Random Persistence Diagram Generation
ArXiv(2021)
摘要
Topological data analysis (TDA) studies the shape patterns of data. Persistent homology (PH) is a widely used method in TDA that summarizes homological features of data at multiple scales and stores them in persistence diagrams (PDs). In this paper, we propose a random persistence diagram generation (RPDG) method that generates a sequence of random PDs from the ones produced by the data. RPDG is underpinned by (i) a model based on pairwise interacting point processes for inference of persistence diagrams, and (ii) by a reversible jump Markov chain Monte Carlo (RJ-MCMC) algorithm for generating samples of PDs. A first example, which is based on a synthetic dataset, demonstrates the efficacy of RPDG and provides a detailed comparison with other existing methods for sampling PDs. A second example demonstrates the utility of RPDG to solve a materials science problem given a real dataset of small sample size.
更多查看译文
关键词
generation
AI 理解论文
溯源树
样例
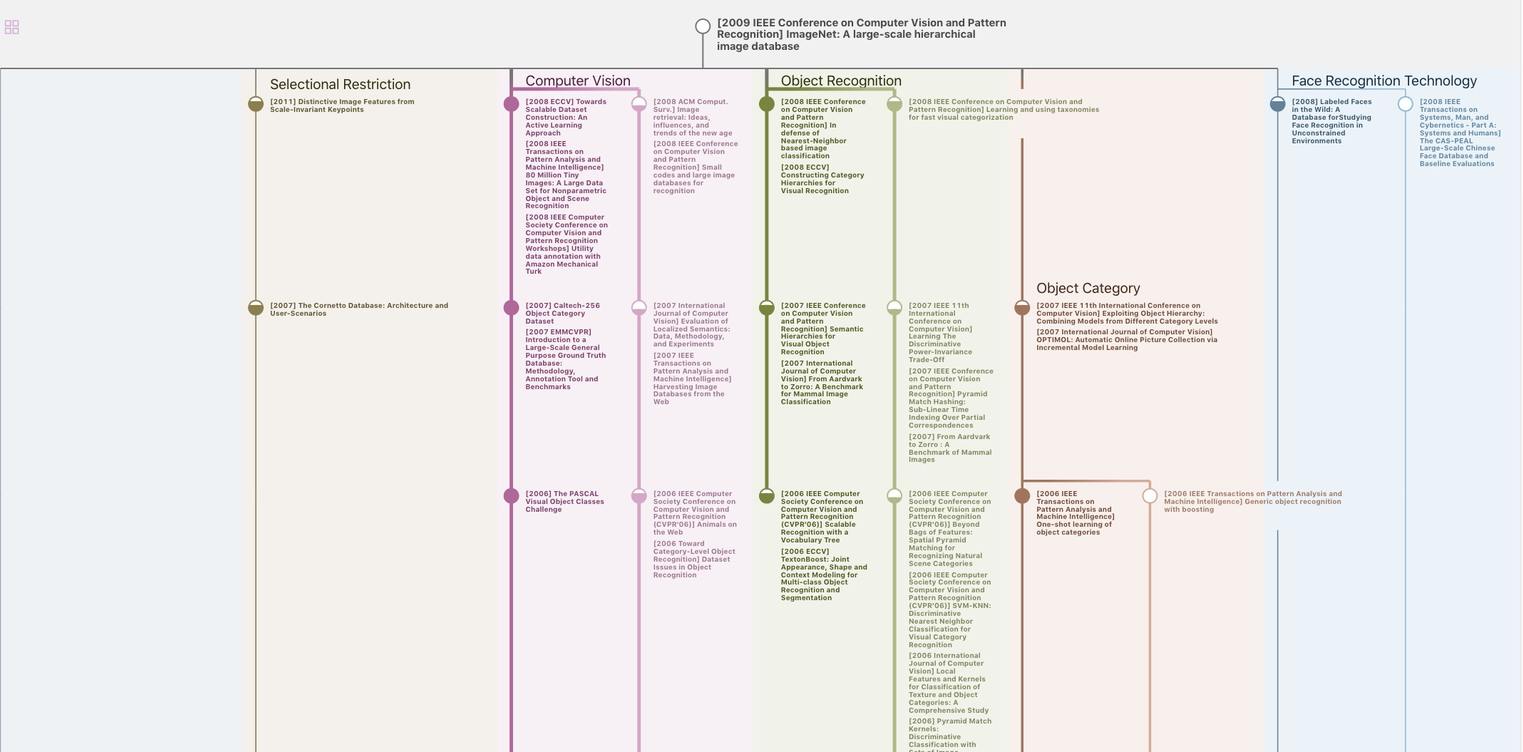
生成溯源树,研究论文发展脉络
Chat Paper
正在生成论文摘要