Cutting plane generation through sparse principal component analysis
SIAM JOURNAL ON OPTIMIZATION(2022)
摘要
Quadratically constrained quadratic programs (QCQPs) are optimization models whose remarkable expressiveness have made them a cornerstone of methodological research for non convex optimization problems. However, modern methods to solve a general QCQP fail to scale, encountering computational challenges even with just a few hundred variables. Specifically, a semi definite programming (SDP) relaxation is typically employed, which provides strong dual bounds for QCQPs but relies on memory-intensive algorithms. An appealing alternative is to replace the SDP with an easier-to-solve linear programming relaxation while still achieving strong bounds. In this work, we make advances toward achieving this goal by developing a computationally efficient linear cutting plane algorithm that emulates the SDP-based approximations of nonconvex QCQPs. The cutting planes are required to be sparse, in order to ensure a numerically attractive approximation, and efficiently computable. We present a novel connection between such sparse cut generation and the sparse principal component analysis problem in statistics, which allows us to achieve these two goals. We show extensive computational results advocating for the use of our approach.
更多查看译文
关键词
quadratically constrained quadratic programs,nonconvex optimization,sparse cutting planes,sparse principal component analysis
AI 理解论文
溯源树
样例
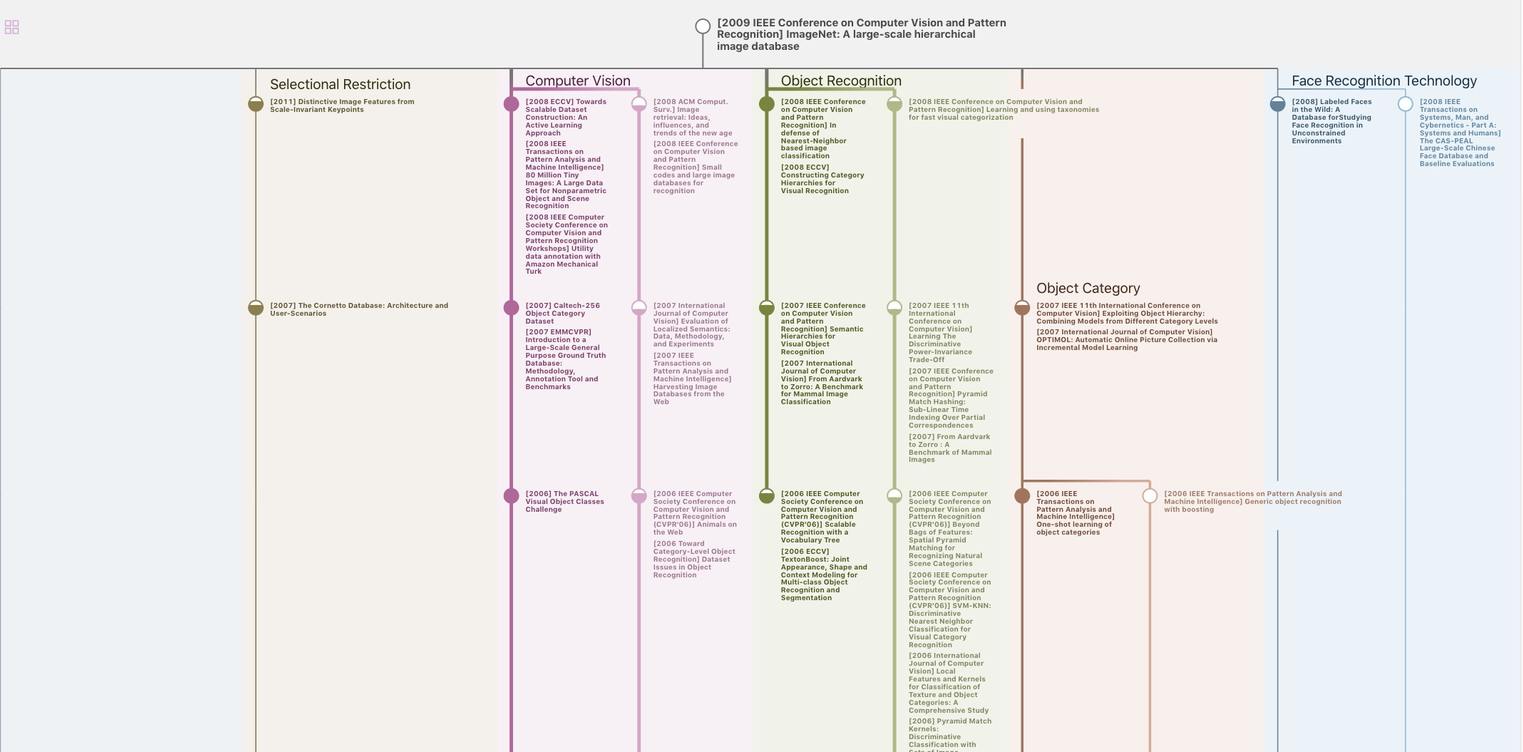
生成溯源树,研究论文发展脉络
Chat Paper
正在生成论文摘要