Deep Network Approximation With Accuracy Independent of Number of Neurons∗
semanticscholar(2021)
摘要
This paper develops simple feed-forward neural networks that achieve the universal approximation property for all continuous functions with a fixed finite number of neurons. These neural networks are simple because they are designed with a simple and computable continuous activation function σ leveraging a triangularwave function and a softsign function. We prove that σ-activated networks with width 36d(2d + 1) and depth 11 can approximate any continuous function on a d-dimensioanl hypercube within an arbitrarily small error. Hence, for supervised learning and its related regression problems, the hypothesis space generated by these networks with a size not smaller than 36d(2d+1)×11 is dense in the space of continuous functions. Furthermore, classification functions arising from image and signal classification are in the hypothesis space generated by σ-activated networks with width 36d(2d + 1) and depth 12, when there exist pairwise disjoint closed bounded subsets of R such that the samples of the same class are located in the same subset.
更多查看译文
AI 理解论文
溯源树
样例
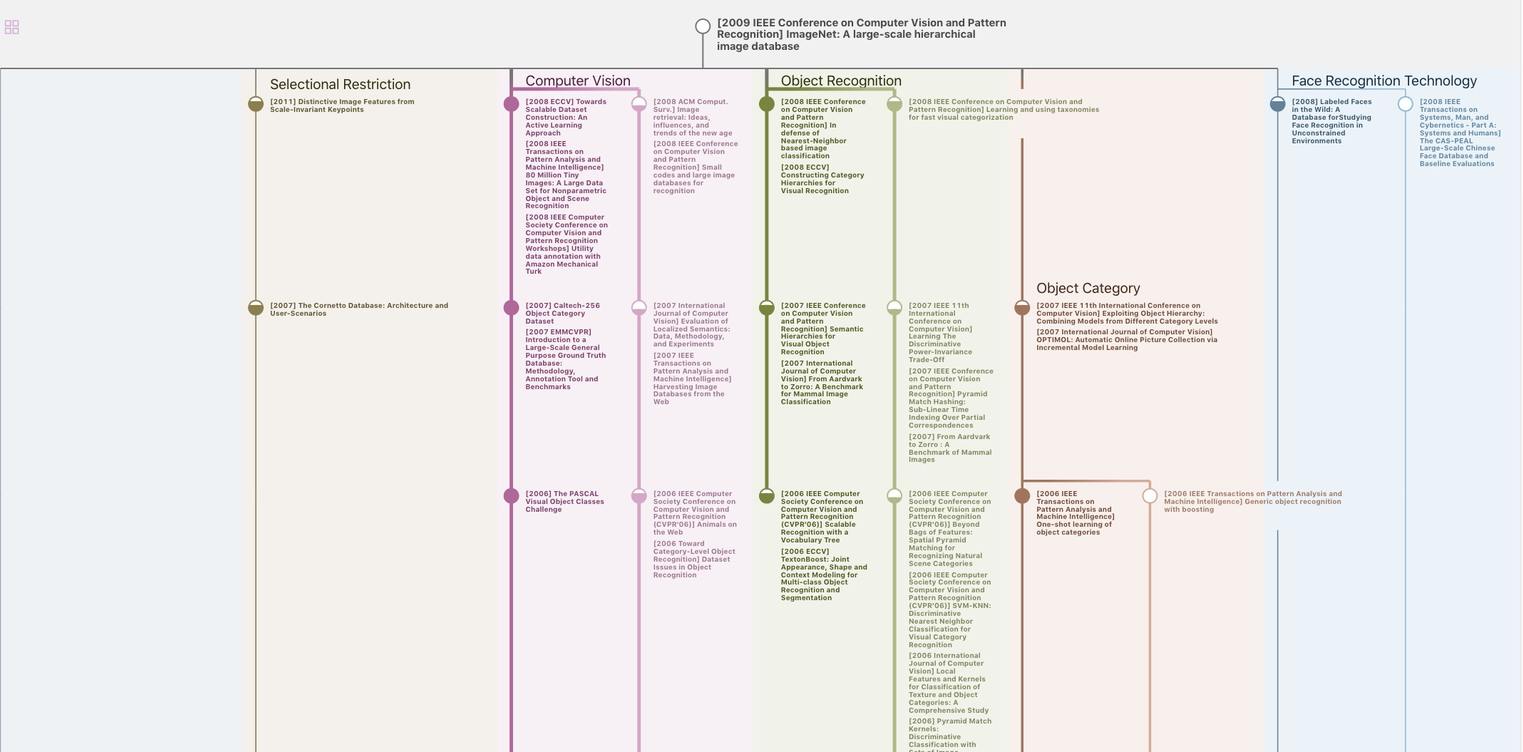
生成溯源树,研究论文发展脉络
Chat Paper
正在生成论文摘要