Infinite Markov pooling of predictive distributions
Journal of Econometrics(2022)
摘要
This paper introduces novel approaches to forecast pooling methods based on a nonparametric prior for a weight vector combining predictive densities. The first approach places a Dirichlet process prior on the weight vector and generalizes the static linear pool. The second approach uses a hierarchical Dirichlet process prior to allow the weight vector to follow an infinite hidden Markov chain. This generalizes dynamic prediction pools to the nonparametric setting. Efficient posterior simulation based on MCMC methods is also examined. Detailed applications to short-term interest rates, realized covariance matrices and asset pricing models demonstrate that the nonparametric pool forecasts well. The paper concludes with extensions and an application for calibrating and combining predictive densities.
更多查看译文
关键词
Prediction pools,Dirichlet process,Beam sampling,Infinite Markov switching,Density forecast,Short-term interest rates,Realized covariance matrices
AI 理解论文
溯源树
样例
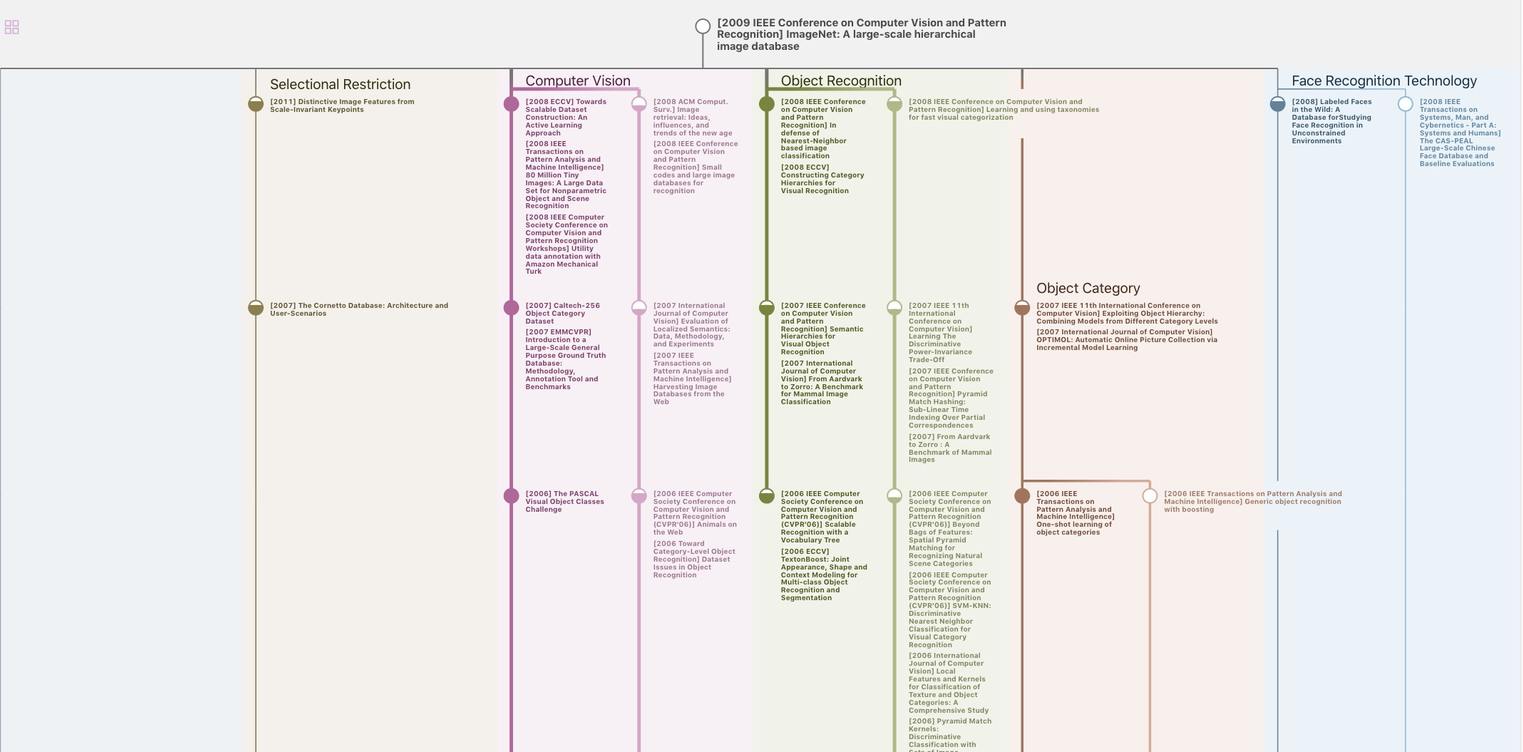
生成溯源树,研究论文发展脉络
Chat Paper
正在生成论文摘要