Response time correction of slow response sensor data by deconvolution of the growth-law equation
GEOSCIENTIFIC INSTRUMENTATION METHODS AND DATA SYSTEMS(2021)
摘要
Abstract. Accurate, high resolution measurements are essential to improve our understanding of environmental processes. Several chemical sensors relying on membrane separation extraction techniques have slow response times due to a dependence on equilibrium partitioning across the membrane separating the measured medium (i.e., a measuring chamber) and the medium of interest (i.e., a solvent). We present a new technique for deconvolving slow sensor response signals using statistical inverse theory; applying a weighted linear least squares estimator with the growth-law as measurement model. The solution is regularized using model sparsity, assuming changes in the measured quantity occurs with a certain time-step, which can be selected based on domain-specific knowledge or L-curve analysis. The advantage of this method is that it: 1) models error propagation, providing an explicit uncertainty estimate of the response time corrected signal, 2) enables evaluation of the solutions self consistency, and 3) only requires instrument accuracy, response time, and data as input parameters. Functionality of the technique is demonstrated using simulated, laboratory, and field measurements. In the field experiment, the coefficient of determination (R2) of a slow response methane sensor in comparison with an alternative, fast response sensor, significantly improved from 0.18 to 0.91 after signal deconvolution. This shows how the proposed method can open up a considerably wider set of applications for sensors and methods suffering from slow response times due to a reliance on the efficacy of diffusion processes.
更多查看译文
关键词
deconvolution,sensor,slow-response,growth-law
AI 理解论文
溯源树
样例
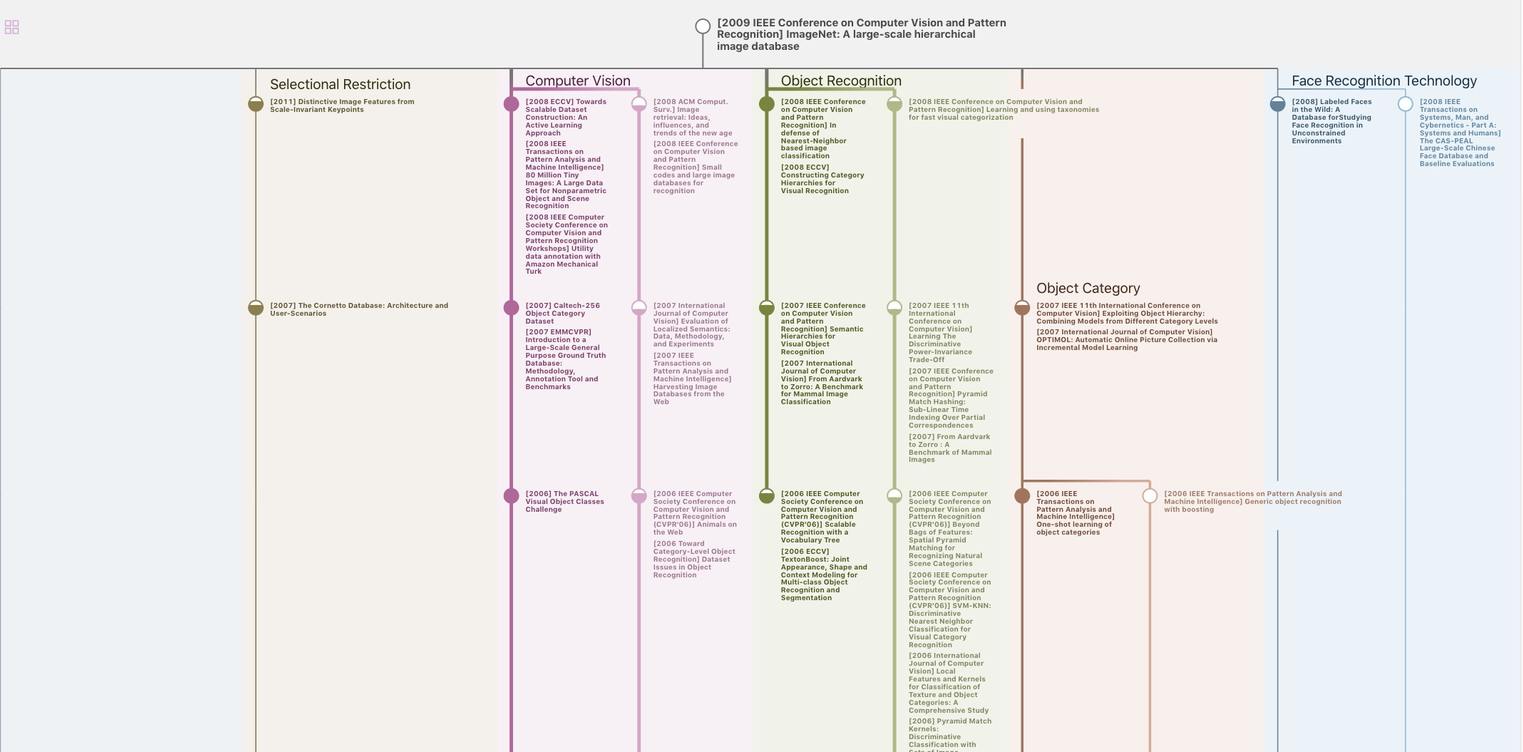
生成溯源树,研究论文发展脉络
Chat Paper
正在生成论文摘要