Relevance attack on detectors
PATTERN RECOGNITION(2022)
摘要
This paper focuses on high-transferable adversarial attacks on detectors, which are hard to attack in a black-box manner, because of their multiple-output characteristics and the diversity across architectures. To pursue a high attack transferability, one plausible way is to find a common property across detectors, which facilitates the discovery of common weaknesses. We are the first to suggest that the relevance map from interpreters for detectors is such a property. Based on it, we design a Relevance Attack on Detectors (RAD), which achieves a state-of-the-art transferability, exceeding existing results by above 20%. On MS COCO, the detection mAPs for all 8 black-box architectures are more than halved and the segmentation mAPs are also significantly influenced. Given the great transferability of RAD, we generate the first adversarial dataset for object detection and instance segmentation, i.e., Adversarial Objects in COntext (AOCO), which helps to quickly evaluate and improve the robustness of detectors. (c) 2021 Elsevier Ltd. All rights reserved.
更多查看译文
关键词
Adversarial attack,Attack transferability,Black-box attack,Relevance map,Interpreters,Object detection
AI 理解论文
溯源树
样例
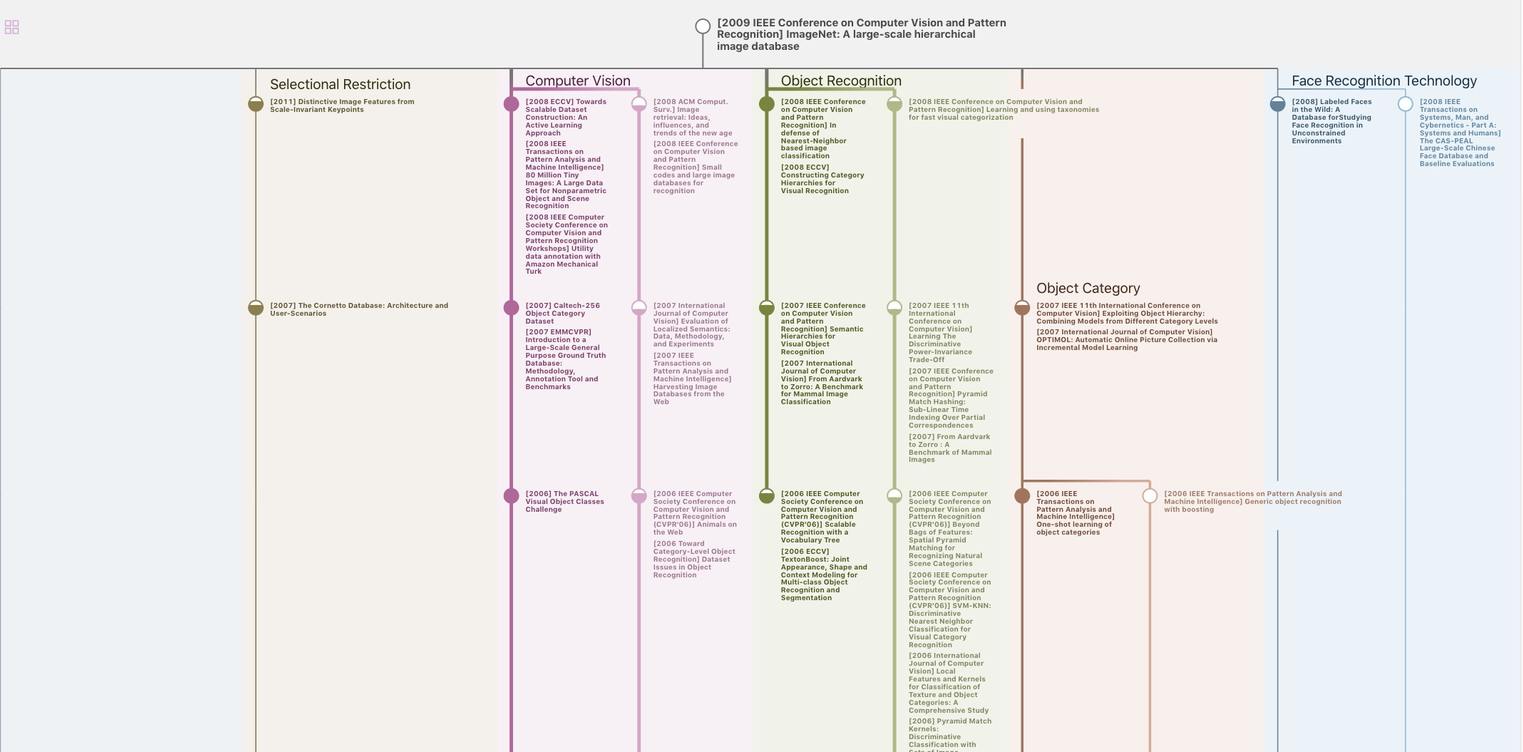
生成溯源树,研究论文发展脉络
Chat Paper
正在生成论文摘要