A GAN framework for Instance Segmentation using the Mutex Watershed Algorithm
semanticscholar(2018)
摘要
Modern deep learning approaches for image segmentation without semantics often utilize pixelwise loss functions for boundary prediction, especially when dense groundtruth is available. However, these approaches may not be sensitive to structural similarity (e.g. tiny holes), that can severely affect postprocessing. This work explores the possibility to augment the training with a GAN loss function and in conjunction with the Mutex Watershed graph clustering algorithm.We show that this method and additional auxiliary task losses improve the quality of the Adjusted Rand Score over the score reported in [1]. Additionally, we present an ablation study to show that when the images in the target domain are constrained to be discrete, adding an auxiliary task with smooth target images significantly improves the training performance/stability. We also use the discriminator to train on unlabeled images which further improves our results.
更多查看译文
AI 理解论文
溯源树
样例
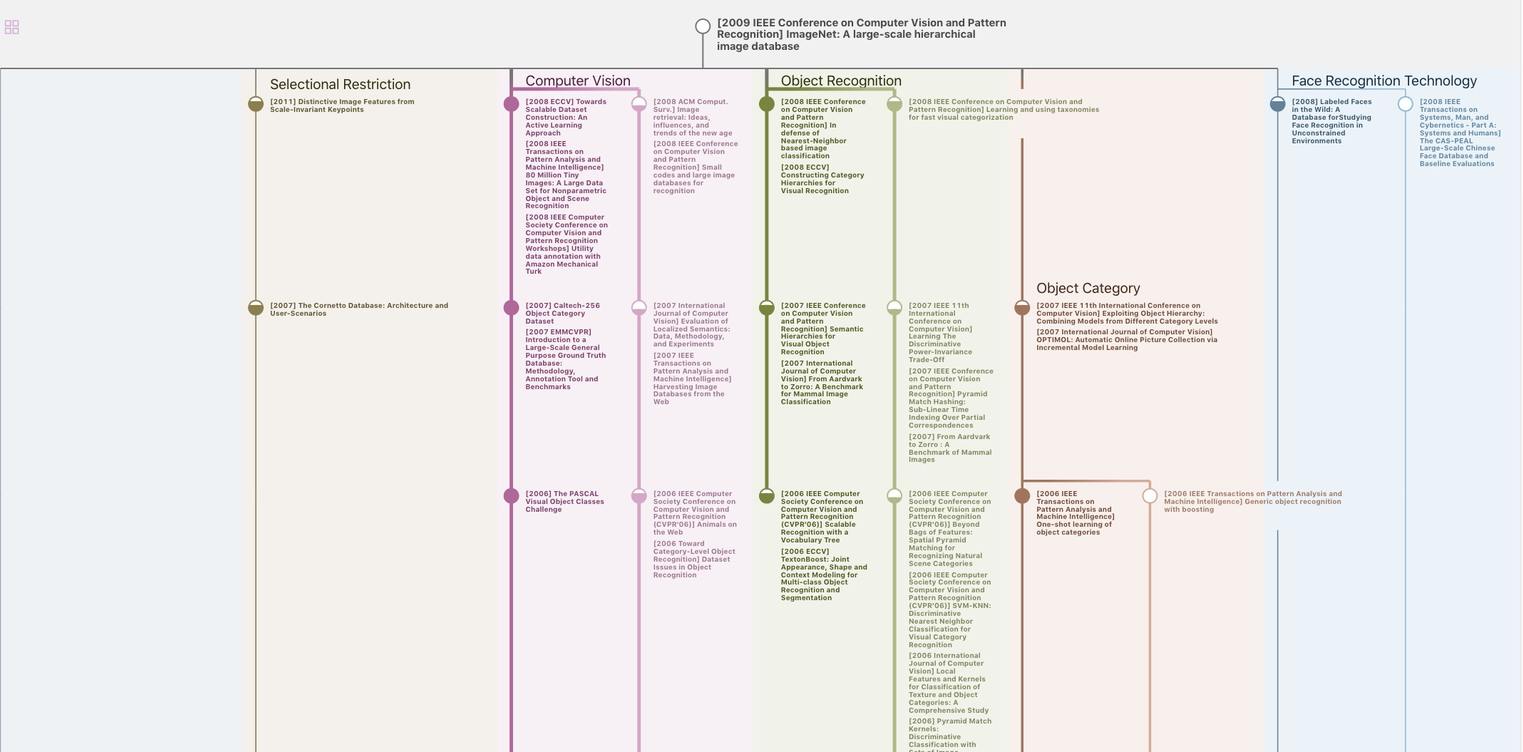
生成溯源树,研究论文发展脉络
Chat Paper
正在生成论文摘要