An explainable artificial intelligence approach for predicting cardiovascular outcomes using electronic health records.
medRxiv(2022)
摘要
Understanding the conditionally-dependent clinical variables that drive cardiovascular health outcomes is a major challenge for precision medicine. Here, we deploy a recently developed massively scalable comorbidity discovery method called Poisson Binomial based Comorbidity discovery (PBC), to analyze Electronic Health Records (EHRs) from the University of Utah and Primary Children's Hospital (over 1.6 million patients and 77 million visits) for comorbid diagnoses, procedures, and medications. Using explainable Artificial Intelligence (AI) methodologies, we then tease apart the intertwined, conditionally-dependent impacts of comorbid conditions and demography upon cardiovascular health, focusing on the key areas of heart transplant, sinoatrial node dysfunction and various forms of congenital heart disease. The resulting multimorbidity networks make possible wide-ranging explorations of the comorbid and demographic landscapes surrounding these cardiovascular outcomes, and can be distributed as web-based tools for further community-based outcomes research. The ability to transform enormous collections of EHRs into compact, portable tools devoid of Protected Health Information solves many of the legal, technological, and data-scientific challenges associated with large-scale EHR analyses.
更多查看译文
关键词
predicting cardiovascular outcomes,explainable artificial intelligence approach,artificial intelligence,health
AI 理解论文
溯源树
样例
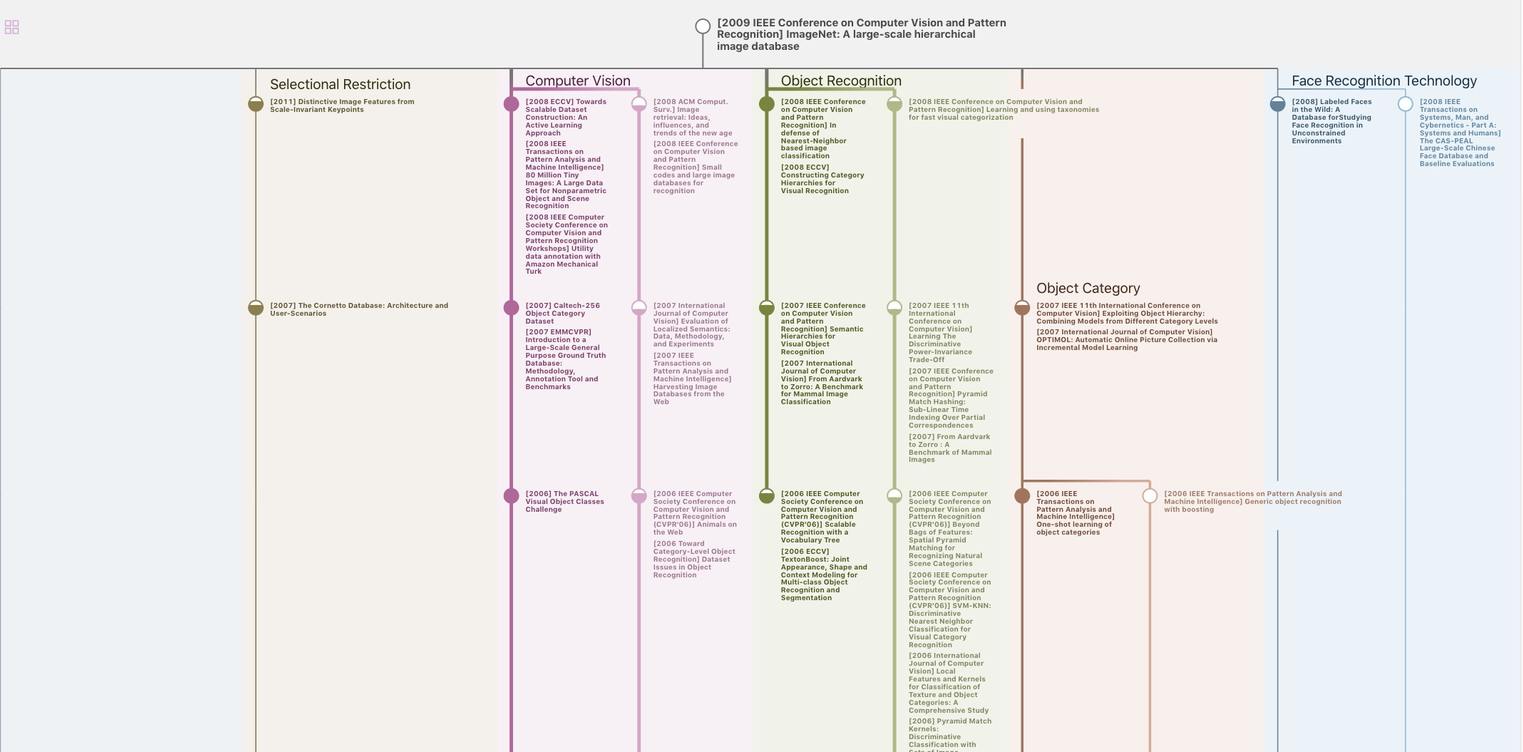
生成溯源树,研究论文发展脉络
Chat Paper
正在生成论文摘要