Three-Dimensional Permeability Inversion Using Convolutional Neural Networks and Positron Emission Tomography
WATER RESOURCES RESEARCH(2022)
摘要
Quantification of heterogeneous multiscale permeability in geologic porous media is key for understanding and predicting flow and transport processes in the subsurface. Recent utilization of in situ imaging, specifically positron emission tomography (PET), enables the measurement of three-dimensional (3-D) time-lapse radiotracer solute transport in geologic media. However, accurate and computationally efficient characterization of the permeability distribution that controls the solute transport process remains challenging. Leveraging the relationship between local permeability variation and solute advection behavior, an encoder-decoder based convolutional neural network (CNN) is implemented as a permeability inversion scheme using a single PET scan of a radiotracer pulse injection experiment as input. The CNN can accurately capture the 3-D spatial correlation between the permeability and the radiotracer solute arrival time difference maps in geologic cores. We first test the inversion accuracy using synthetic test datasets and then test the accuracy on a suite of experimental PET imaging datasets acquired on four different geologic cores. The network-predicted permeability maps from the geologic cores are used to parameterize forward numerical models that are directly compared with the experimental PET imaging data. The results indicate that a single trained network can generate robust 3-D permeability inversion maps in seconds. Numerical models parameterized with these permeability maps closely capture the experimentally observed solute arrival time behavior. This work provides an unprecedented approach for efficiently characterizing multiscale permeability heterogeneity in complex geologic samples.
更多查看译文
关键词
convolutional neural network, deep learning, machine learning, permeability inversion, positron emission tomography, X-ray computed tomography
AI 理解论文
溯源树
样例
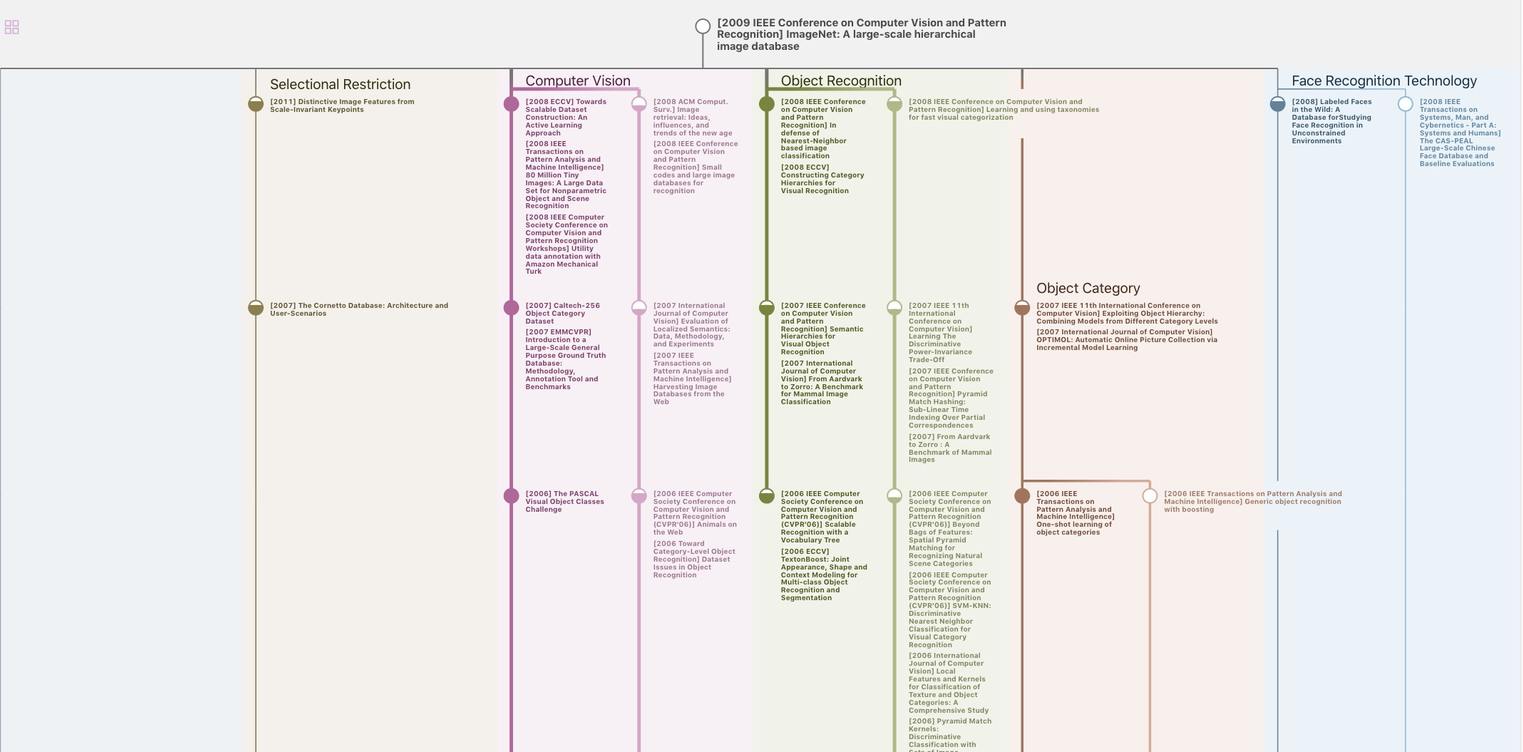
生成溯源树,研究论文发展脉络
Chat Paper
正在生成论文摘要