Compositional perturbation autoencoder for single-cell response modeling
bioRxiv (Cold Spring Harbor Laboratory)(2021)
摘要
AbstractRecent advances in multiplexing single-cell transcriptomics across experiments are enabling the high-throughput study of drug and genetic perturbations. However, an exhaustive exploration of the combinatorial perturbation space is experimentally unfeasible, so computational methods are needed to predict, interpret and prioritize perturbations. Here, we present the Compositional Perturbation Autoencoder (CPA), which combines the interpretability of linear models with the flexibility of deep-learning approaches for single-cell response modeling. CPA encodes and learns transcriptional drug response across different cell types, doses, and drug combinations. The model produces easy-to-interpret embeddings for drugs and cell types, allowing drug similarity analysis and predictions for unseen dosages and drug combinations. We show CPA accurately models single-cell perturbations across compounds, dosages, species, and time. We further demonstrate that CPA predicts combinatorial genetic interactions of several types, implying it captures features that distinguish different interaction programs. Finally, we demonstrate CPA allows in-silico generation of 5,329 missing combinations (97.6% of all possibilities) with diverse genetic interactions. We envision our model will facilitate efficient experimental design by enabling in-silico response prediction at the single-cell level.
更多查看译文
关键词
compositional perturbation autoencoder,single-cell
AI 理解论文
溯源树
样例
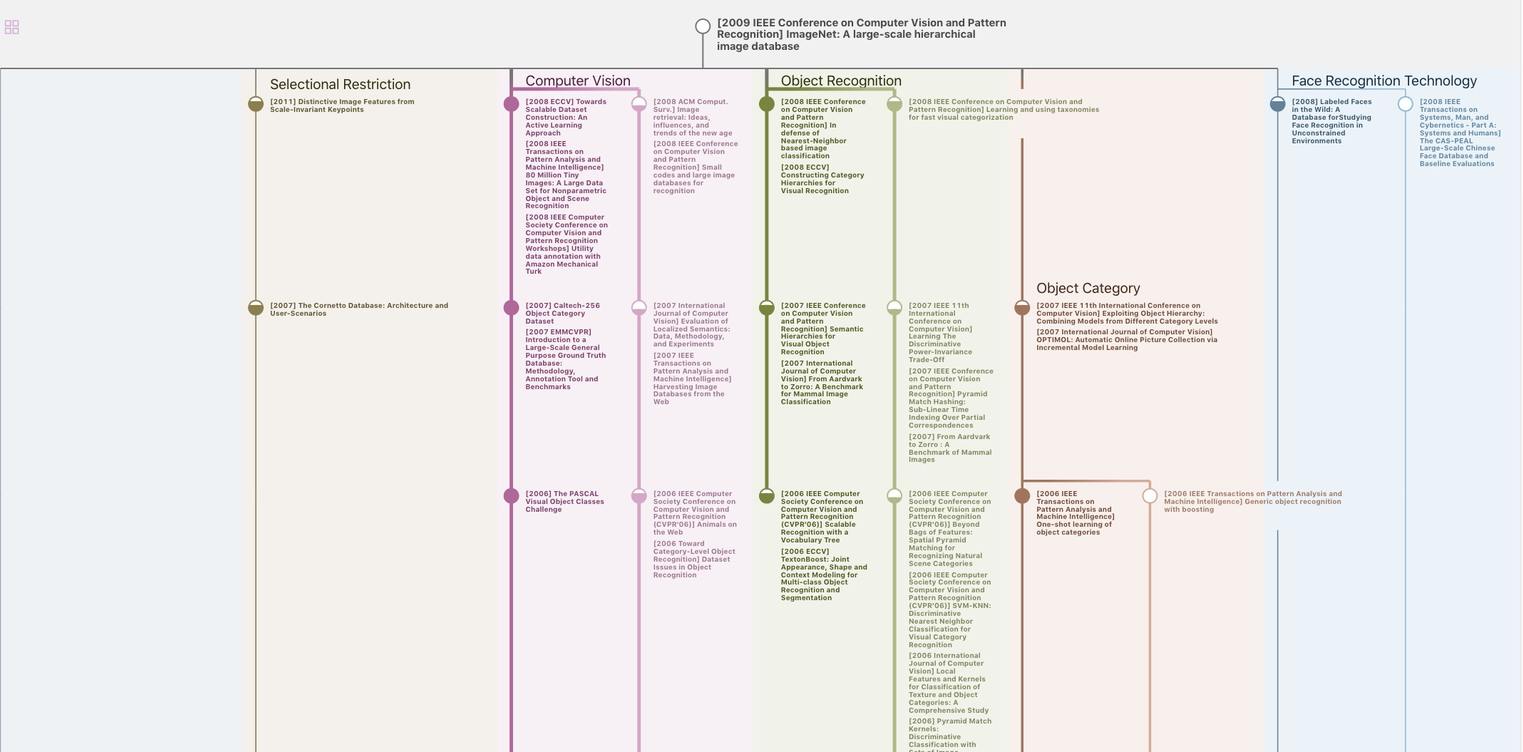
生成溯源树,研究论文发展脉络
Chat Paper
正在生成论文摘要