Interpretability of statistical approaches in speech and language neuroscience
semanticscholar(2019)
摘要
Brain functions are traditionally investigated using general linear models (GLM) in controlled experiments, which allow for a straightforward interpretation of the data. However, constraints imposed by controlled experimental setups have led neuroscientists to increasingly use machine-learning inspired approaches, which allow to work with large datasets collected in relatively natural experimental settings. Given the disparity of available methods and underlying concepts, synthesizing and relating the results of both traditional and machine-learning inspired experiments represents a substantial challenge. In this review, we propose to use a taxonomy based on the spatial specificity of the available methods and the extent to which they are constrained by a priori hypotheses, to classify their interpretability. Taking speech processing as a case example, we dissect the advantages and limitations of each of these methodological approaches, and highlight the ways in which they can be fruitfully combined to facilitate data interpretation.
更多查看译文
AI 理解论文
溯源树
样例
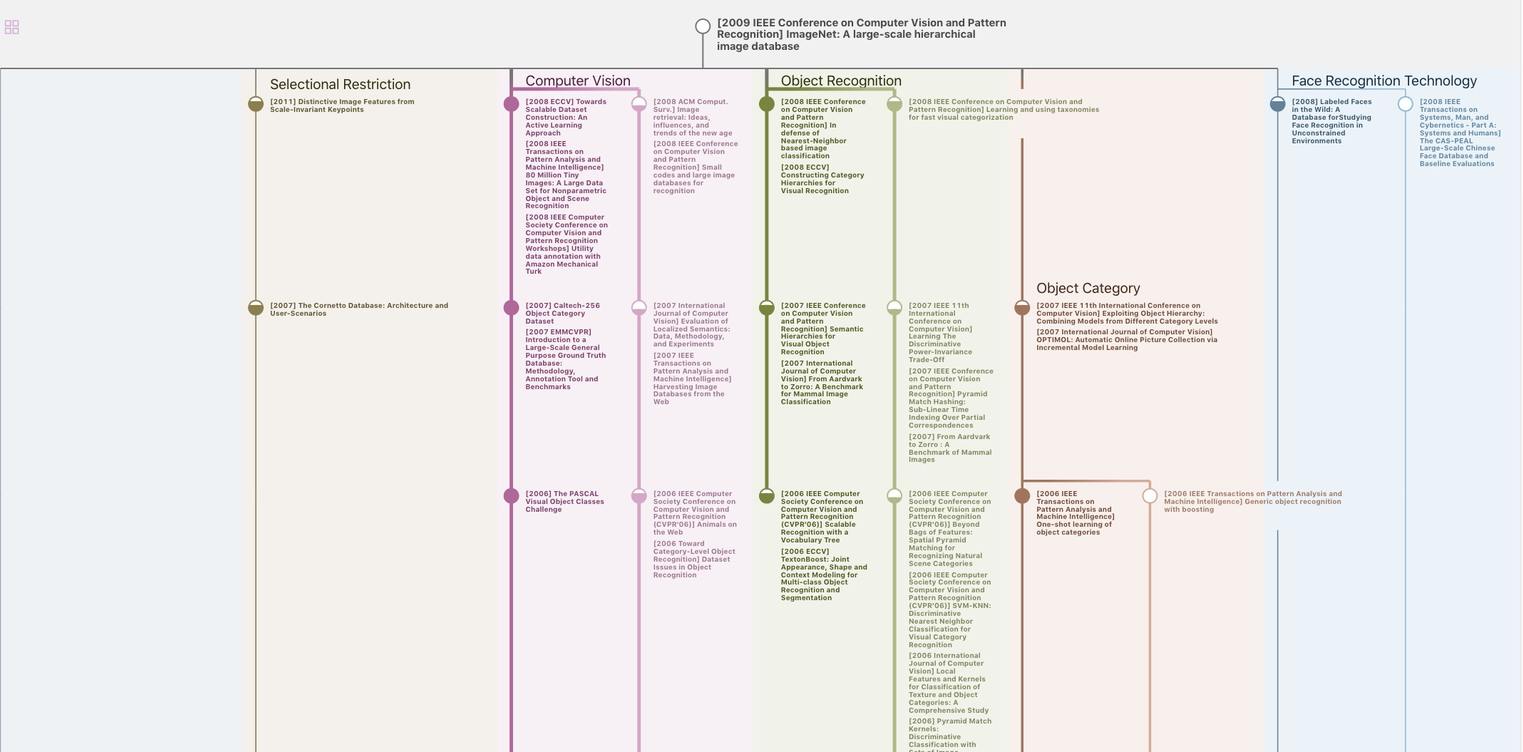
生成溯源树,研究论文发展脉络
Chat Paper
正在生成论文摘要