A Dataset for Tackling Gender Bias in Text
semanticscholar(2018)
摘要
Gender bias is found in personal conversations, in the media, in historical writings, popular culture, in the labor force, household responsibilities and now in machines (Fiebert and Meyer, 1997; Kingdon, 2005). Gender bias occurs in machine learning models when they are trained with data that contains human-like biases (Haussler, 1988). Current research is focused on detecting and correcting for gender bias in existing machine learning models, such as word embeddings (Zhao et al., 2018: Bolukbasi et al., 2016), coreference resolution (Zhao et al., 2018) and visual recognition tasks involving language (captioning) (Zhao et al., 2017). Rather than removing gender bias in current machine learning models, we are tackling the issue at its root and creating a gender bias dataset with which to train a machine learning model. Enabling a model to learn gender bias would allow for gender bias detection and possibly correction in text.
更多查看译文
AI 理解论文
溯源树
样例
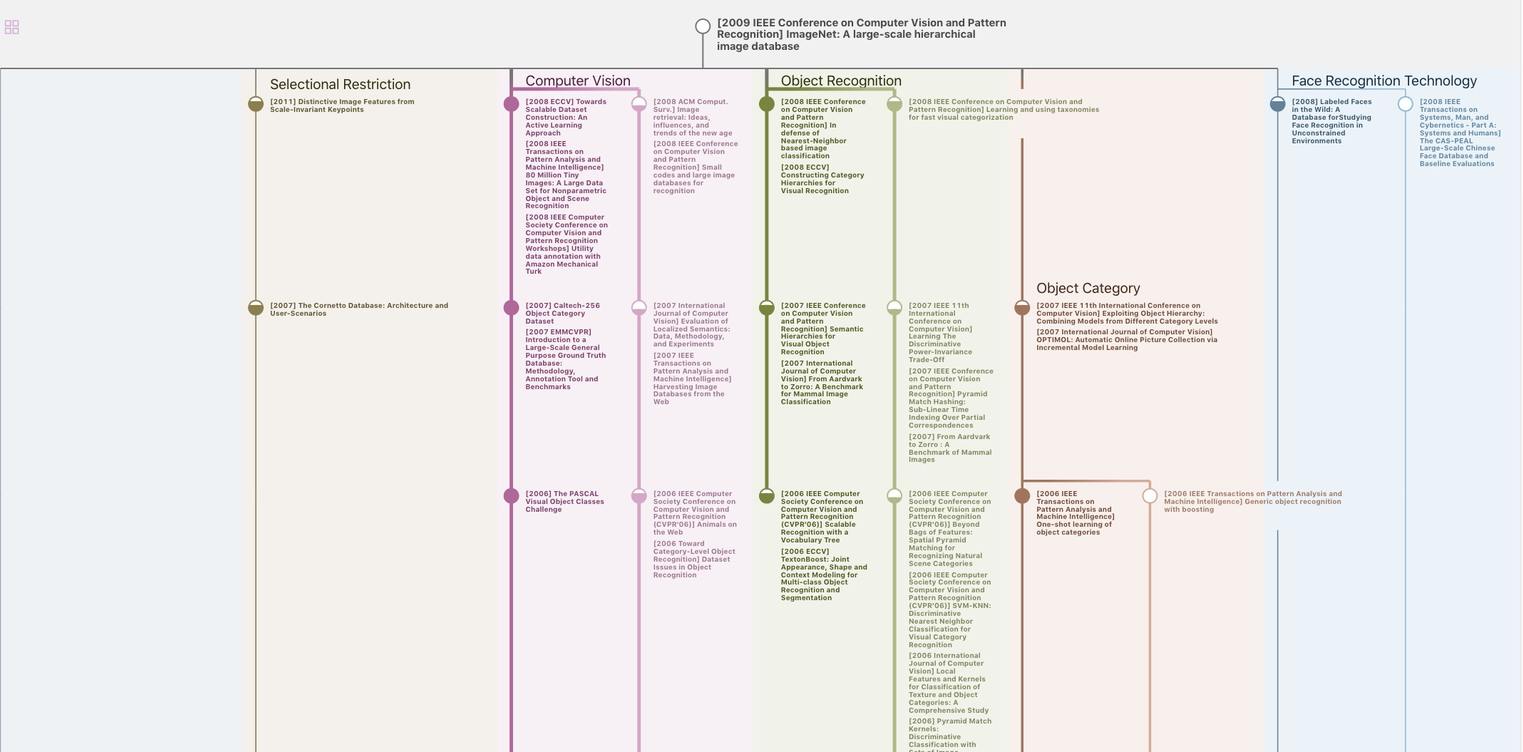
生成溯源树,研究论文发展脉络
Chat Paper
正在生成论文摘要