Real-time Full Body Capture with Inter-part Correlations – Supplemental Document –
semanticscholar(2021)
摘要
In Fig. 1, we present more qualitative results on in-thewild videos. To process the image sequence, we first use the off-the-shell human detector [8] to obtain the body bounding box of the first frame. After that, for each frame, its body bounding box is updated according to the 2D keypoint estimation of the previous frame. In this way, our method tracks the subject and performs 3D capture fully automatically. As a frame-based approach, our method inevitably suffers from the temporal jittering, which is also shared by the previous work of Choutas et al. [2]. We adopt a basic temporal filter [1] for smooth visualization. Further, we compare our results with the state-of-the-art approaches of Choutas et al. [2] and Xiang et al. [10] in Fig. 2, where we present results of equal visual quality but much faster inference speed. We present failure cases in Fig. 3. In the first row, our method cannot handle the handhand interaction very well. This is because distinguishing the two hands from monocular color input is a very challenging task, and such samples are rare in our training data. In the second row, our approach does not estimate the face color and the hand pose very well due to the unseen appearance: the face is occluded by the goggles, while the hands are under the gloves. Finally, to illustrate the discrepancy in keypoint definitions of different datasets, we present the result of our model on the same image under different sets of dataset-specific extended keypoints in Fig. 4. The positions for the hips, shoulders, and neck are quite different, while the elbows, ankles, knees are always consistent across datasets. Please refer to our supplementary video for more results.
更多查看译文
AI 理解论文
溯源树
样例
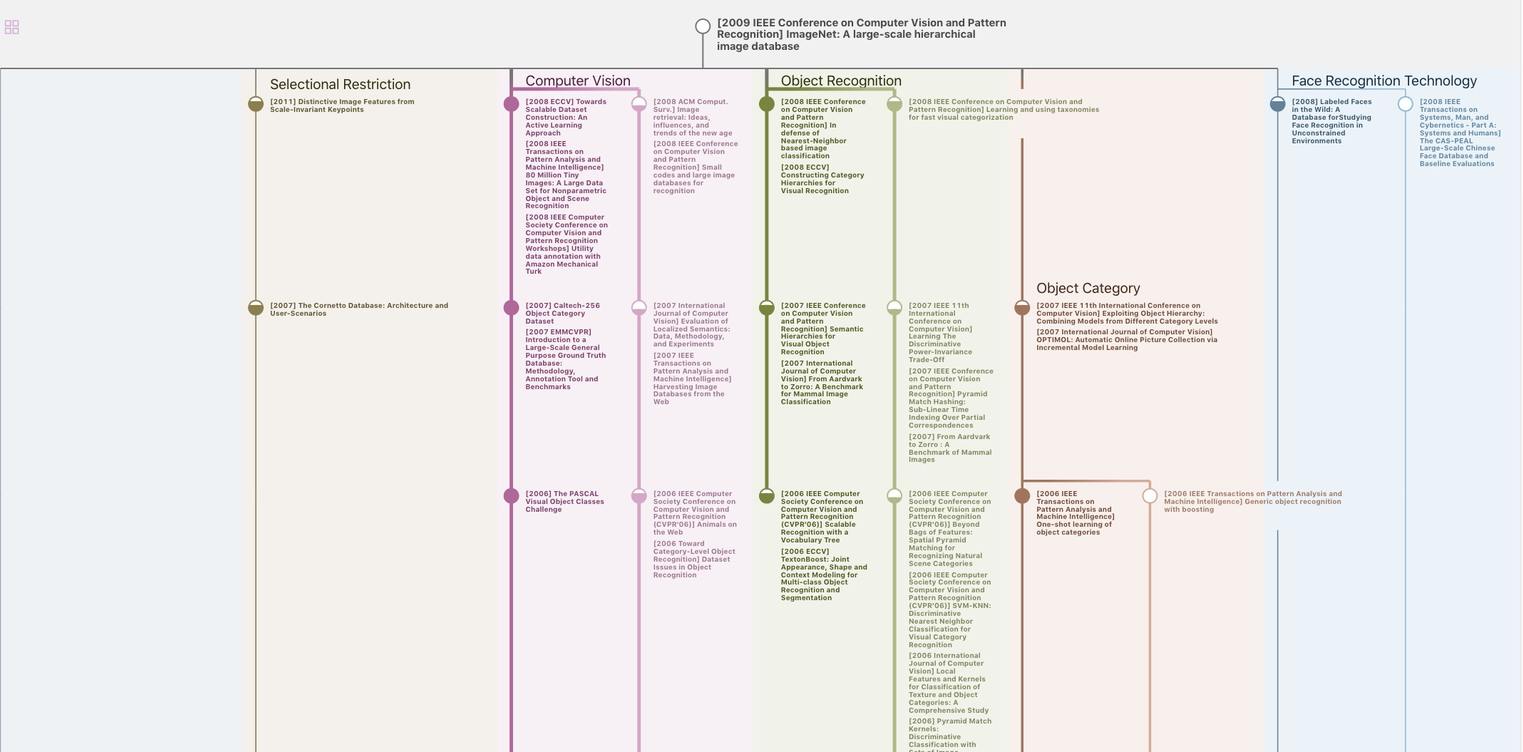
生成溯源树,研究论文发展脉络
Chat Paper
正在生成论文摘要