Adversarial Robustness of Deep Sensor Fusion Models
WACV(2022)
摘要
We experimentally study the robustness of deep camera-LiDAR fusion architectures for 2D object detection in autonomous driving. First, we find that the fusion model is usually both more accurate, and more robust against single-source attacks than single-sensor deep neural networks. Furthermore, we show that without adversarial training, early fusion is more robust than late fusion, whereas the two perform similarly after adversarial training. However we note that single-channel adversarial training of deep fusion is often detrimental even to robustness. Moreover we observe cross-channel externalities, where single-channel adversarial training reduces robustness to attacks on the other channel. Additionally, we observe that the choice of adversarial model in adversarial training is critical: using attacks restricted to cars' bounding boxes is more effective in adversarial training and exhibits less significant cross-channel externalities. Finally, we find that joint-channel adversarial training helps mitigate many of the issues above, but does not significantly boost adversarial robustness.
更多查看译文
关键词
Object Detection/Recognition/Categorization Datasets, Evaluation and Comparison of Vision Algorithms, Deep Learning -> Adversarial Learning, Adversarial Attack and Defense Methods
AI 理解论文
溯源树
样例
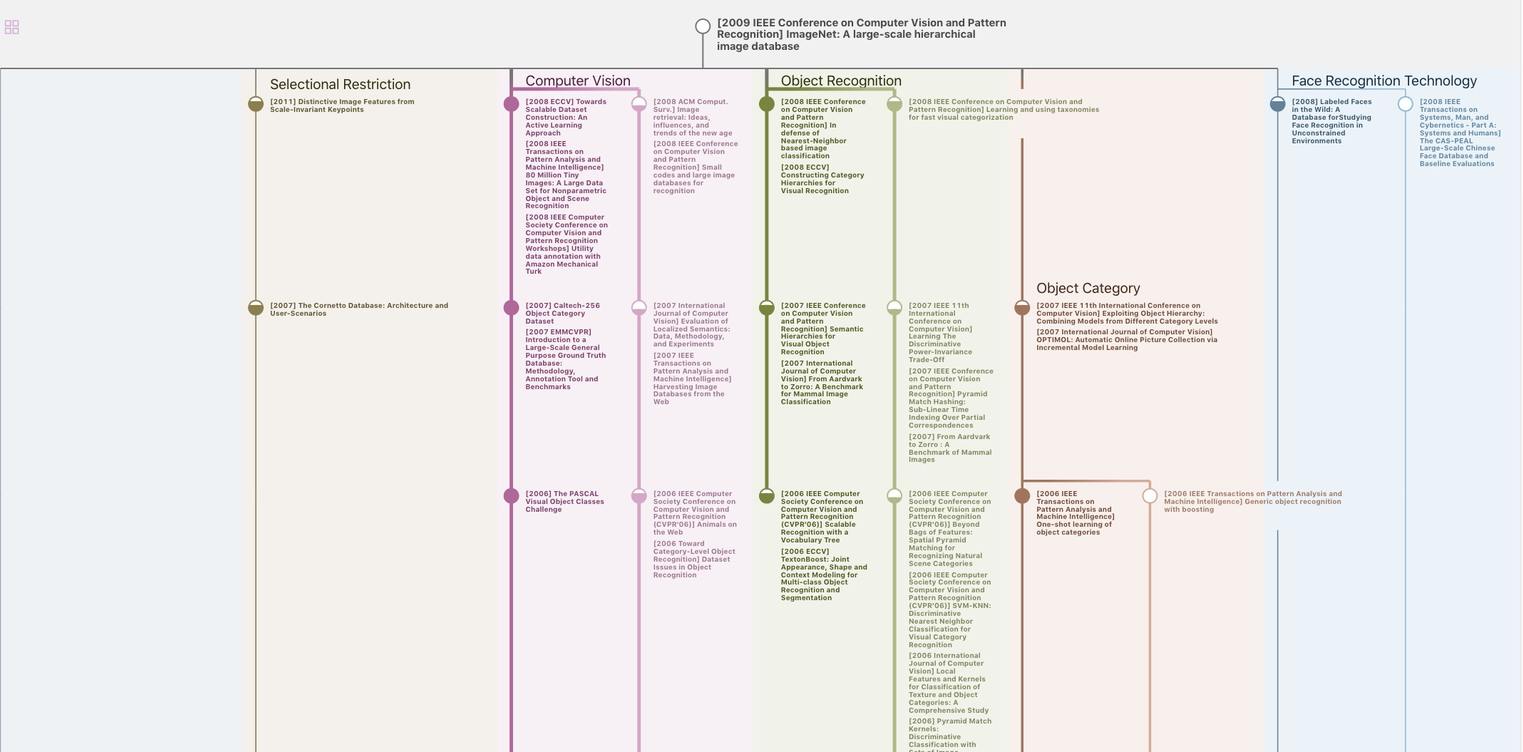
生成溯源树,研究论文发展脉络
Chat Paper
正在生成论文摘要