Kernel-based data transformation model for nonlinear classification of symbolic data
SOFT COMPUTING(2022)
摘要
Symbolic data are usually composed of some categorical variables used to represent discrete entities in many real-world applications. Mining of symbolic data is more difficult than numerical data due to the lack of inherent geometric properties of this type of data. In this paper, we use two kinds of kernel learning methods to create a kernel estimation model and a nonlinear classification algorithm for symbolic data. By using the kernel smoothing method, we construct a squared-error consistent probability estimator for symbolic data, followed by a new data transformation model proposed to embed symbolic data into Euclidean space. Based on the model, the inner product and distance measure between symbolic data objects are reformulated, allowing a new Support Vector Machine (SVM), called SVM-S, to be defined for nonlinear classification on symbolic data using the Mercer kernel learning method. The experiment results show that SVM can be much more effective for symbolic data classification based on our proposed model and measures.
更多查看译文
关键词
Symbolic data,Kernel learning method,Data transformation model,Nonlinear classification
AI 理解论文
溯源树
样例
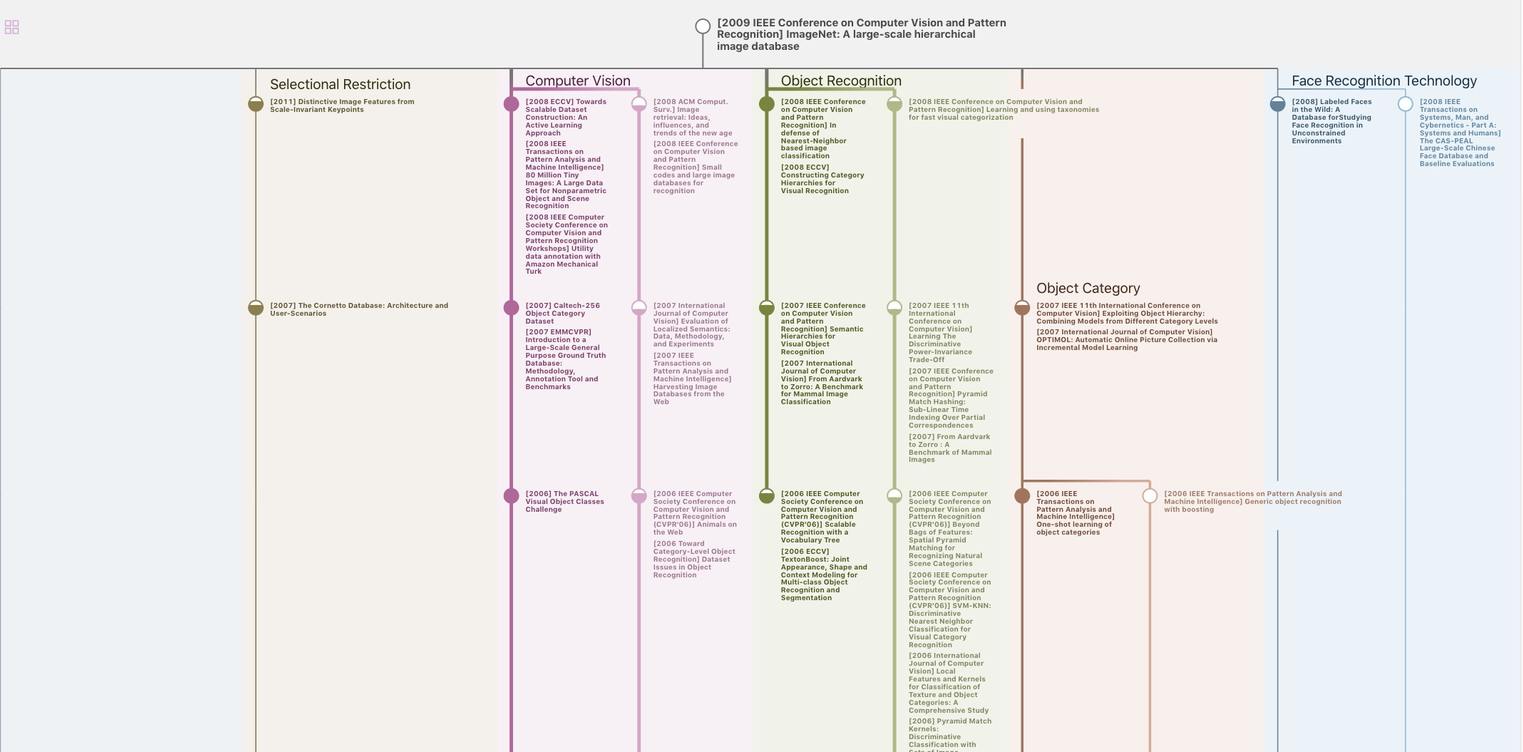
生成溯源树,研究论文发展脉络
Chat Paper
正在生成论文摘要