Detecting deception through facial expressions in a dataset of videotaped interviews: A comparison between human judges and machine learning models
COMPUTERS IN HUMAN BEHAVIOR(2022)
摘要
In the last decades, research has claimed facial micro-expressions are a reliable means to detect deception. However, experimental results showed that trained and nave human judges observing facial micro-expressions can distinguish liars from truth-tellers with an accuracy just slightly above the chance level. More promising results recently came from the field of artificial intelligence, in which machine learning (ML) techniques are used to identify micro-expressions and are trained to distinguish deceptive statements from genuine ones. In this paper, we test the ability of different feature extraction methods (i.e., improved dense trajectories, OpenFace) and ML techniques (i.e., support vector machines vs. deep neural networks) to distinguish liars from truth-tellers based on facial micro-expressions, using a new video data set collected in low-stakes situations. During the interviews, a technique to increase liars' cognitive load was applied, facilitating cues of lies to emerge. Results highlighted that support vector machines (SVMs) coupled with OpenFace resulted in the best performing method (AUC = 0.72 videos without cognitive load; AUC = 0.78 videos with cognitive load). All the tested classifiers performed better when a cognitive load was imposed on the interviewee, confirming that the technique of increasing cognitive load during an interview facilitates deception recognition. In the same task, human judges obtained an accuracy of 57%. Results are discussed and compared with the previous literature, confirming that artificial intelligence performs better than humans in lie-detection tasks do, even when humans have more information to make a decision.
更多查看译文
关键词
Facial micro-expressions,Deception detection,Machine learning,Deep learning,Low-stakes lies
AI 理解论文
溯源树
样例
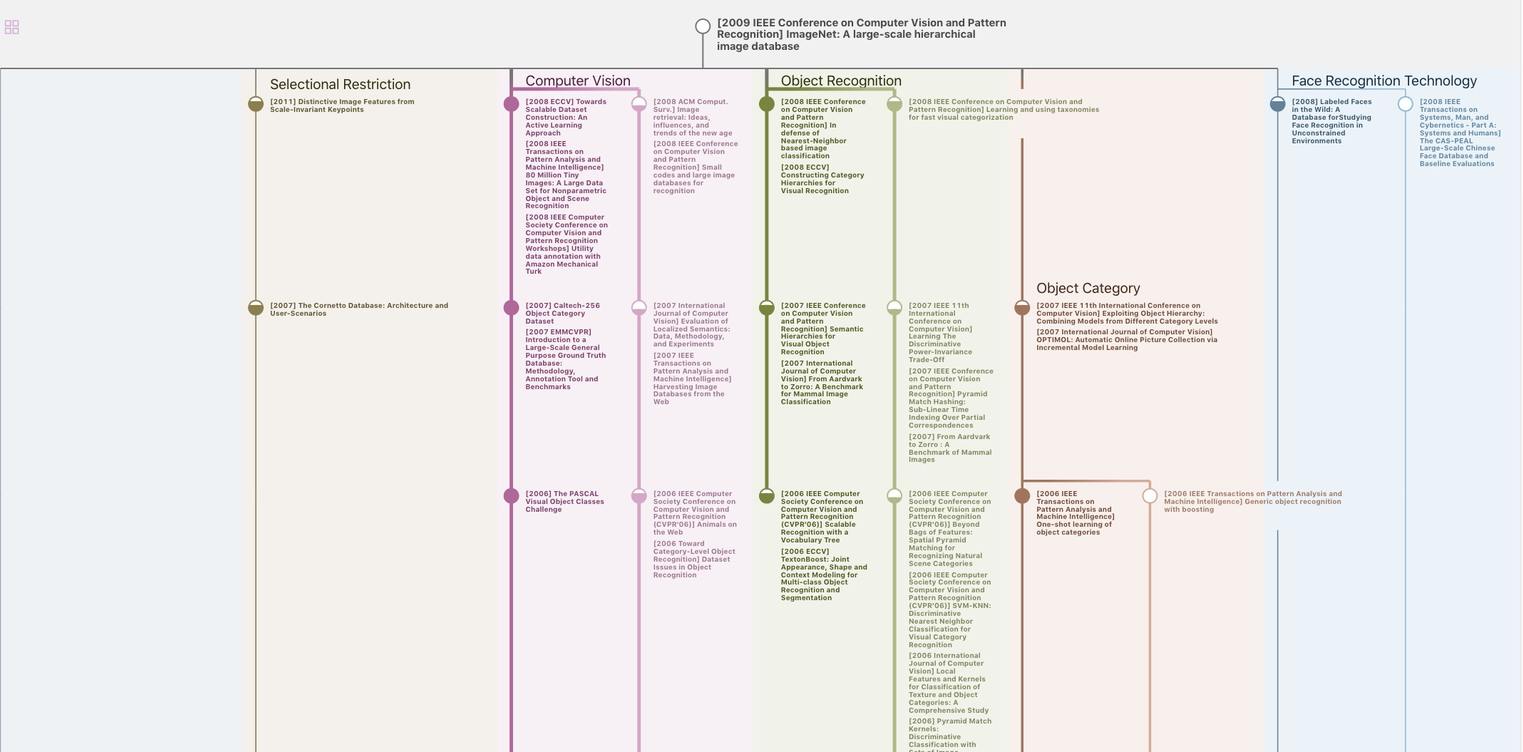
生成溯源树,研究论文发展脉络
Chat Paper
正在生成论文摘要