Preprocessing approaches in machine learning-based groundwater potential mapping: an application to the Koulikoro and Bamako regions, Mali
semanticscholar(2021)
摘要
Groundwater is crucial for domestic supplies in the Sahel, where the strategic importance of aquifers can only be expected to increase in the coming years due to climate change. Groundwater potential mapping is gaining recognition as a 10 valuable tool to underpin water management practices in the region, and hence, to improve water access. This paper presents a machine learning method to map groundwater potential and illustrates it through an application to two regions of Mali. A set of explanatory variables for the presence of groundwater is developed first. Several scaling methods (standardization, normalization, maximum absolute value and min-max scaling) are used to avoid the pitfalls associated with the reclassification of explanatory variables. A number of supervised learning classifiers is then trained and tested on a large borehole database 15 (n=3,345) in order to find meaningful correlations between the presence or absence of groundwater and the explanatory variables. This process identifies noisy, collinear and counterproductive variables and excludes them from the input dataset. Tree-based algorithms, including the AdaBoost, Gradient Boosting, Random Forest, Decision Tree and Extra Trees classifiers were found to outperform other algorithms on a consistent basis (accuracy >0.85), whereas maximum absolute value and standardization proved the most efficient methods to scale explanatory variables. Borehole flow rate data is used to calibrate 20 the results beyond standard machine learning metrics, thus adding robustness to the predictions. The southern part of the study area was identified as the better groundwater prospect, which is consistent with the geological and climatic setting. From a methodological standpoint, the outcomes lead to three major conclusions: (1) because there is a priori no way to know which algorithm will work better on a given dataset, we advocate the use of a large number of machine learning classifiers, out of which the best are subsequently picked for ensembling; (2) standard machine learning metrics may be of limited value when 25 appraising map outcomes, and should be complemented with hydrogeological indicators whenever possible; and (3) the scaling of the variables helps to minimize bias arising from expert judgement and maintains robust predictive capabilities.
更多查看译文
AI 理解论文
溯源树
样例
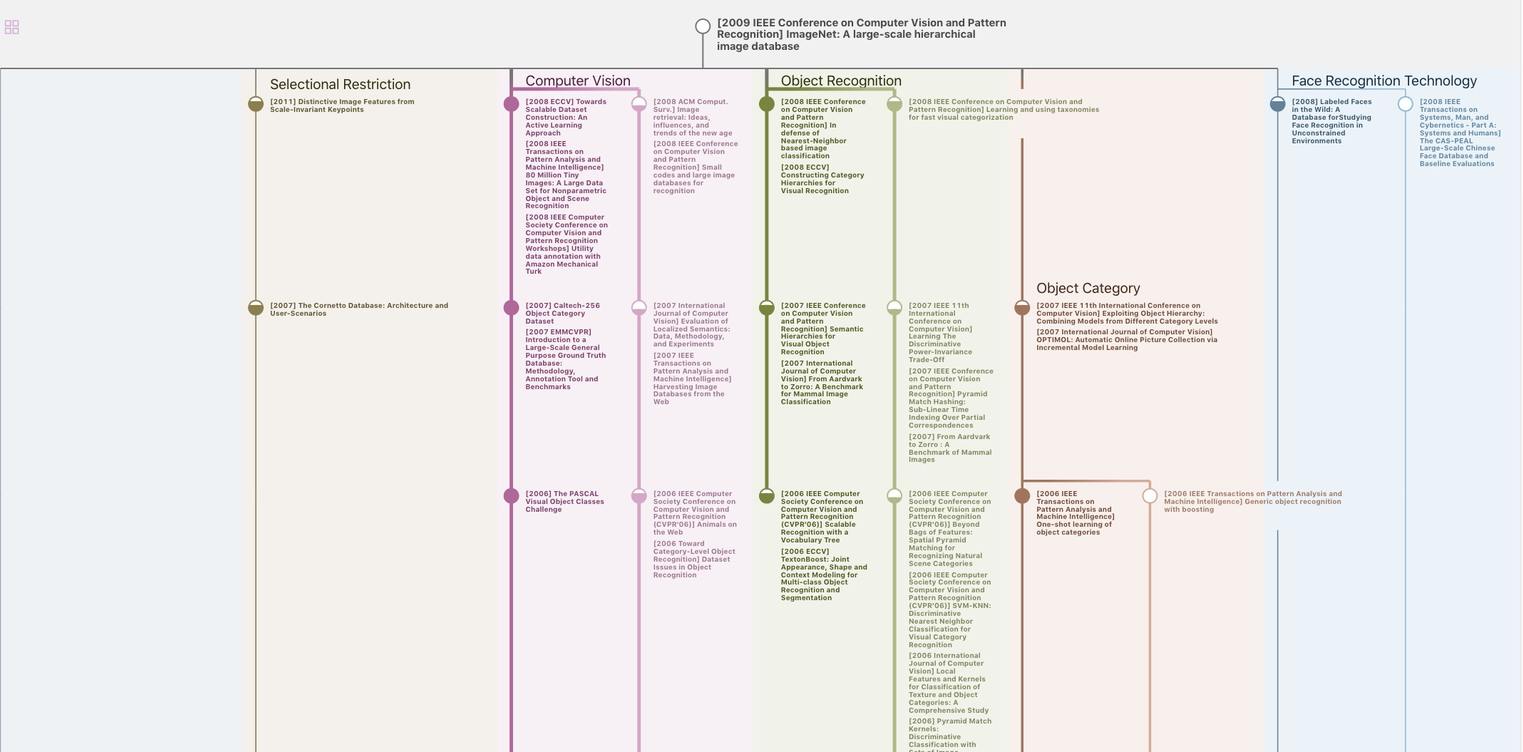
生成溯源树,研究论文发展脉络
Chat Paper
正在生成论文摘要