J ul 2 01 1 Sublinear Time , Measurement-Optimal , Sparse Recovery ForAll
semanticscholar(2021)
摘要
An approximate sparse recovery s stem inl1 norm makes a small number of measurements of a noisy vector with at most k large entries and recovers those h avy hittersapproximately. Formally, it consists of parameters N, k, ǫ, anm-by-N measurement matrix , Φ, and adecoding algorithm,D. Given a vector,x, wherexk denotes the optimal k-term approximation tox, the system approximates x by x̂ = D(Φx), which must satisfy ‖x̂− x‖ 1 ≤ (1 + ǫ) ‖x− xk‖1 . Among the goals in designing such systems are minimizing the numberm of measurements and the runtime of the decoding algorithm, D. We consider the “forall” model, in which a single matrix Φ, possibly “constructed” non-explicitly using the probabil ist c method, is used for all signals x. Many previous papers have provided algorithms for this prob lem. But all such algorithms that use the optimal numberm = O(k log(N/k)) of measurements require superlinear time Ω(N log(N/k)). In this paper, we give the first algorithm for this problem that uses t he optimum number of measurements (up to constant factors) and runs in sublinear time o(N) whenk is sufficiently less thanN . Specifically, for any positive integer l, our approach uses time O(lǫk(N/k)) and usesm = O(lǫk log(N/k)) measurements, with access to a data structure requiring spa ce and preprocessing time O(lNk/ǫ). Bar Ilan University. Email:porately@gmail.com University of Michigan. Supported in part by NSF CCF 0743372 and DARPA/ONR N66001-08-1-2065. Email: martinjs@eecs.umich.edu
更多查看译文
AI 理解论文
溯源树
样例
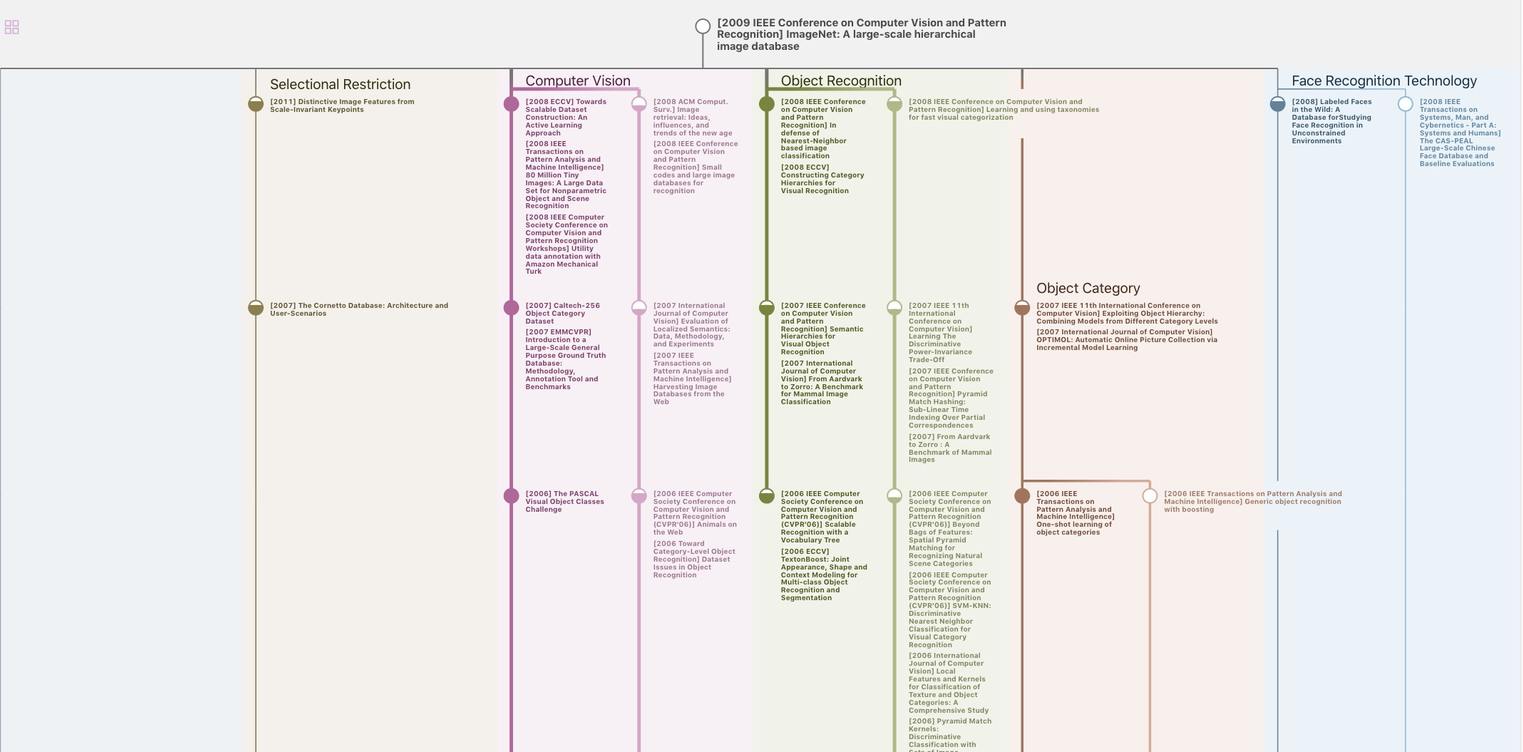
生成溯源树,研究论文发展脉络
Chat Paper
正在生成论文摘要