Predic ng the tensile proper es of addi vely manufactured Ti-6Al-4V via electron beam deposi on
semanticscholar(2020)
摘要
Addi vely manufactured materials are gaining wide a�en on owing to the manufacturing benefits as it results in near net shape components. It is well known that the manufacturing processes affects the performance of the components via microstructural features and the mechanical proper es. There is an urgent need to understand the processing-structure-property-performance rela onship for the materials manufactures via such innova ve techniques. Strategies are needed to quan fy and modify the mechanical proper es. This study assists to design and tailor the process parameters based on the final proper es required. Current work predicts the yield strength of addi vely manufactured Ti-6Al-4V with different post heat treatments. A thermal model predicted by ABAQUS is fed into an implementa on of Langmuir equa on that predicts the chemistry which is then used in a phenomenological equa on predic ng the yield strength. The model is confirmed via experiments showing less than 2% devia on from the predicated proper es. A sta s cal model gives design allowables that have an uncertainty of less than 1 ksi. 1. Introduc on In the main, it is desirable to have an integrated computa onal materials engineering (ICME) strategy to predict the proper es of addi vely manufactured materials, and thereby accelerate the applica on of these advanced manufacturing approaches by various commercial companies. In any ICME strategy, it is necessary to fully understand and capture the relevant details of the materials science paradigm: composi on, processing, microstructure, proper es, and performance. This work represents a mul -ins tu on, 6-year effort to develop a robust ICME strategy capable of predic ng the proper es and performance of addi vely manufactured Ti-6Al-4V to within 7MPa (1ksi). Key components of the framework include: (i) a thermal model; (ii) a Langmuir-based model to predict the asdeposited composi on; (iii) a phase field model to predict the microstructure; (iv) an ar ficial neural network-gene c algorithm extracted phenomenological equa on to predict strength; (v) a sta s cally based model for the probabilis c predic on of strength; and (vi) an ABAQUS model and framework that integrates the knowledge of the program into a predic ve tool. 2. Materials and Experiments MATEC Web of Conferences 321, 11083 (2020) https://doi.org/10.1051/matecconf/202032111083 The 14 World Conference on Titanium © The Authors, published by EDP Sciences. This is an open access article distributed under the terms of the Creative Commons Attribution License 4.0 (http://creativecommons.org/licenses/by/4.0/). To develop an ICME framework, a large-scale method of addi ve manufacturing (i.e., the Sciaky electron beam addi vely manufactured (EBAM) process) was selected to produce Ti-6Al-4V. This par cular method uses a high energy electron beam as a heat source, which operates under vacuum, and feeds in a wire that is melted and added to the previously deposited material. The as-deposited material was sec oned and subjected to various thermal histories [1]. The sta c mechanical proper es were determined, and a database was developed that consisted of measurements of the as-deposited composi on, microstructure, and mechanical proper es. A variety of state-of-the-art materials characteriza on techniques were adopted, ranging from the more rou ne backsca�ered electron microscope images that were subsequently quan fied to advanced precession electron diffrac on techniques that were applied to spa ally resolve and quan fy disloca on densi es by rela ng the presence of such defects to la ce curvature at a nm length scale [2]. Following materials characteriza on, a physically based equa on was developed using a hybrid ar ficial neural network gene c algorithm approach that has been described elsewhere. Briefly, this approach permits a gene c algorithm approach to match an n-variable equa on that has been determined using the flexible ar ficial neural network to a n-variable phenomenological equa on that has been postulated based upon known physics. The resul ng equa on overcomes several of the limita ons of ar ficial neural networks, including devia ons in the equa on in regions of data sparsity. This equa on, originally developed for wrought Ti-6Al-4V, was modified slightly for the addi vely manufactured material. Specifically, a texture debit term was introduced for the observed decrease in yield strength along the primary x-y-z cartesian coordinate axis, and a Taylor hardening terms incorporated for varia ons in disloca on density. A sophis cated sta s cal approach was incorporated to manage the small variability that exists between the predicted values and the experimentally measured values when both simulated and experimentally measured data is plo�ed on a cumula ve probability distribu on func on plot (c-pdf). A sta s cal adjustment of the model data and experimental data is sufficient to apply to future data and have such future data lie almost perfectly on the c-pdf. In parallel, an ABAQUS code was developed to predict: the thermal history; the chemistry varia on due to vola liza on of certain species under vacuum; the microstructure; and the resul ng sta c mechanical proper es. The predicted c-pdfs were compared to the measured c-pdfs and found to be in very good agreement. 3. Results and Discussion The results of this work are extensive, and too broad to appear in this proceedings paper. For complete descrip ons, the reader is pointed elsewhere [3-6]. The most important details of this work are given below.
