Data Augmentation Methods for Reject Inference in Credit Risk Models
semanticscholar(2021)
摘要
A significant challenge in credit risk models for underwriting is data representativeness. When credit scoring models are built using only applicants who have been accepted for credit which is the common strategy in the industry, such nonrandom sampling mainly influenced by credit policy makers and previous loan performances may introduce sampling bias to the estimated credit models and accordingly influence the models’ prediction of default on loan payment when screening applications from all borrowers. In this paper, we proposed two data augmentation methods that aim to identify and pseudo-label parts of the declined loan applications based on the confidence level of the estimated labels to mitigate sampling bias in the training data. Besides prevalent model performance metrics, we also reported loan application approval rates at various loan default rate intervals from the business perspective. Our proposed methods were compared to the original supervised model and the traditional reject inference method using fuzzy augmentation. The results showed that self-training model with calibrated probability as data augmentation selection criteria improved the ability of credit score to differentiate good/bad loan applications and, more importantly, increased loan approval rate by 2.6% while keeping similar default rate comparing to the KGB model. The results demonstrate practical implications on how future underwriting model development process should follow.
更多查看译文
AI 理解论文
溯源树
样例
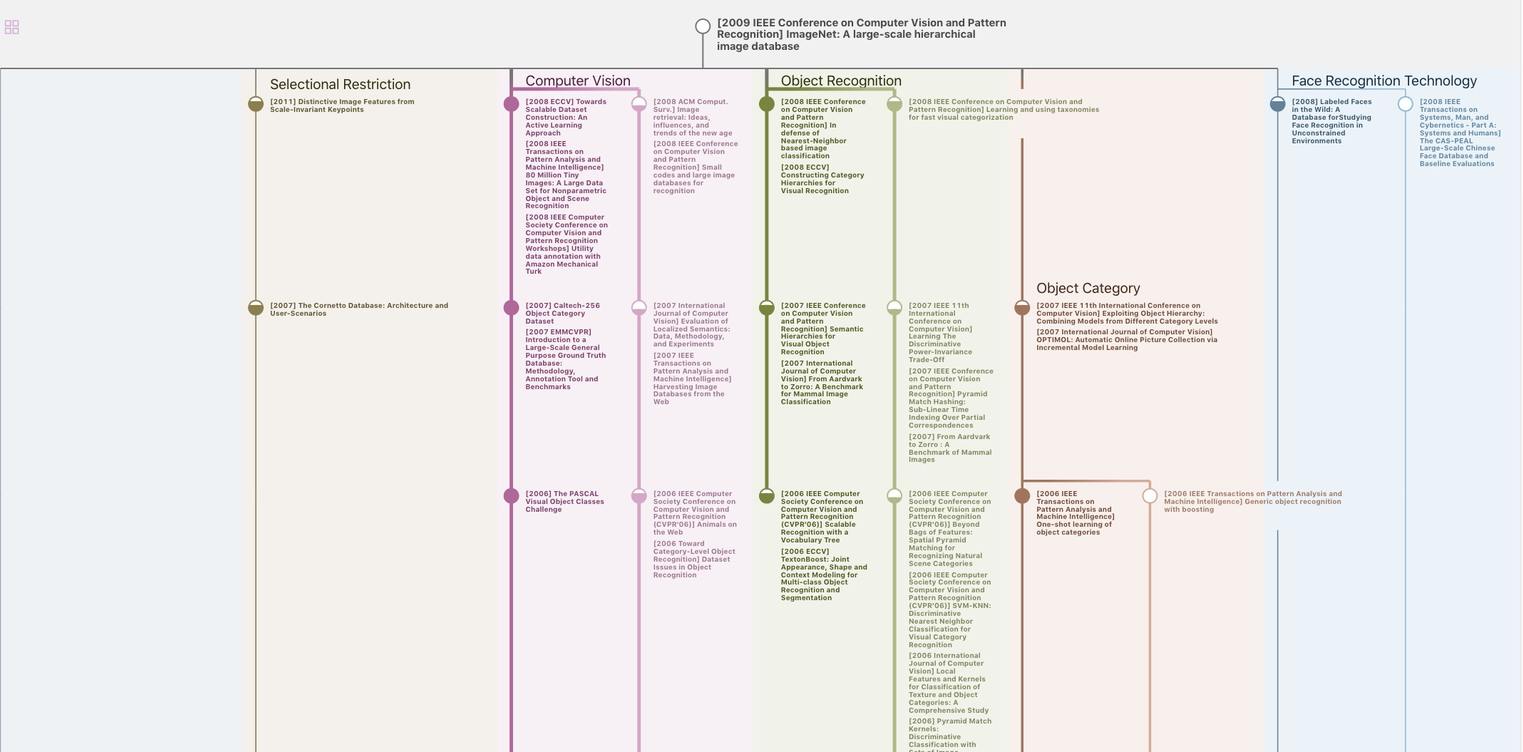
生成溯源树,研究论文发展脉络
Chat Paper
正在生成论文摘要