Learning to generate shape from global-local spectra
ArXiv(2021)
摘要
In this work, we present a new learning-based pipeline for the generation of 3D shapes. We build our method on top of recent advances on the so called shape-from-spectrum paradigm, which aims at recovering the full 3D geometric structure of an object only from the eigenvalues of its Laplacian operator. In designing our learning strategy, we consider the spectrum as a natural and ready to use representation to encode variability of the shapes. Therefore, we propose a simple decoder-only architecture that directly maps spectra to 3D embeddings; in particular, we combine information from global and local spectra, the latter being obtained from localized variants of the manifold Laplacian. This combination captures the relations between the full shape and its local parts, leading to more accurate generation of geometric details and an improved semantic control in shape synthesis and novel editing applications. Our results confirm the improvement of the proposed approach in comparison to existing and alternative methods.
更多查看译文
关键词
spectra,shape,learning,global-local
AI 理解论文
溯源树
样例
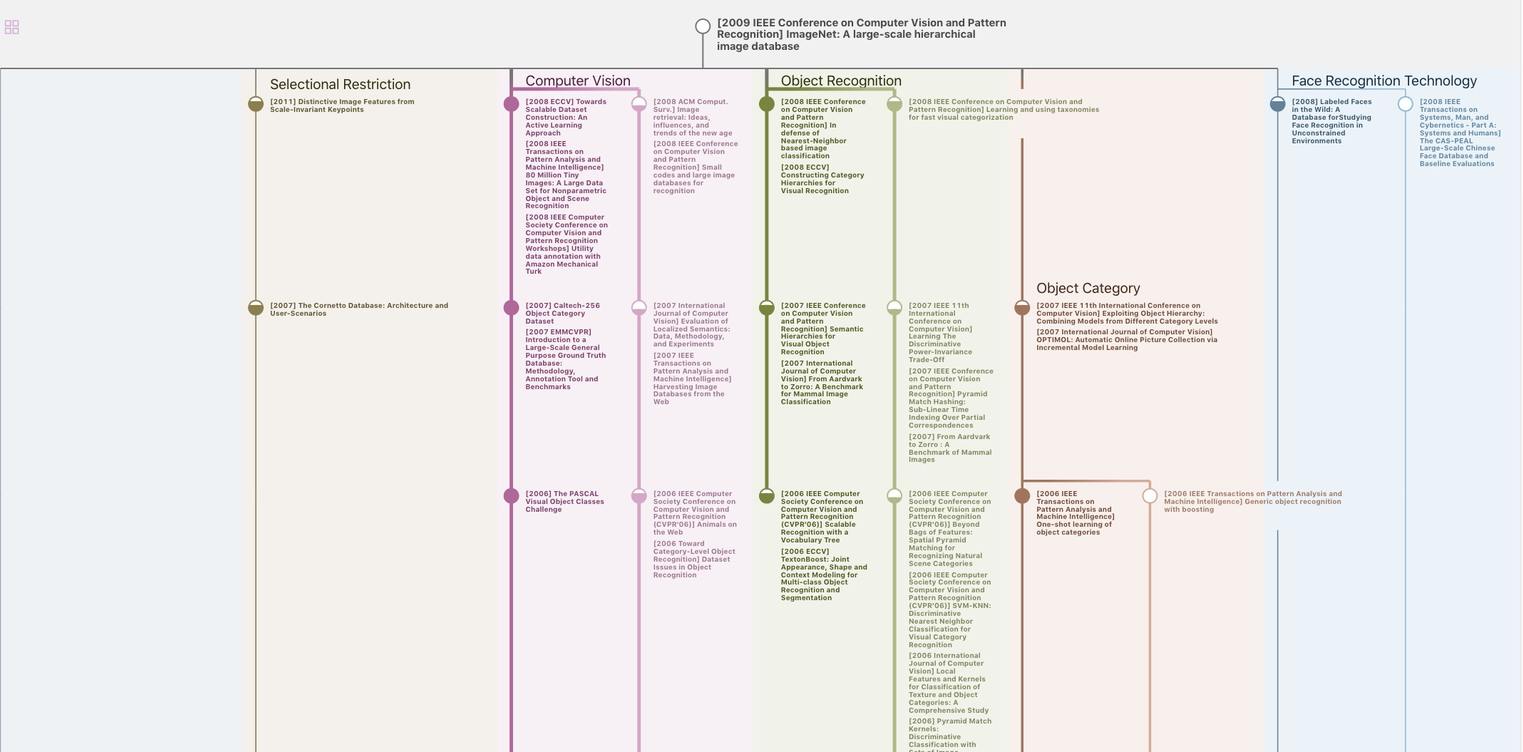
生成溯源树,研究论文发展脉络
Chat Paper
正在生成论文摘要