Predicting the Oxidant Demand in Full-Scale Water Treatment using an Artificial Neural Network: Uncertainty and Sensitivity Analysis
semanticscholar(2019)
摘要
Drinking Water Treatment Plants face changes in raw water quality and quantity and the treatment needs to be adjusted accordingly to produce the best water quality at the minimum environmental cost. The amount of data generated along drinking water treatment plants allows developing data-based models like artificial neural networks that are able to predict operational parameters and can be incorporated into environmental decision support systems. In the present study, an artificial neural network is developed for predicting the potassium permanganate demand at the inlet of a full-scale Drinking Water Treatment Plant. A systematic methodology is carried out for outlier detection and removal from the original dataset. Afterwards, model parameters estimation, uncertainty and sensitivity analysis is reported to assess prediction quality and uncertainty of the models. Bootstrap method was used for parameter estimation, and uncertainty of the inputs onto the model outputs was propagated using a Monte Carlo scheme. Several sensitivity analysis methods were evaluated to understand the contribution of the inputs on the output of the models, and this was in accordance with the knowledge of the process and other studies found in the literature. The selected architecture consisted of a feed-forward multi-layer perceptron with four inputs and one node in the hidden layer with a sigmoid activation function. The mean absolute error of the resulting model is 0.128 mg·L-1, which was considered acceptable by the DWTP operators. The resulting model provided good results in terms of replicative, predictive and structural performance and is to be used for supporting decisionmaking in the daily operation of the plant.
更多查看译文
AI 理解论文
溯源树
样例
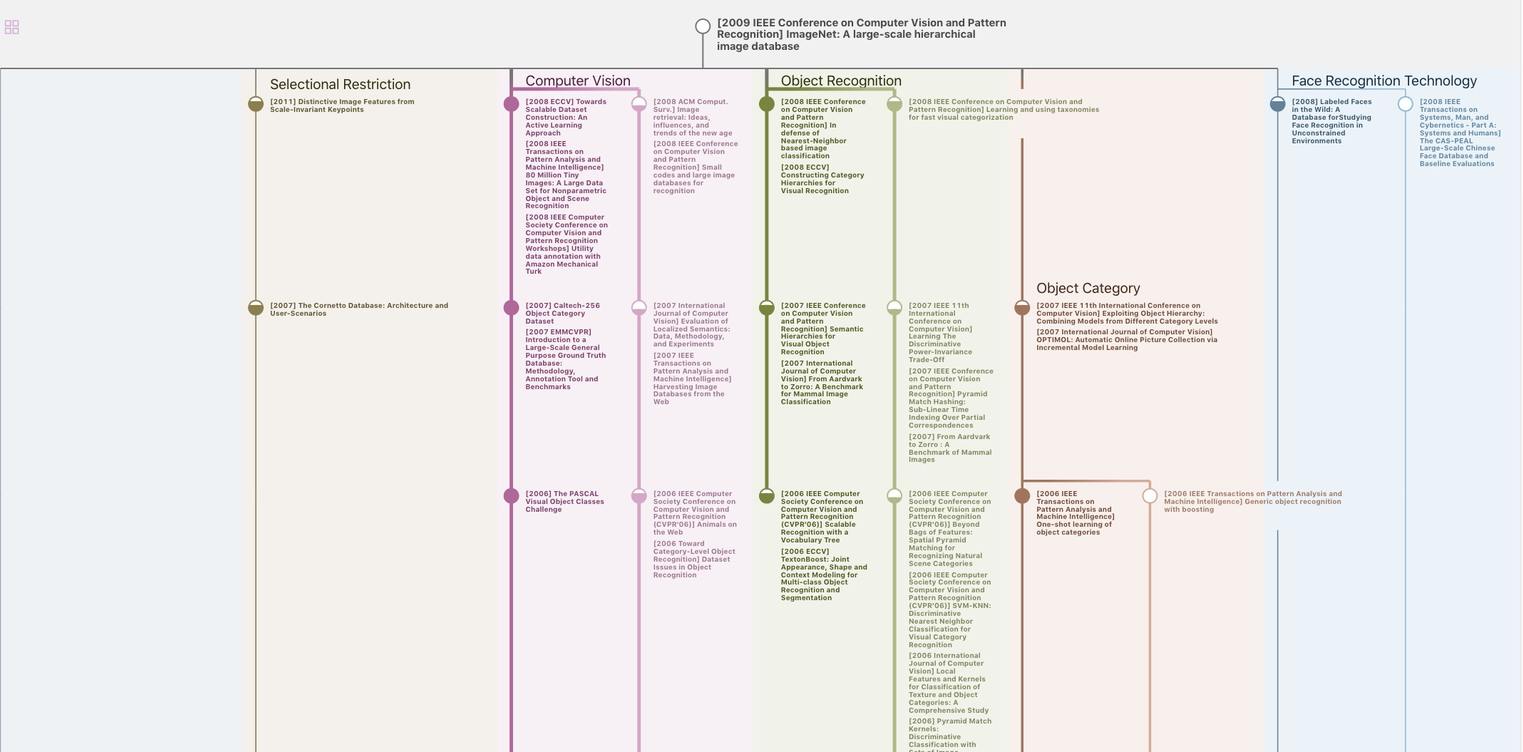
生成溯源树,研究论文发展脉络
Chat Paper
正在生成论文摘要