Towards Autonomous Machine Learning in Chemistry via Evolutionary Algorithms
semanticscholar(2019)
摘要
Machine learning has been emerging as a promising tool in the chemical and materials domain. In this paper, we introduce a framework to automatically perform rational model selection and hyperparameter optimization that are important concerns for the efficient and successful use of machine learning, but have so far largely remained unexplored by this community. The framework features four variations of genetic algorithm and is implemented in the chemml program package. Its performance is benchmarked against popularly used algorithms and packages in the data science community and the results show that our implementation outperforms these methods both in terms of time and accuracy. The effectiveness of our implementation is further demonstrated via a scenario involving multi-objective optimization for model selection.
更多查看译文
AI 理解论文
溯源树
样例
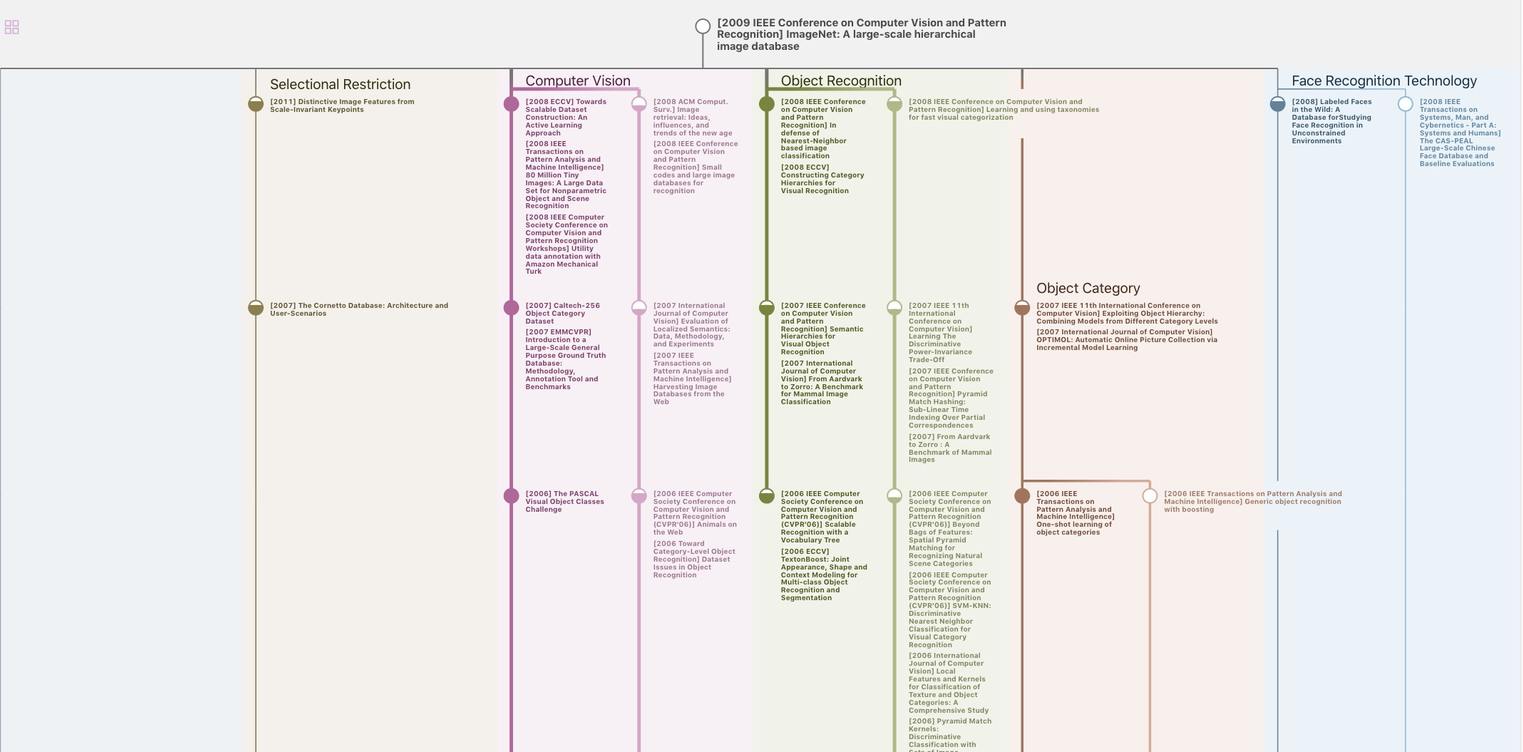
生成溯源树,研究论文发展脉络
Chat Paper
正在生成论文摘要