Spatio-Temporal SAR-Optical Data Fusion for Cloud Removal via a Deep Hierarchical Model
semanticscholar(2021)
摘要
The abundance of clouds, located both spatially and temporally, often makes remote sensing (RS) applications with optical images difficult or even impossible to perform. Traditional cloud removing techniques have been studied for years, and recently, Machine Learning (ML)-based approaches have also been considered. In this manuscript, a novel method for the restoration of clouds-corrupted optical images is presented, able to generate the whole optical scene of interest, not only the cloudy pixels, and based on a Joint Data Fusion paradigm, where three deep neural networks are hierarchically combined. Spatio-temporal features are separately extracted by a conditional Generative Adversarial Network (cGAN) and by a Convolutional Long Short-Term Memory (ConvLSTM), from Synthetic Aperture Radar (SAR) data and optical time-series of data respectively, and then combined with a U-shaped network. The use of time-series of data has been rarely explored in the state of the art for this peculiar objective, and moreover existing models do not combine both spatio-temporal domains and SARoptical imagery. Quantitative and qualitative results have shown a good ability of the proposed method in producing cloud-free images, by also preserving the details and outperforming the cGAN and the ConvLSTM when individually used. Both the code and the dataset have been implemented from scratch and made available to interested researchers for further analysis and investigation.
更多查看译文
关键词
cloud removal,fusion,spatio-temporal,sar-optical
AI 理解论文
溯源树
样例
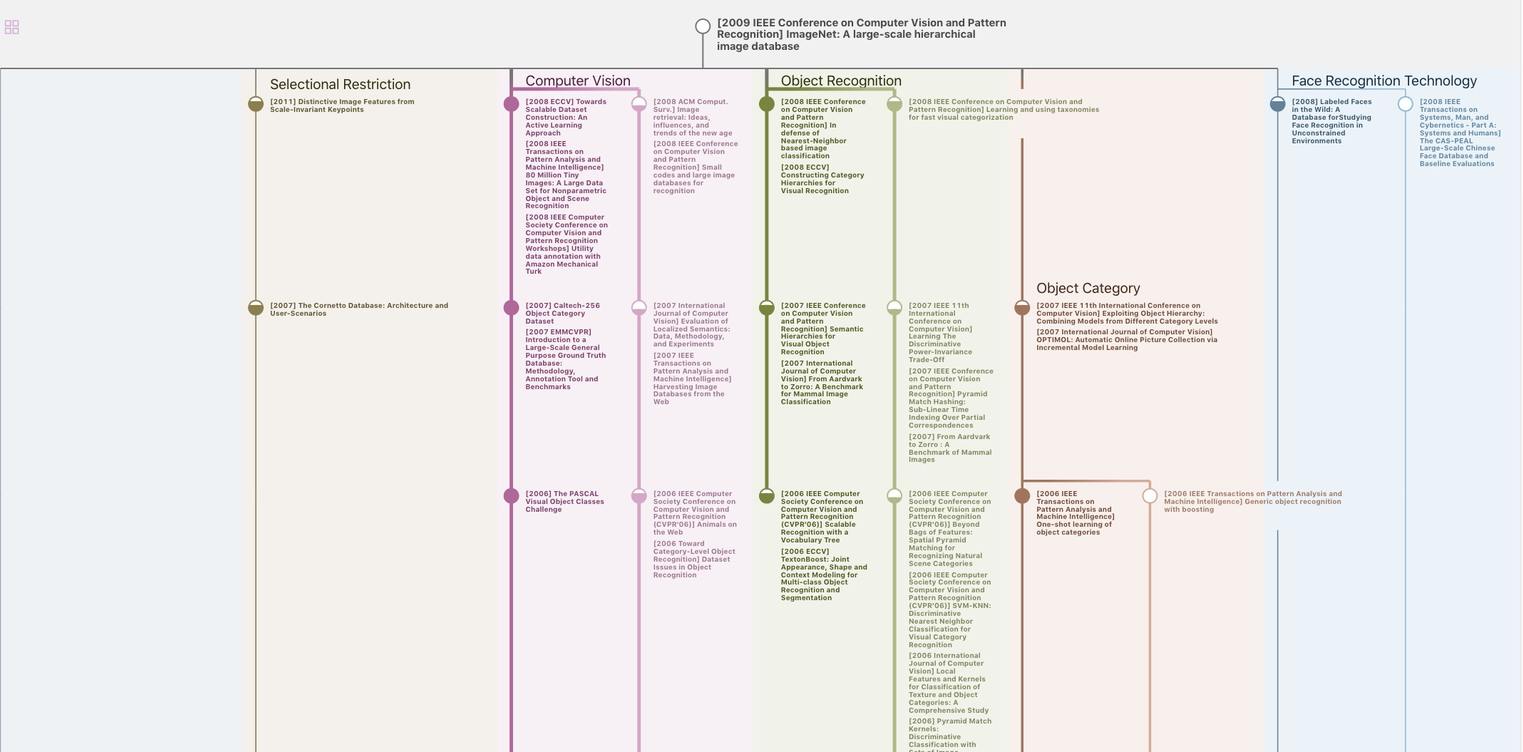
生成溯源树,研究论文发展脉络
Chat Paper
正在生成论文摘要