Deep Sensing Approach to Single-Sensor Bridge Weighing in Motion
semanticscholar(2018)
摘要
Bridge weighing in motion (BWIM) is a system that weighs vehicles crossing a road bridge without stopping them. It is used to calculate structural damage by detecting overloads. To achieve accurate weighing, vehicle properties such as lane, speed, trajectory, and number of axles must be estimated in advance. Conventionally, those have been obtained by additional devices on the road, such as optical or pavement sensors. To make an inexpensive BWIM system free from maintenance, nothing-on-road sensors have been proposed that detect vehicles without sensors upon the road. The accepted practice of installing multiple sensors at multiple points underneath the road slab for vehicle detection requires synchronization between sensors, and is less applicable to short bridges. The possibility of extracting richer information from a single sensor has been neglected. In this paper, we propose a novel vehicle detection technique for a single-sensor BWIM system. It employs a deep neural network that extracts complex features through multiple neural layers and can detect vehicle properties from a single strain sensor. We name this approach deep sensing. The estimated properties include lane, speed, trajectory, and number of axles. The system adjusts parameters for vehicle detection automatically by consulting a traffic surveillance camera, thereby eliminating any heuristic parameter adjustment. The reliability of the system will be verified automatically, considering the huge numbers of real vehicles crossing a bridge. Once the system is optimized, the camera may be removed. In future work, the detector could be combined with the main strain sensor for weighing. This paper paves the way toward monosensor BWIM systems.
更多查看译文
AI 理解论文
溯源树
样例
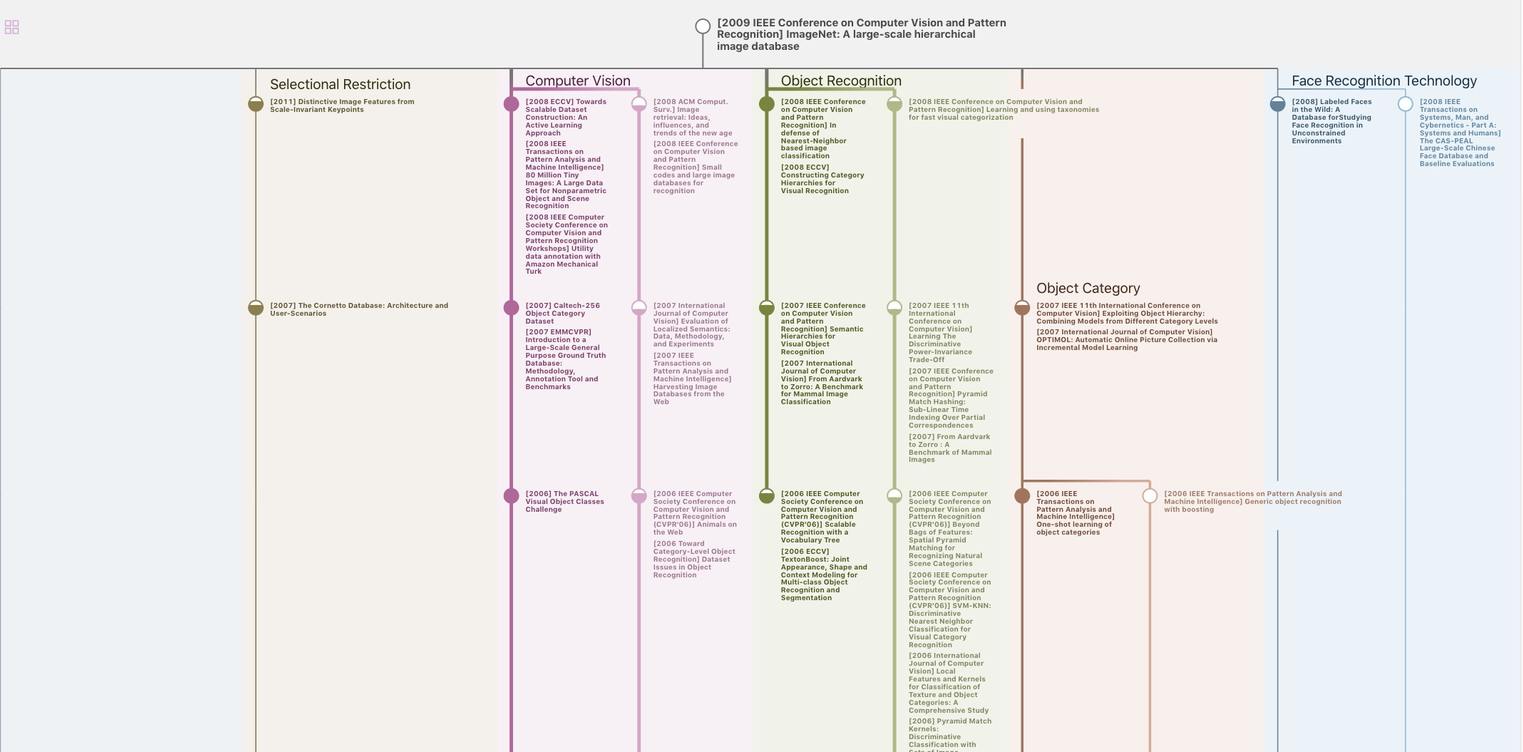
生成溯源树,研究论文发展脉络
Chat Paper
正在生成论文摘要