A geometric deep learning approach to predict binding conformations of bioactive molecules
Nature Machine Intelligence(2021)
摘要
Understanding the interactions formed between a ligand and its molecular target is key to guiding the optimization of molecules. Different experimental and computational methods have been applied to better understanding these intermolecular interactions. Here we report a method based on geometric deep learning that is capable of predicting the binding conformations of ligands to protein targets. The model learns a statistical potential based on the distance likelihood, which is tailor-made for each ligand–target pair. This potential can be coupled with global optimization algorithms to reproduce the experimental binding conformations of ligands. We show that the potential based on distance likelihood, described here, performs similarly or better than well-established scoring functions for docking and screening tasks. Overall, this method represents an example of how artificial intelligence can be used to improve structure-based drug design.
更多查看译文
关键词
Cheminformatics,Computational models,Machine learning,Engineering,general
AI 理解论文
溯源树
样例
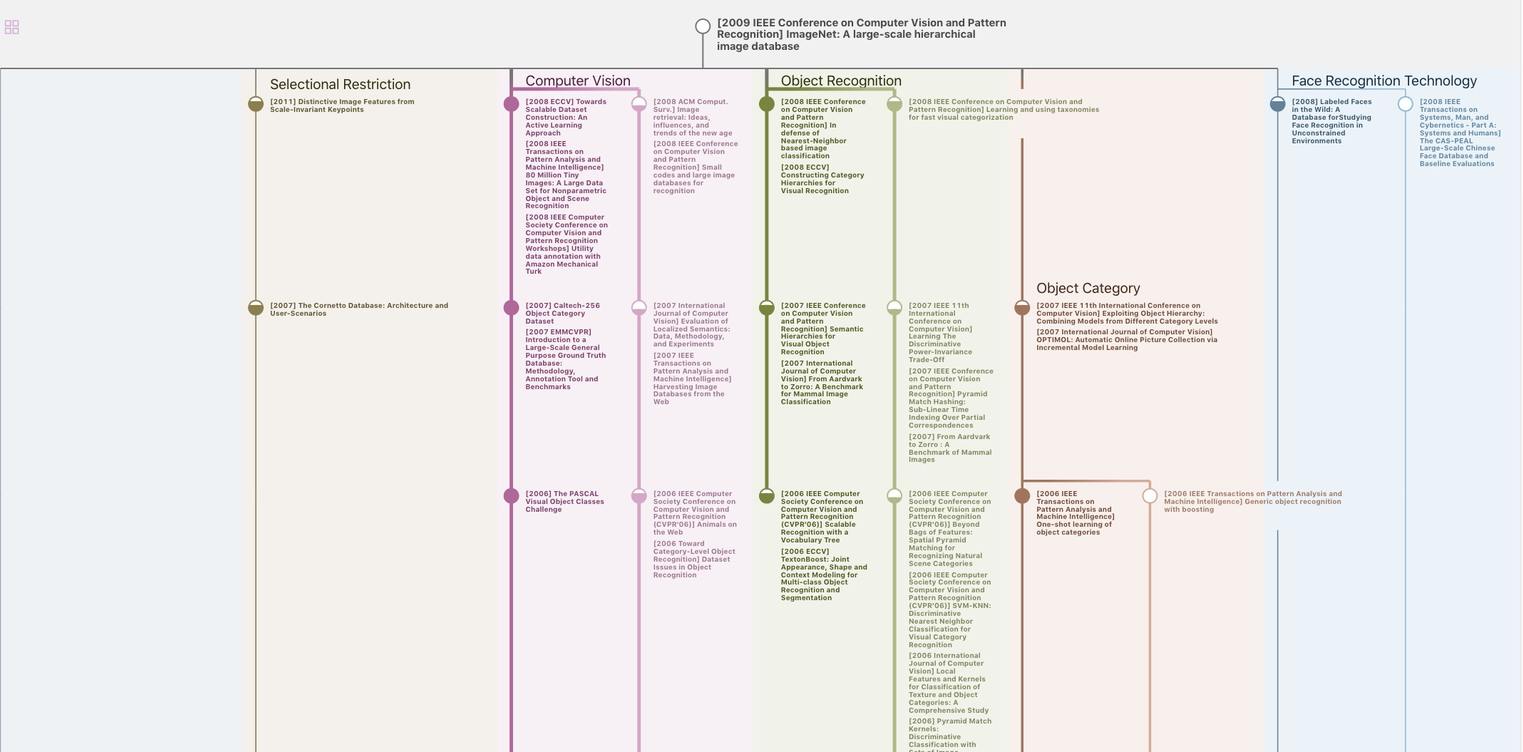
生成溯源树,研究论文发展脉络
Chat Paper
正在生成论文摘要