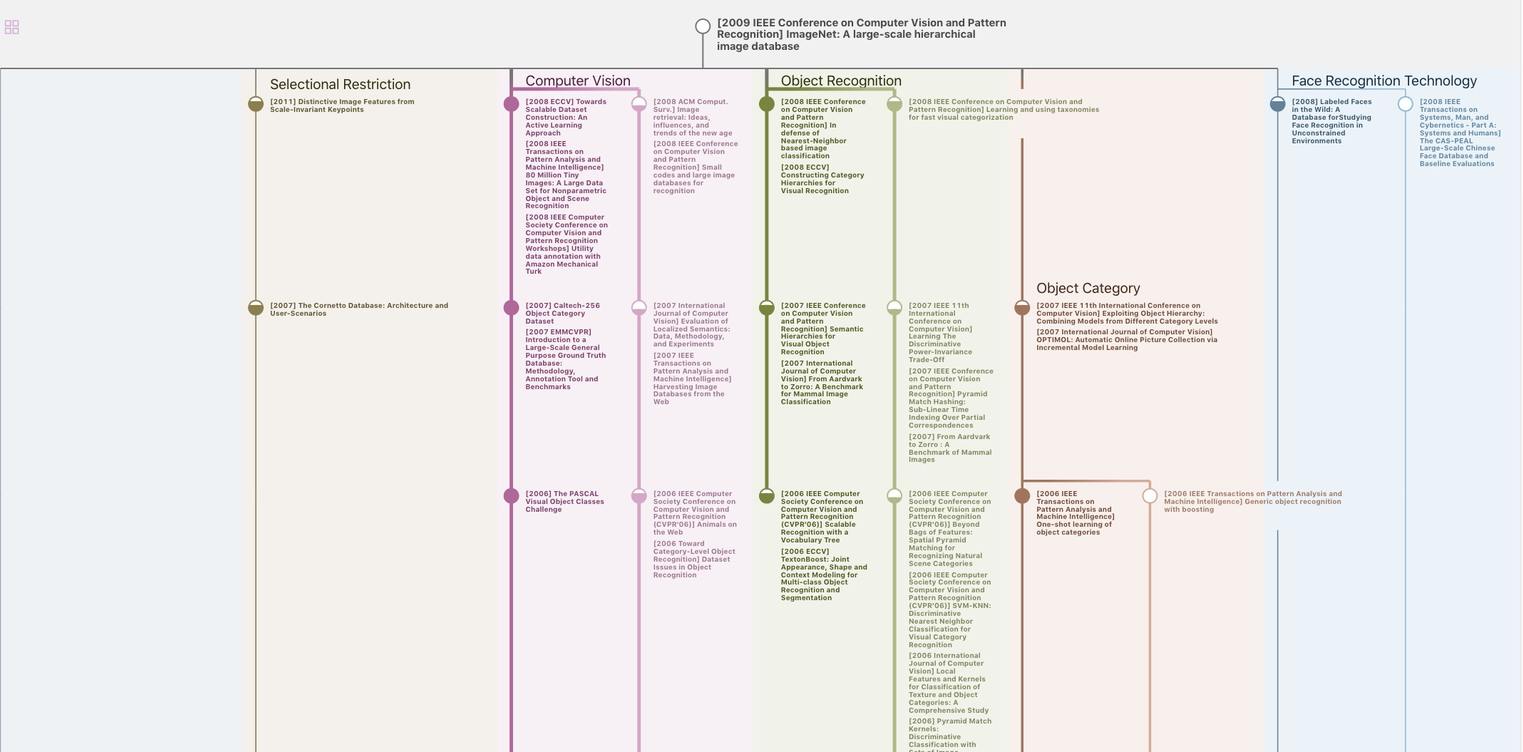
生成溯源树,研究论文发展脉络
Chat Paper
正在生成论文摘要