更多查看译文
AI 理解论文
溯源树
样例
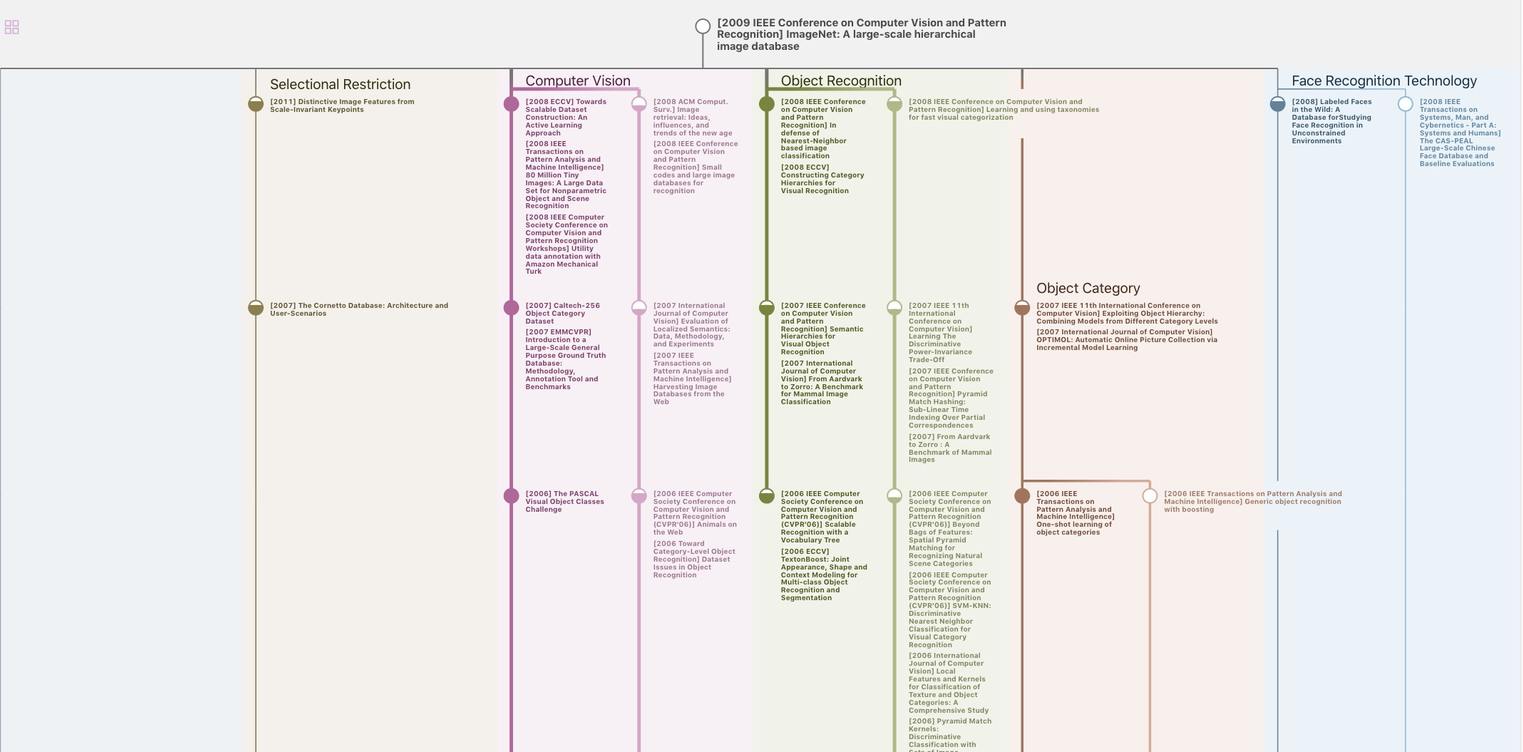
生成溯源树,研究论文发展脉络
Chat Paper
正在生成论文摘要